Near On-Policy Experience Sampling in Multi-Objective Reinforcement Learning.
International Joint Conference on Autonomous Agents and Multi-agent Systems(2022)
摘要
In multi-objective decision problems, the same state-action pair under different preference weights between the objectives, constitutes different optimal policies. The introduction of changing preference weights interferes with the convergence of the network, and can even stop the network from converging. In this paper, we propose a novel experience sampling strategy for multi-objective RL problems, which samples transitions based on the weight and state similarities, to get the sampled experiences close to on-policy. We apply our sampling strategy in multi-objective deep RL algorithms on known benchmark problems, and show that this strongly improves performance.
更多查看译文
AI 理解论文
溯源树
样例
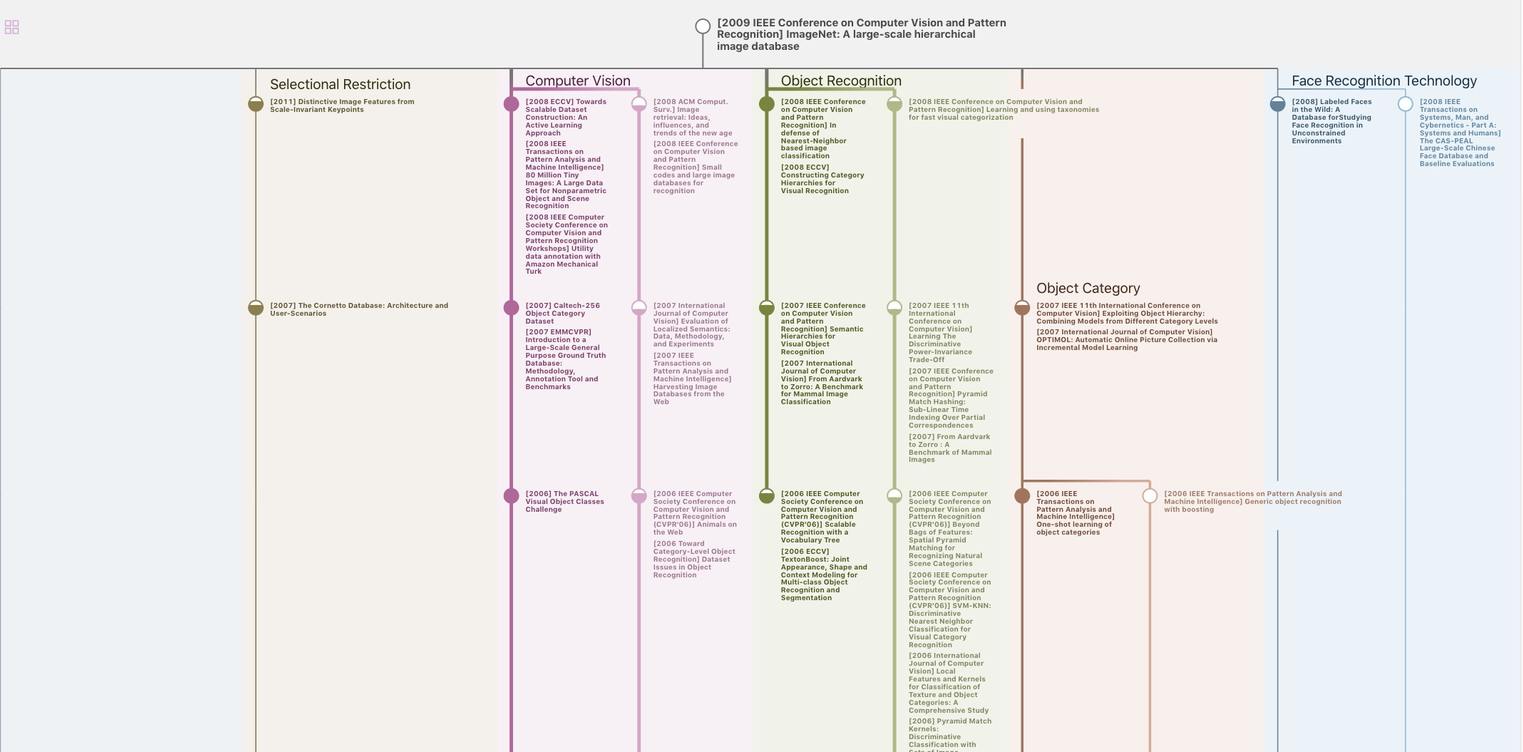
生成溯源树,研究论文发展脉络
Chat Paper
正在生成论文摘要