Reinforcement Learning for Traffic Signal Control Optimization: A Concept for Real-World Implementation.
International Joint Conference on Autonomous Agents and Multi-agent Systems(2022)
摘要
The improvement of traffic control is one of the most important but also most ambitious goals in the field of urban traffic today. Due to the domain's long history, new methods must first establish themselves in practice and, above all, demonstrate reasonable and robust optimization results. Potential methods which came up in recent years are Reinforcement Learning (RL) and Multi-Agent Reinforcement Learning (MARL). In research, the use of RL/MARL for traffic optimization is widely spread. However, it has not yet managed to make it from simulations into practical implementation due to: (1) The lack of real data for the online estimation of the state space. (2) The compatibility to real controllers. (3) The necessity of guarantees to ensure the resilience of the controls. Enabled by a project developing testbeds and practical approaches to optimize traffic through AI, we present a concept to close this gap to the online control of real networks and to overcome the stated issues.
更多查看译文
AI 理解论文
溯源树
样例
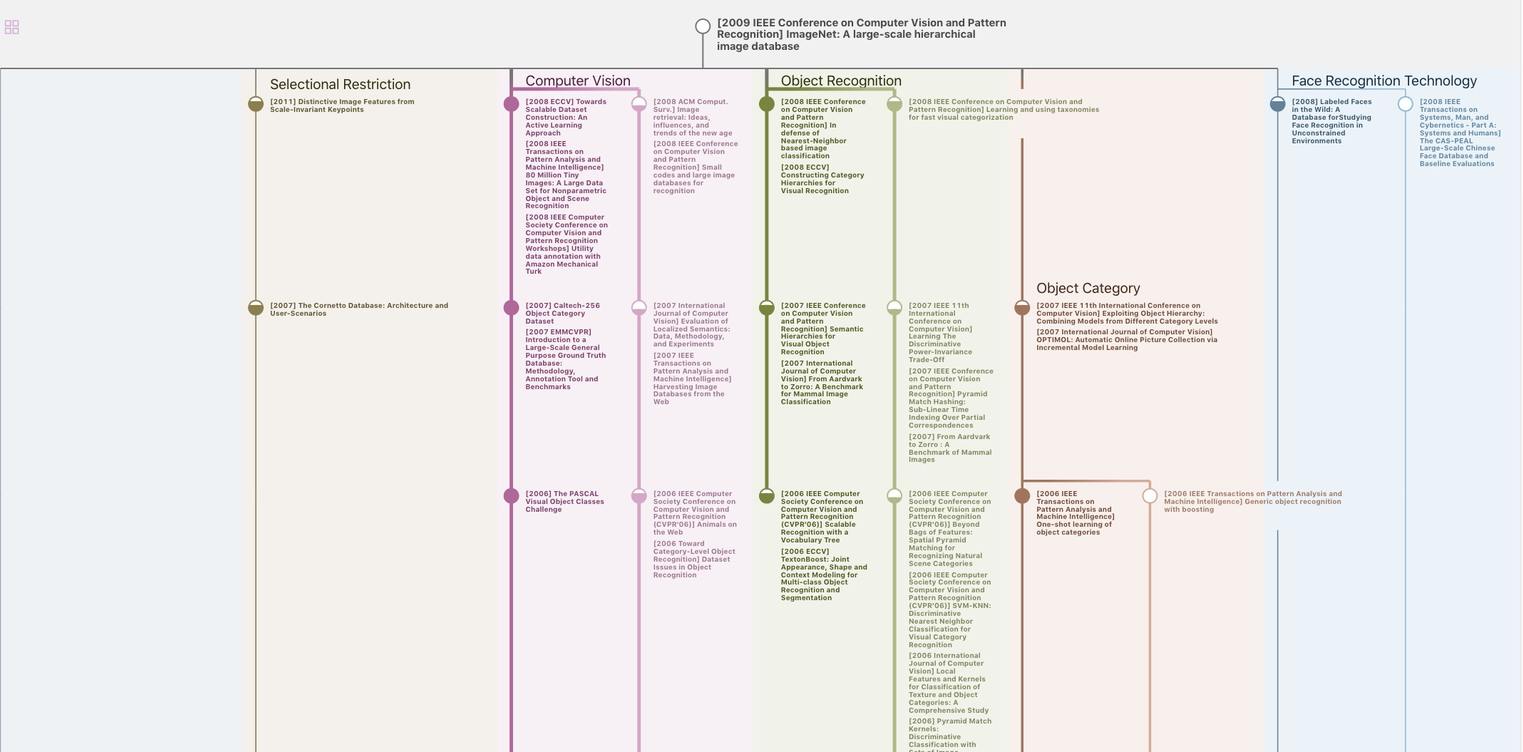
生成溯源树,研究论文发展脉络
Chat Paper
正在生成论文摘要