Optimal Local Bayesian Differential Privacy over Markov Chains.
International Joint Conference on Autonomous Agents and Multi-agent Systems(2022)
摘要
In the literature of data privacy, differential privacy is the most popular model. An algorithm is differentially private if its outputs with and without any individual's data are indistinguishable. In this paper, we focus on data generated from a Markov chain and argue that Bayesian differential privacy (BDP) offers more meaningful guarantees in this context. Our main theoretical contribution is providing a mechanism for achieving BDP when data is drawn from a binary Markov chain. We improve on the state-of-the-art BDP mechanism and show that our mechanism provides the optimal noise-privacy tradeoffs for any local mechanism up to negligible factors. We also briefly discuss a non-local mechanism which adds correlated noise. Lastly, we perform experiments on synthetic data that detail when DP is insufficient, and experiments on real data to show that our privacy guarantees are robust to underlying distributions that are not simple Markov chains.
更多查看译文
关键词
privacy,markov chains,local
AI 理解论文
溯源树
样例
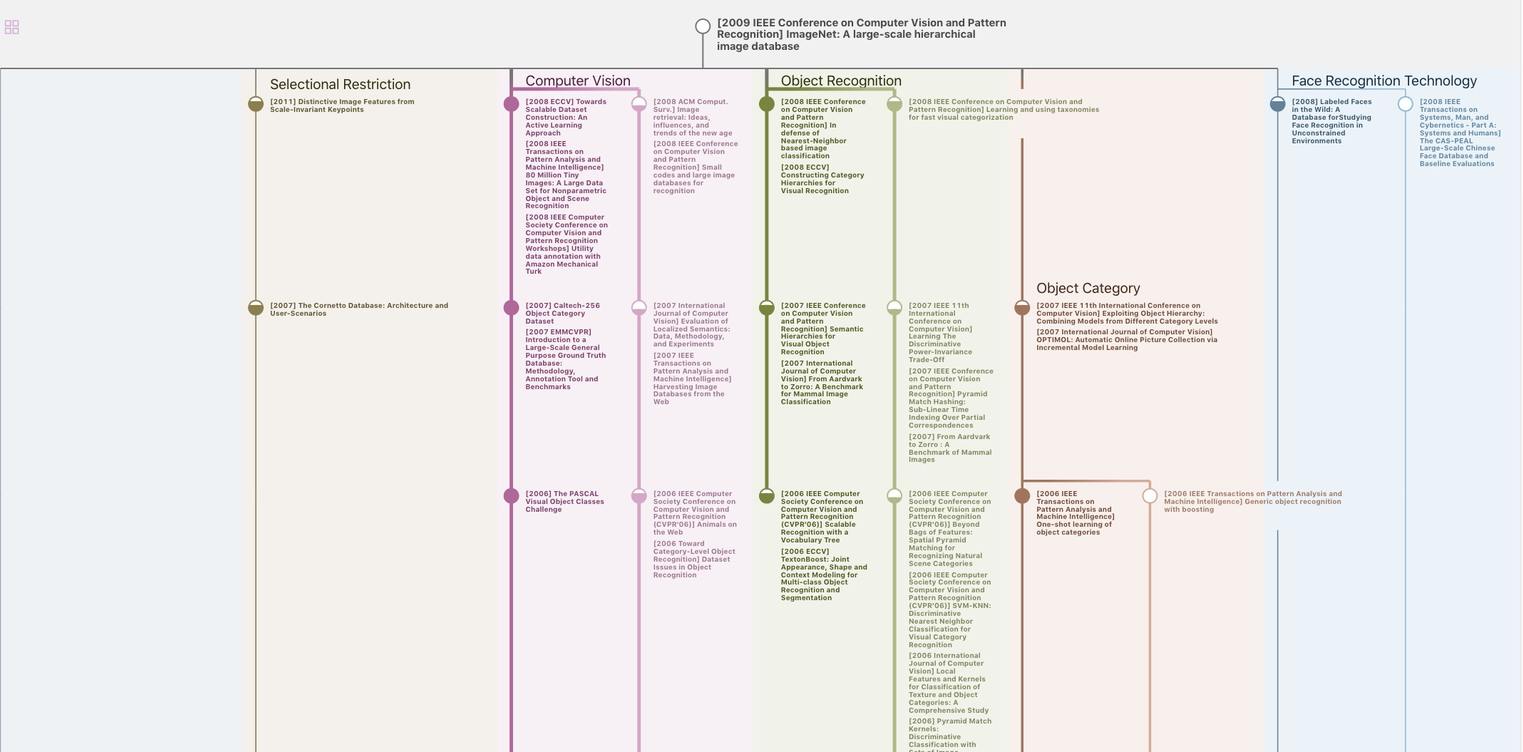
生成溯源树,研究论文发展脉络
Chat Paper
正在生成论文摘要