TERMS: textual emotion recognition in multidimensional space
Applied Intelligence(2022)
摘要
Microblogs generate a vast amount of data in which users express their emotions regarding almost all aspects of everyday life. Capturing affective content from these context-dependent and subjective texts is a challenging task. We propose an intelligent probabilistic model for textual emotion recognition in multidimensional space (TERMS) that captures the subjective emotional boundaries and contextual information embedded in a text for robust emotion recognition. It is implausible with discrete label assignment;therefore, the model employs a soft assignment by mapping varying emotional perceptions in a multidimensional space and generates them as distributions via the Gaussian mixture model (GMM). To strengthen emotion distributions, TERMS integrates a probabilistic emotion classifier that captures the contextual and linguistic information from texts. The integration of these aspects, the context-aware emotion classifier and the learned GMM parameters provide a complete coverage for accurate emotion recognition. The large-scale experimentation shows that compared to baseline and state-of-the-art models, TERMS achieved better performance in terms of distinguishability, prediction, and classification performance. In addition, TERMS provide insights on emotion classes, the annotation patterns, and the models application in different scenarios.
更多查看译文
关键词
Emotion recognition, Text classification, Valence-Arousal, Gaussian mixture model, Emotion distribution, Subjectivity
AI 理解论文
溯源树
样例
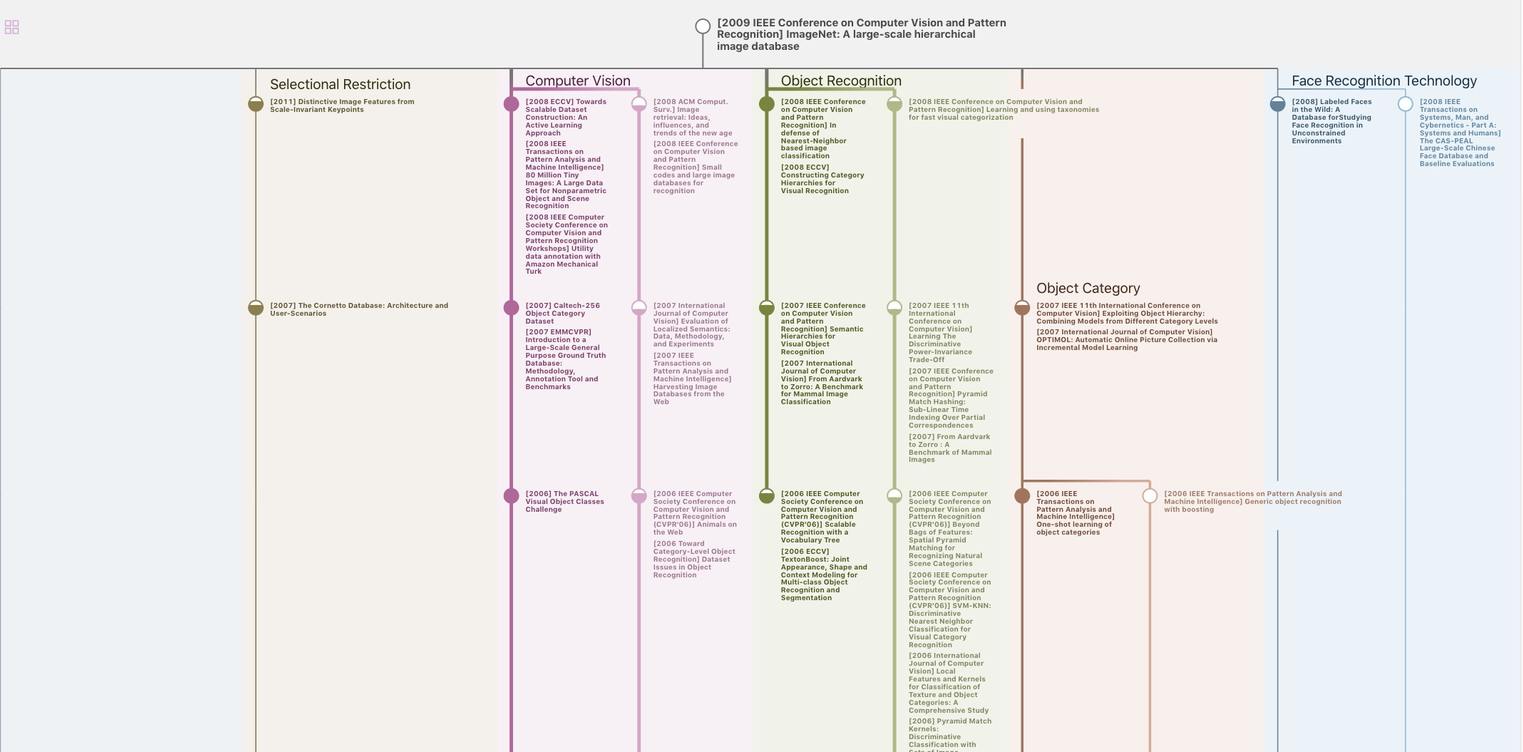
生成溯源树,研究论文发展脉络
Chat Paper
正在生成论文摘要