Mondrian forest for data stream classification under memory constraints
Data Mining and Knowledge Discovery(2024)
摘要
Supervised learning algorithms generally assume the availability of enough memory to store data models during the training and test phases. However, this assumption is unrealistic when data comes in the form of infinite data streams, or when learning algorithms are deployed on devices with reduced amounts of memory. In this paper, we adapt the online Mondrian forest classification algorithm to work with memory constraints on data streams. In particular, we design five out-of-memory strategies to update Mondrian trees with new data points when the memory limit is reached. Moreover, we design node trimming mechanisms to make Mondrian trees more robust to concept drifts under memory constraints. We evaluate our algorithms on a variety of real and simulated datasets, and we conclude with recommendations on their use in different situations: the Extend Node strategy appears as the best out-of-memory strategy in all configurations, whereas different node trimming mechanisms should be adopted depending on whether a concept drift is expected. All our methods are implemented in the OrpailleCC open-source library and are ready to be used on embedded systems and connected objects.
更多查看译文
关键词
Mondrian forest,Trimming,Concept drift,Data stream,Memory constraints
AI 理解论文
溯源树
样例
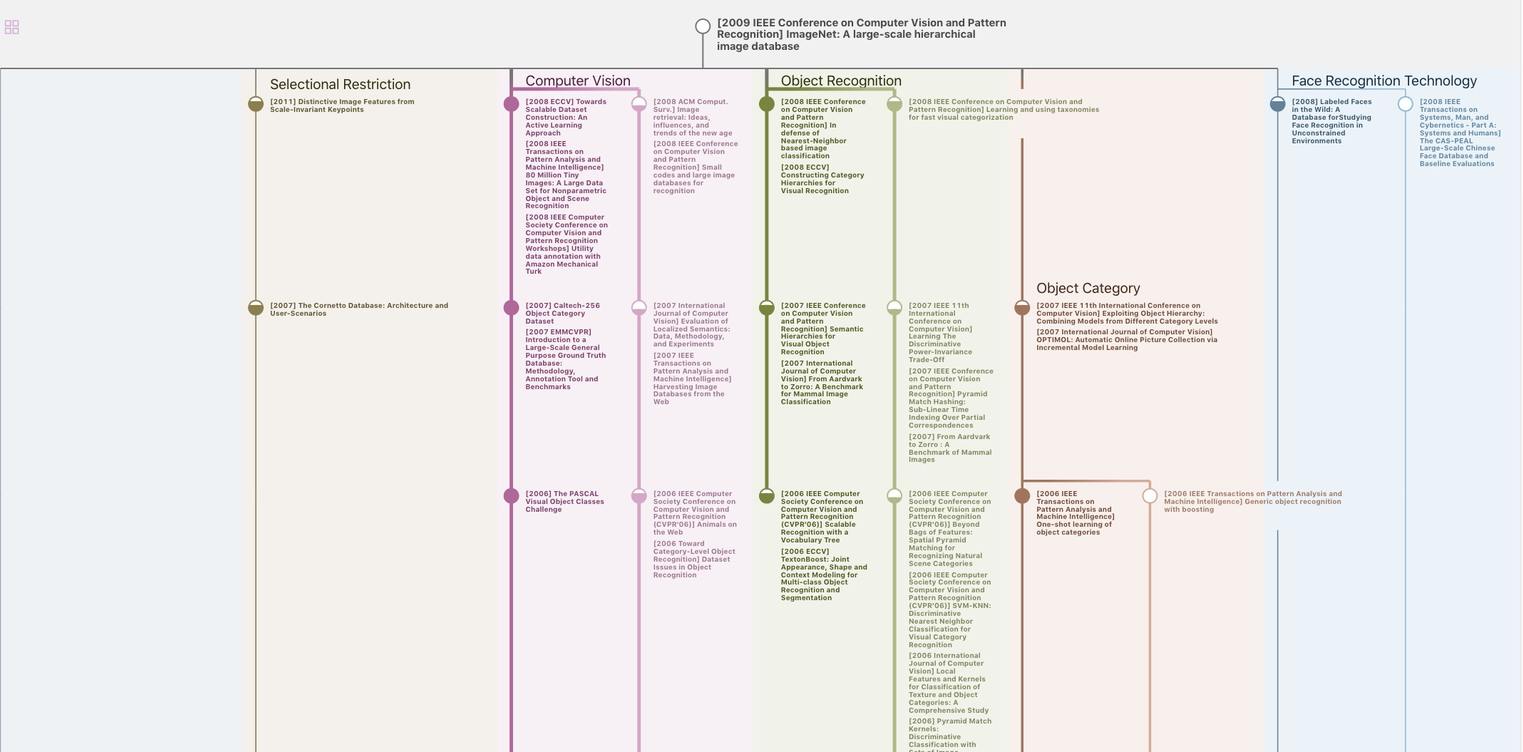
生成溯源树,研究论文发展脉络
Chat Paper
正在生成论文摘要