An efficient and secure recommendation system based on federated matrix factorization in digital economy
Personal and Ubiquitous Computing(2022)
摘要
The recommendation system is widely used in the digital economy to establish relationship between users and products. However, the data is usually scattered in different organizations in the form of “data islands” to protect privacy. To solve the above problem, we proposed an efficient and secure recommendation system based on federated matrix factorization, in which every user trains the model locally with their own data and uploads the calculated gradient vector instead of the original data. In the scheme, one server verifies the aggregation results transmitted by other servers and completes the model update without revealing user privacy. Additionally, users are allowed to drop out during the training process, and each participant’s behavior will be recorded to calculate the rewards or punishments. Finally, we evaluated the performance from different perspectives and proved its feasibility.
更多查看译文
关键词
Digital economy,Recommendation system,Federated learning,Matrix factorization
AI 理解论文
溯源树
样例
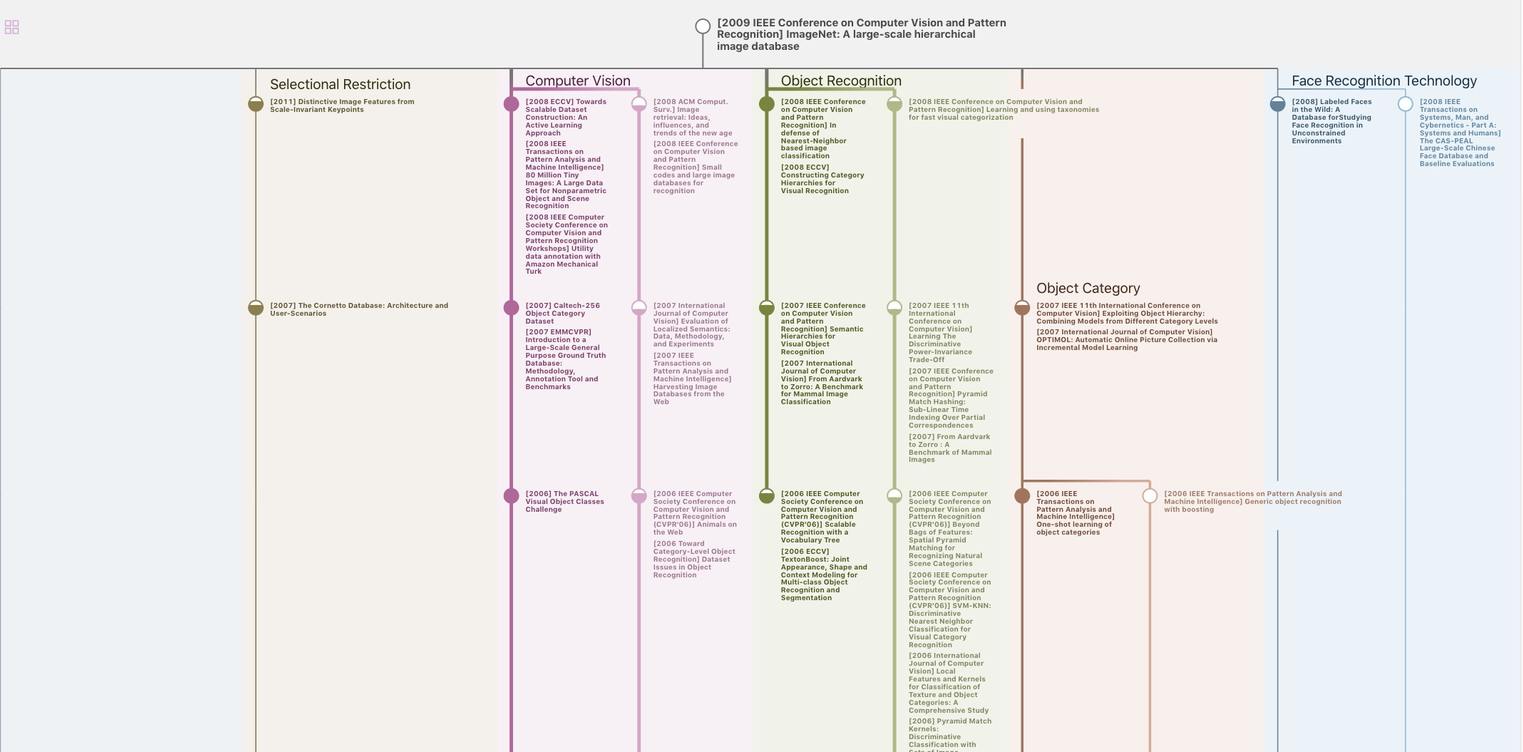
生成溯源树,研究论文发展脉络
Chat Paper
正在生成论文摘要