Towards Lossless ANN-SNN Conversion under Ultra-Low Latency with Dual-Phase Optimization
arXiv (Cornell University)(2022)
摘要
Spiking neural networks (SNNs) operating with asynchronous discrete events
show higher energy efficiency with sparse computation. A popular approach for
implementing deep SNNs is ANN-SNN conversion combining both efficient training
of ANNs and efficient inference of SNNs. However, the accuracy loss is usually
non-negligible, especially under a few time steps, which restricts the
applications of SNN on latency-sensitive edge devices greatly. In this paper,
we first identify that such performance degradation stems from the
misrepresentation of the negative or overflow residual membrane potential in
SNNs. Inspired by this, we decompose the conversion error into three parts:
quantization error, clipping error, and residual membrane potential
representation error. With such insights, we propose a two-stage conversion
algorithm to minimize those errors respectively. Besides, We show each stage
achieves significant performance gains in a complementary manner. By evaluating
on challenging datasets including CIFAR-10, CIFAR- 100 and ImageNet, the
proposed method demonstrates the state-of-the-art performance in terms of
accuracy, latency and energy preservation. Furthermore, our method is evaluated
using a more challenging object detection task, revealing notable gains in
regression performance under ultra-low latency when compared to existing
spike-based detection algorithms. Codes are available at
https://github.com/Windere/snn-cvt-dual-phase.
更多查看译文
关键词
ann-snn,ultra-low,dual-phase
AI 理解论文
溯源树
样例
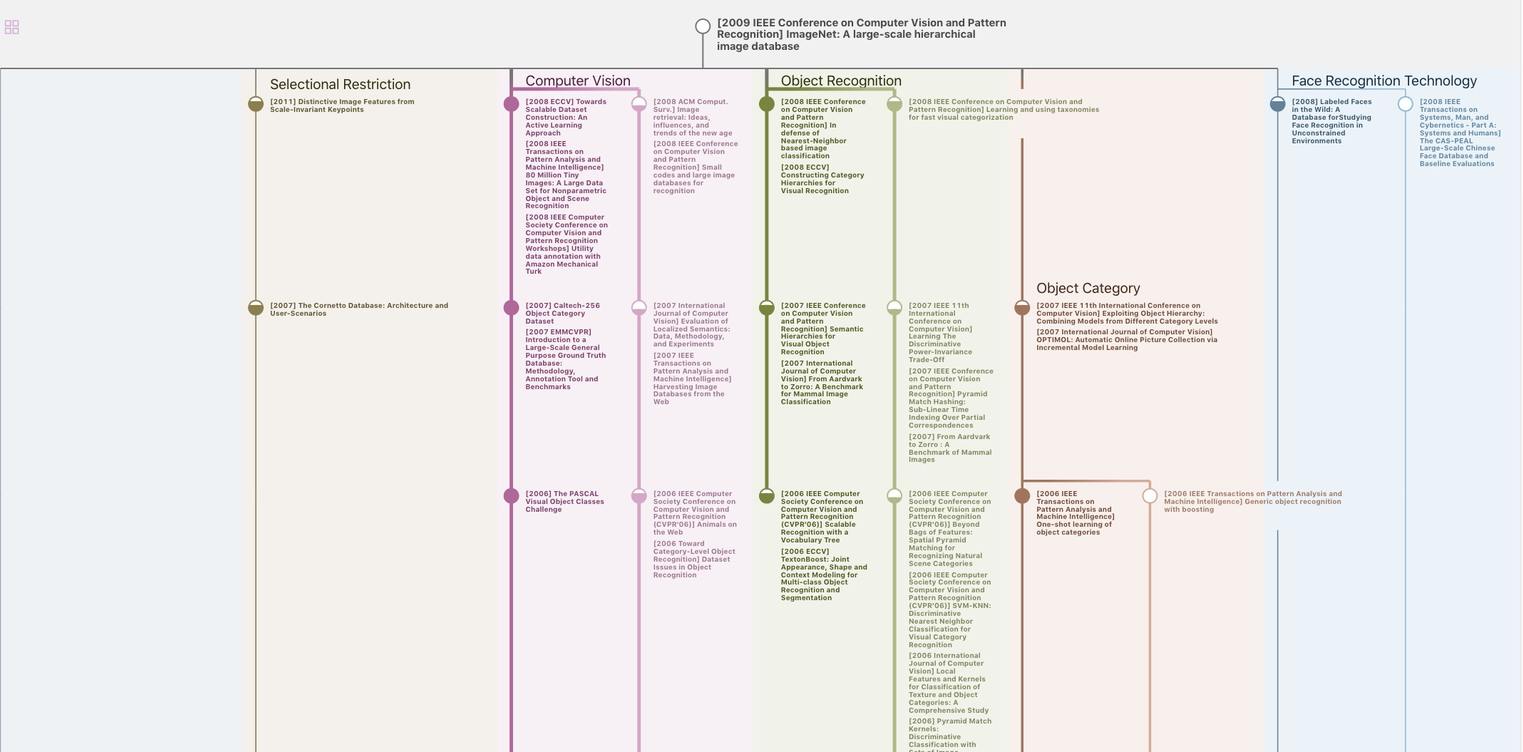
生成溯源树,研究论文发展脉络
Chat Paper
正在生成论文摘要