Exploring Generalizability of Fine-Tuned Models for Fake News Detection
arxiv(2022)
摘要
The Covid-19 pandemic has caused a dramatic and parallel rise in dangerous misinformation, denoted an `infodemic' by the CDC and WHO. Misinformation tied to the Covid-19 infodemic changes continuously; this can lead to performance degradation of fine-tuned models due to concept drift. Degredation can be mitigated if models generalize well-enough to capture some cyclical aspects of drifted data. In this paper, we explore generalizability of pre-trained and fine-tuned fake news detectors across 9 fake news datasets. We show that existing models often overfit on their training dataset and have poor performance on unseen data. However, on some subsets of unseen data that overlap with training data, models have higher accuracy. Based on this observation, we also present KMeans-Proxy, a fast and effective method based on K-Means clustering for quickly identifying these overlapping subsets of unseen data. KMeans-Proxy improves generalizability on unseen fake news datasets by 0.1-0.2 f1-points across datasets. We present both our generalizability experiments as well as KMeans-Proxy to further research in tackling the fake news problem.
更多查看译文
关键词
COVID 19,Transformers,Fake news,Clustering
AI 理解论文
溯源树
样例
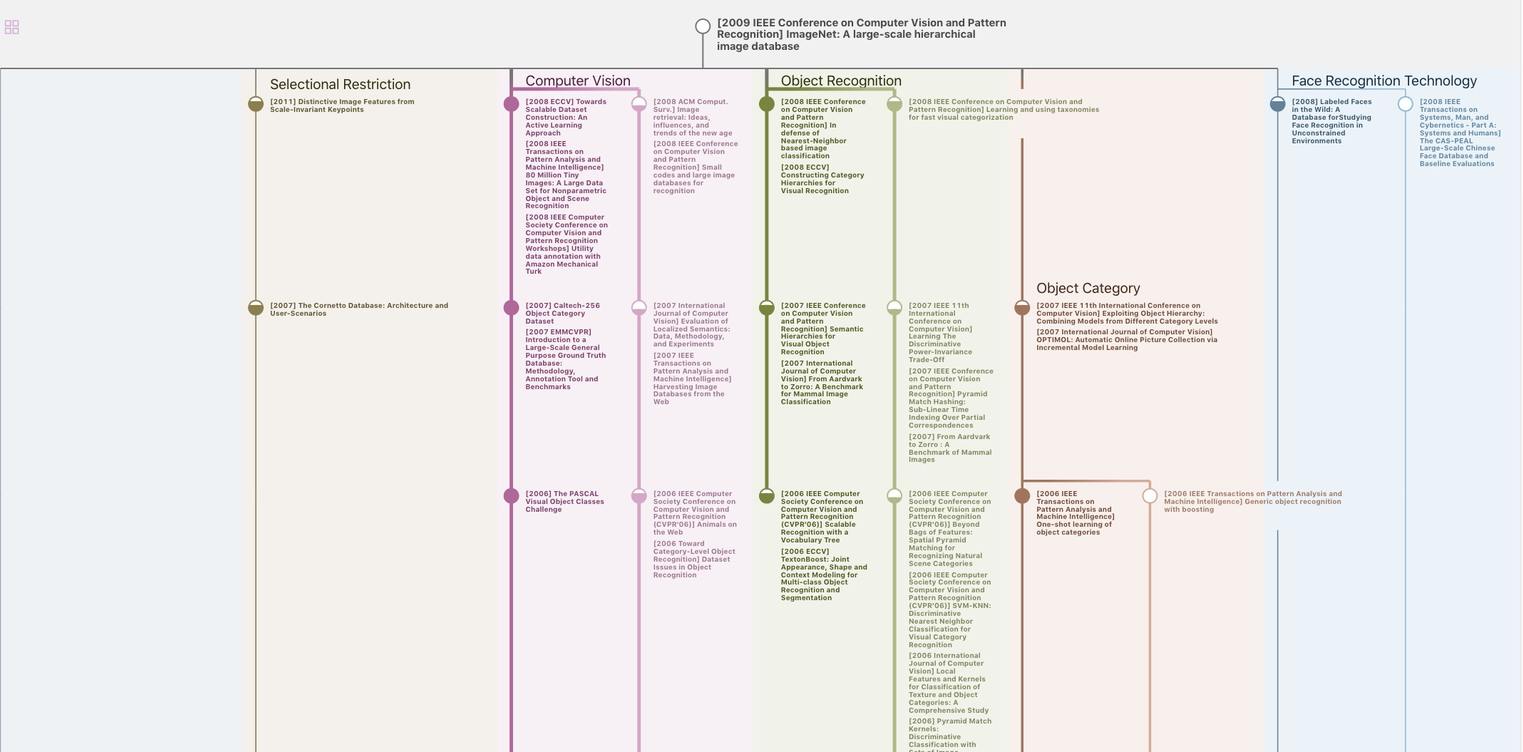
生成溯源树,研究论文发展脉络
Chat Paper
正在生成论文摘要