Robust Regularized Low-Rank Matrix Models for Regression and Classification
arxiv(2022)
摘要
While matrix variate regression models have been studied in many existing works, classical statistical and computational methods for the analysis of the regression coefficient estimation are highly affected by high dimensional and noisy matrix-valued predictors. To address these issues, this paper proposes a framework of matrix variate regression models based on a rank constraint, vector regularization (e.g., sparsity), and a general loss function with three special cases considered: ordinary matrix regression, robust matrix regression, and matrix logistic regression. We also propose an alternating projected gradient descent algorithm. Based on analyzing our objective functions on manifolds with bounded curvature, we show that the algorithm is guaranteed to converge, all accumulation points of the iterates have estimation errors in the order of $O(1/\sqrt{n})$ asymptotically and substantially attaining the minimax rate. Our theoretical analysis can be applied to general optimization problems on manifolds with bounded curvature and can be considered an important technical contribution to this work. We validate the proposed method through simulation studies and real image data examples.
更多查看译文
关键词
classification,models,regression,low-rank
AI 理解论文
溯源树
样例
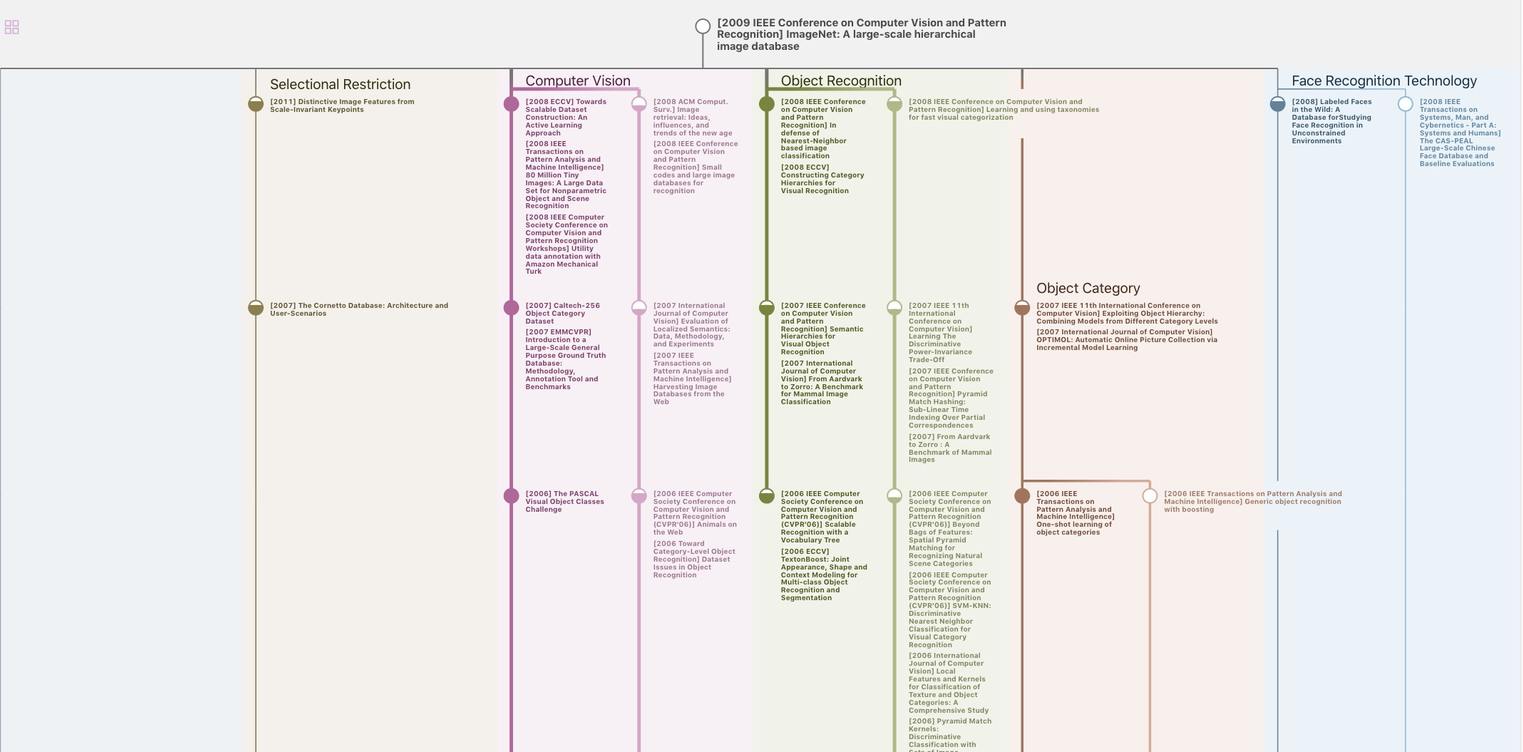
生成溯源树,研究论文发展脉络
Chat Paper
正在生成论文摘要