A Hierarchical Data Driven Method for Spatial Electric Load Forecasting
2021 International Conference on Power System Technology (POWERCON)(2021)
摘要
Spatial Load Forecasting (SLF) is the basis for distribution network planning. Most of the previous SLF methods, including empirical load density index method and the top-down simulation method, fail to give sufficient accurate forecasts on the quantity and distribution of the future load growth. Motivated by the increasing data sources and widely fulfilled land use planning in cities, a data driven approach for SLF is proposed in this paper. Firstly, a hierarchical bottom-up load accumulating approach is utilized based on clustered typical load profiles. Then land plots are described with multiple indices including intensity, development, climate and location, and the numerical spatial load densities of different land use types are obtained through a nonparametric kernel density estimation. Finally, a machine learning method is introduced to forecast the future plot load. The proposed data driven approach can greatly enhance the accuracy and operability of SLF for distributed network planning.
更多查看译文
关键词
Spatial Load Forecast,data driven,Machine learning,clustering,load density label
AI 理解论文
溯源树
样例
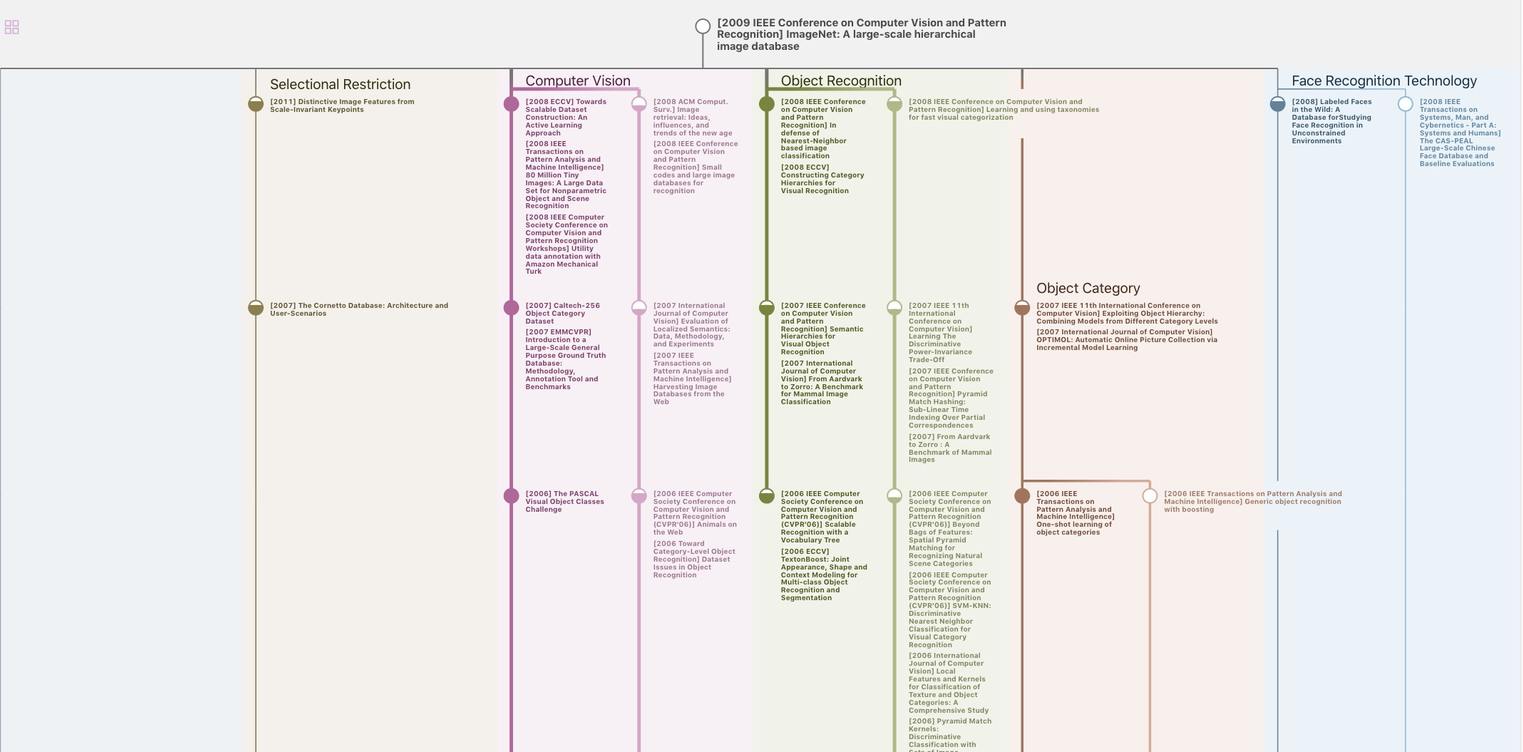
生成溯源树,研究论文发展脉络
Chat Paper
正在生成论文摘要