Advances in modeling emerging magnetoresistive random access memories: from finite element methods to machine learning approaches
International Conference on Micro- and Nano-Electronics 2021(2022)
摘要
Emerging spin transfer torque magnetoresistive random access memories (STT MRAM) are nonvolatile and offer high speed and endurance. They are promising for stand-alone and embedded applications in the automotive industry, microcontrollers, Internet of Things, frame buffer memory, and slow SRAM. The MRAM cell usually includes a CoFeB fixed reference layer and a free magnetic layer (FL), separated by a tunnel barrier. To design ultra-scaled MRAM cells it is necessary to accurately model the torques acting on the magnetization in composite magnetic layers with one or several nonmagnetic inclusions between the ferromagnetic parts. The magnetization dynamics is governed by the Landau-Lifshitz- Gilbert (LLG) equation supplemented with the corresponding torques. The torques depend on nonequilibrium spin accumulation generated by an electric current. The electric current and the spin accumulation also depend on the magnetization. Therefore, the LLG and the spin-charge transport equations are coupled and must be solved simultaneously. We apply the finite element method (FEM) to numerically solve this coupled system of partial differential equations. To develop an open source solver, we use well-developed C++ FEM libraries. The computationally most expensive part is the demagnetizing field calculation. It is performed by a hybrid finite element-boundary element method. This confines the simulation domain for the field evaluation to the ferromagnets only. Advanced compression algorithms for large, dense matrices are used to optimize the performance of the demagnetizing field calculation in complex structures. To evaluate the torques acting on the magnetization, a coupled spin and charge transport approach is implemented. For the computation of the torques acting in a magnetic tunnel junction (MTJ), a magnetization-dependent resistivity of the tunnel barrier is introduced. A fully three-dimensional solution of the equations is performed to accurately model the torques acting on the magnetization. The use of a unique set of equations for the whole memory cell including the FL, fixed layer, contacts, and nonmagnetic spacers is one of the advantages of our approach. To incorporate the temperature increase due to the electric current, we solve the heat transport equation coupled to the electron, spin, and magnetization dynamics, and we demonstrate that the FL temperature is highly inhomogeneous due to a non-uniform magnetization of the FL during switching. Spin-orbit torque (SOT) MRAM is fast-switching and thus well suitable for caches. By means of micromagnetic simulations, we demonstrate the purely electrical switching of a perpendicular FL by the SOTs due to two orthogonal short current pulses. To further optimize the pulse sequence, a machine learning approach based on reinforcement learning is employed. Importantly, a neural network trained on a fixed material parameter set achieves switching for a wide range of material parameter variations.
更多查看译文
AI 理解论文
溯源树
样例
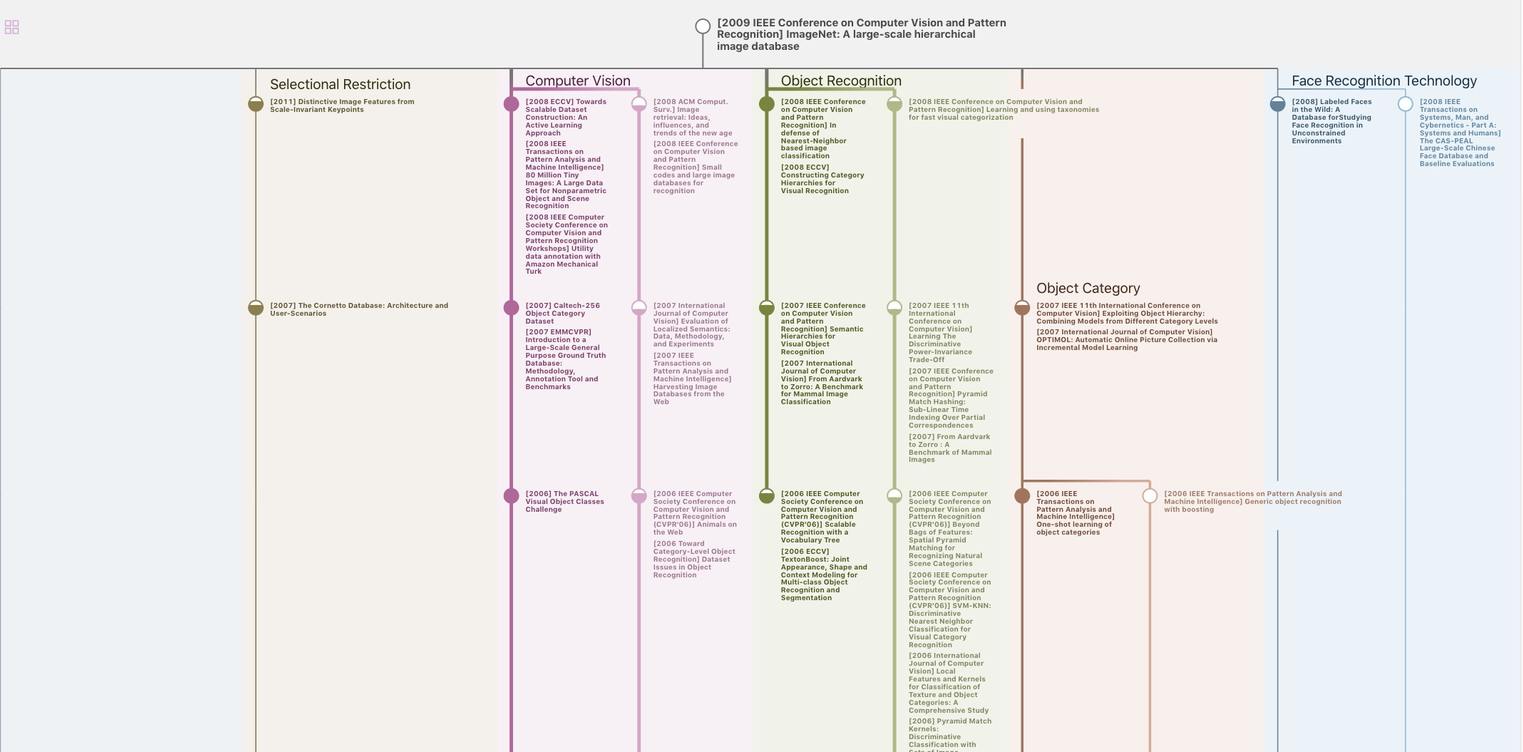
生成溯源树,研究论文发展脉络
Chat Paper
正在生成论文摘要