Development of computational tools for evaluating differential protein expression relative to spatial oxygen gradients using imaging mass cytometry
Poster Presentations - Late-Breaking Proffered Abstracts(2021)
摘要
The solid tumor microenvironment is highly heterogeneous, conferring numerous differences in tumor cell gene expression and phenotype. One predominant driver of this heterogeneity is variable oxygenation. Low oxygen, termed hypoxia, has been shown to increase metastasis, radiation resistance, and lead to an overall poor patient prognosis. Characterizing hypoxia in patients can be used to personalize an adjuvant therapy targeting resistant hypoxic cell populations, which may otherwise survive conventional therapies. Because of the spatial heterogeneity intrinsic to hypoxia, current approaches to measure hypoxia, such as using polarographic oxygen electrodes, may underestimate the severity of hypoxia. Endogenous markers such as CA9 and VEGFA provide some semblance of evaluating hypoxia, however expression of such markers is not always solely driven by oxygenation. Multiple markers in tandem may prove to be a more reliable metric for evaluating hypoxia than individual markers alone. Here, we present an open-source collection of QuPath and Python scripts for analyzing differential protein expression relative to hypoxia gradients for imaging mass cytometry (IMC) data. IMC enables highly multiplexed imaging of tissue sections at the single cell resolution, with no spectral overlap of immunohistochemical markers. This is achieved through substitution of fluorophores with unique isotopes of heavy metals, and a mass spectrometer in place of a conventional fluorescent microscope. Previous work in our lab has identified 17 markers with extensive heterogeneity in clinical glioblastoma multiforme (GBM) patient samples. Pimonidazole, an exogenous hypoxia probe, has been administered to these patients, and is detectable through immunohistochemical techniques such as IMC. Serving as our ground truth for hypoxia, we conduct a differential gene expression analysis across regions of relative hypoxia for all markers in our panel, using a variety of supervised and unsupervised tools available in the open-source digital pathology platform QuPath, and through custom data visualization and statistical analysis tools built in Python. As such, we present an end-to-end image analysis workflow for evaluating hypoxia-regulated differential protein expression of clinical GBM cases imaged through IMC. This method is applicable for evaluating differential gene expression across multiple disease sites, multiplexed imaging modalities, and histomorphological regions of interest. Such computational methods enable high throughput biomarker discovery, while also being used to elucidate novel oxygen-dependent biological pathways. Citation Format: Mark Zaidi, Phoebe Lombard, Sheila Mansouri, Gelareh Zadeh, Trevor D McKee, Bradly G Wouters. Development of computational tools for evaluating differential protein expression relative to spatial oxygen gradients using imaging mass cytometry [abstract]. In: Proceedings of the AACR-NCI-EORTC Virtual International Conference on Molecular Targets and Cancer Therapeutics; 2021 Oct 7-10. Philadelphia (PA): AACR; Mol Cancer Ther 2021;20(12 Suppl):Abstract nr LBA029.
更多查看译文
AI 理解论文
溯源树
样例
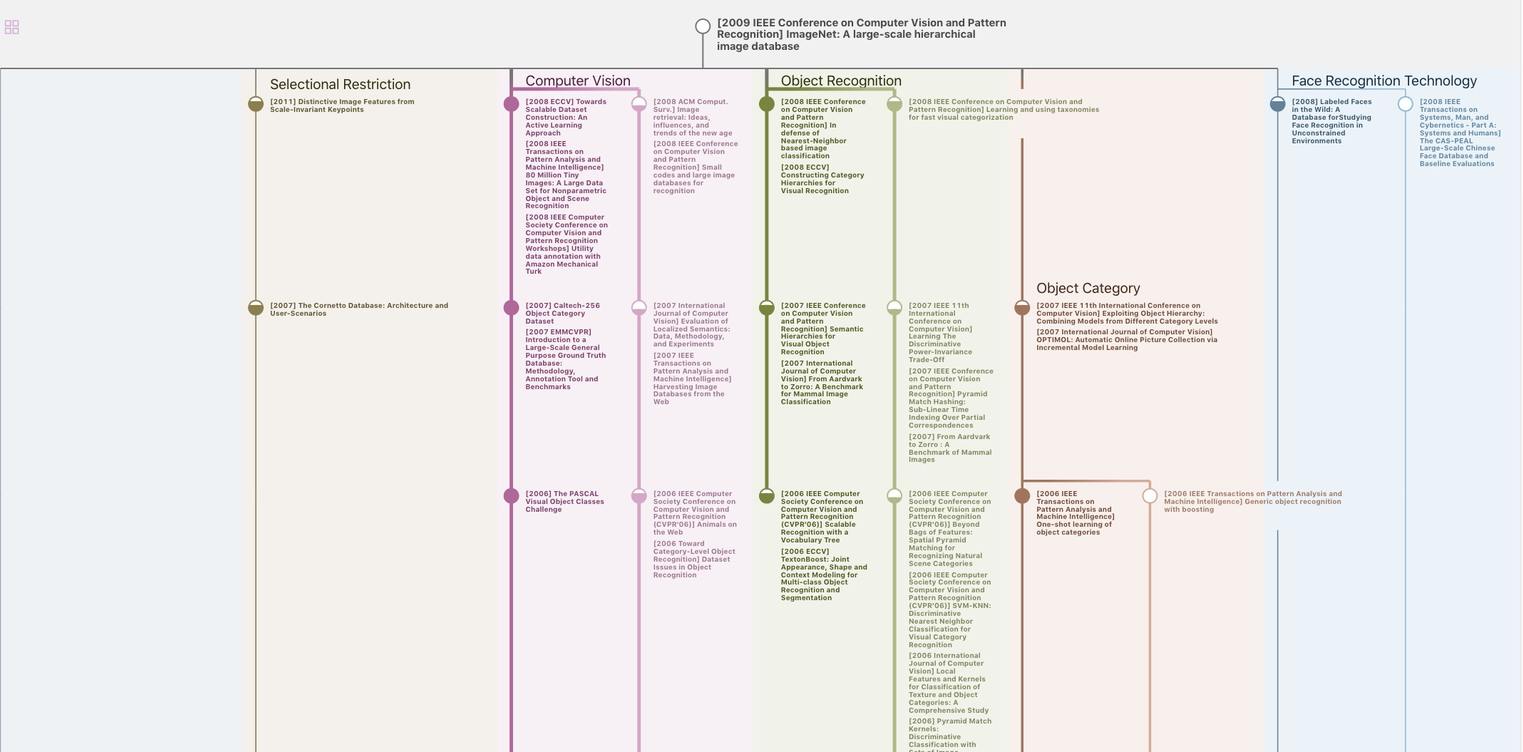
生成溯源树,研究论文发展脉络
Chat Paper
正在生成论文摘要