Resolving large conformational change pathways through machine learning and enhanced sampling simulations
Biophysical Journal(2022)
摘要
Despite the tremendous growth of computational power, atomistic molecular dynamics simulations of slow biological processes, like protein folding, are still prohibitively expensive. Over the years, many enhanced simulation methods have been developed to alleviate this issue. Many of them, however, rely on users to predefine a set of relevant collective variables that properly describe the process being studied, which is often difficult to identify beforehand. Here, we present a deep learning method that learns the optimal set of collective variables automatically from short equilibrium and steered molecular dynamics simulations, and at the same time provides an initial guess of the transition pathway.
更多查看译文
AI 理解论文
溯源树
样例
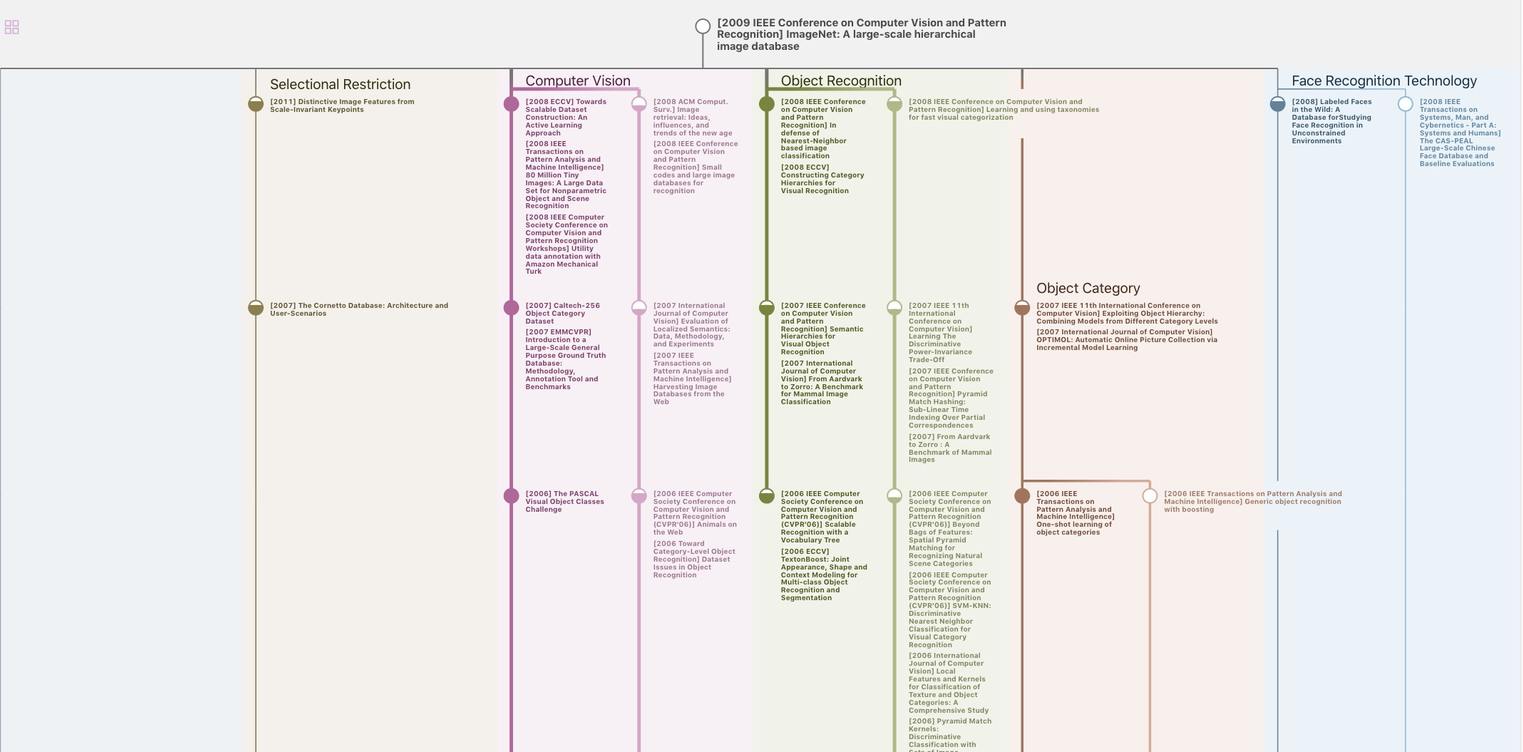
生成溯源树,研究论文发展脉络
Chat Paper
正在生成论文摘要