Towards an interpretable autoencoder: A decision tree-based autoencoder and its application in anomaly detection
IEEE Transactions on Dependable and Secure Computing(2022)
摘要
The importance of understanding and explaining the associated classification results in the utilization of artificial intelligence (AI) in many different practical applications has contributed to the trend of moving away from black-box AI towards explainable AI (XAI). In this paper, we propose the first interpretable autoencoder based on decision trees, which is designed to handle categorical data without the need to transform the data representation. Furthermore, our proposed interpretable autoencoder provides a natural explanation for experts in the application area. The experimental findings show that our proposed interpretable autoencoder is among the top-ranked anomaly detection algorithms, along with one-class SVM and Gaussian Mixture. More specifically, our proposal is on average 2\\% below the best Area Under the Curve (AUC) result and 3\\% over the other Average Precision scores, in comparison to One-class SVM, Isolation Forest, Local Outlier Factor, Elliptic Envelope, Gaussian Mixture Model, and eForest.
更多查看译文
关键词
Interpretable artificial intelligence,autoencoder,decision tree,anomaly detection,explainable artificial intelligence (XAI)
AI 理解论文
溯源树
样例
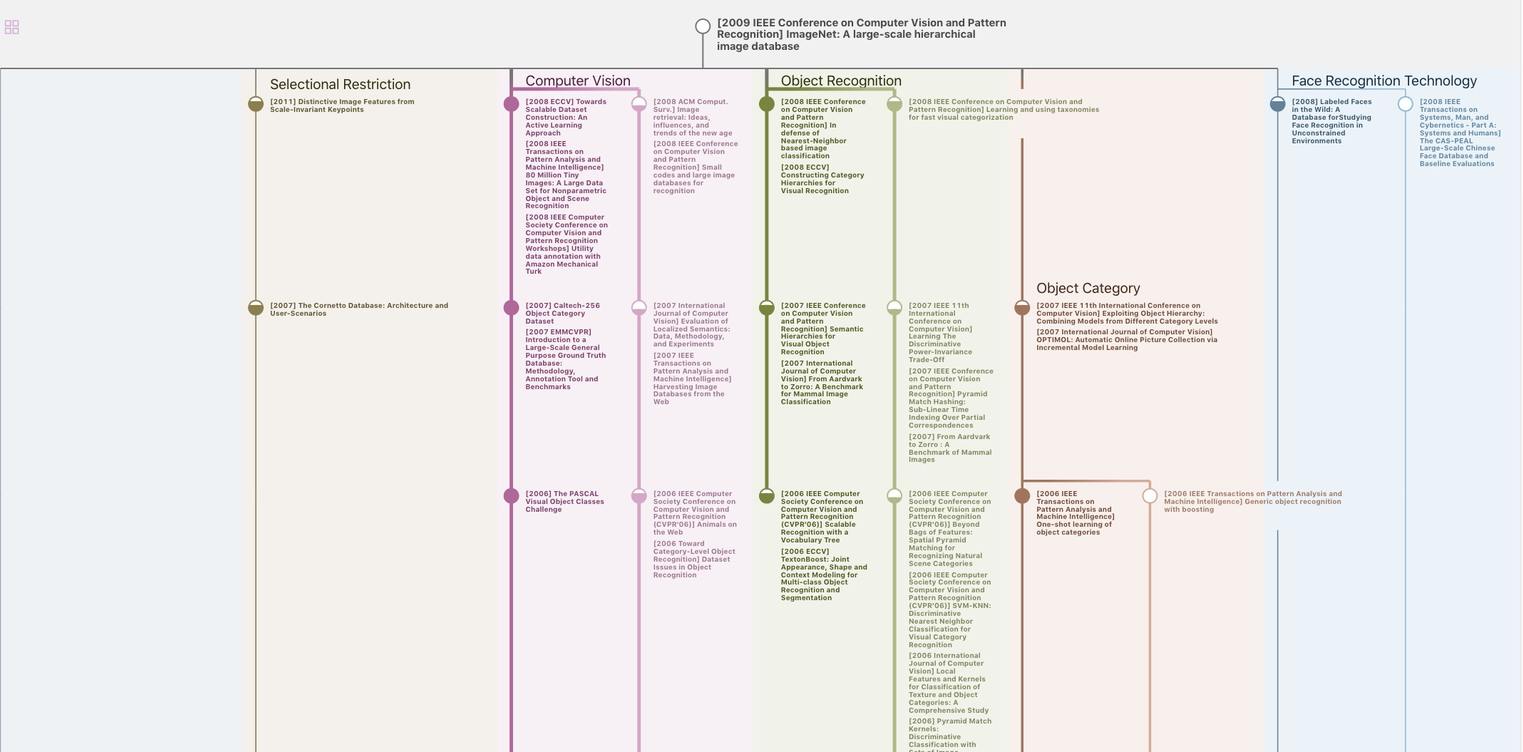
生成溯源树,研究论文发展脉络
Chat Paper
正在生成论文摘要