PGDENet: Progressive Guided Fusion and Depth Enhancement Network for RGB-D Indoor Scene Parsing.
IEEE Transactions on Multimedia(2023)
摘要
Scene parsing is a fundamental task in computer vision. Various RGB-D (color and depth) scene parsing methods based on fully convolutional networks have achieved excellent performance. However, color and depth information are different in nature and existing methods cannot optimize the cooperation of high-level and low-level information when aggregating modal information, which introduces noise or loss of key information in the aggregated features and generates inaccurate segmentation maps. The features extracted from the depth branch are weak because of the low quality of the depth map, which results in unsatisfactory feature representation. To address these drawbacks, we propose a progressive guided fusion and depth enhancement network (PGDENet) for RGB-D indoor scene parsing. First, high-quality RGB images are used to improve depth data through a depth enhancement module, in which the depth maps are strengthened in terms of channel and spatial correlations. Then, we integrate information from the RGB and enhance depth modalities using a progressive complementary fusion module, in which we start with high-level semantic information and move down layerwise to guide the fusion of adjacent layers while reducing hierarchy-based differences. Extensive experiments are conducted on two public indoor scene datasets, and the results show that the proposed PGDENet outperforms state-of-the-art methods in RGB-D scene parsing.
更多查看译文
关键词
Cross-level information,depth enhancement,modality-specific fusion,progressive guided fusion,RGB-D indoor scene parsing
AI 理解论文
溯源树
样例
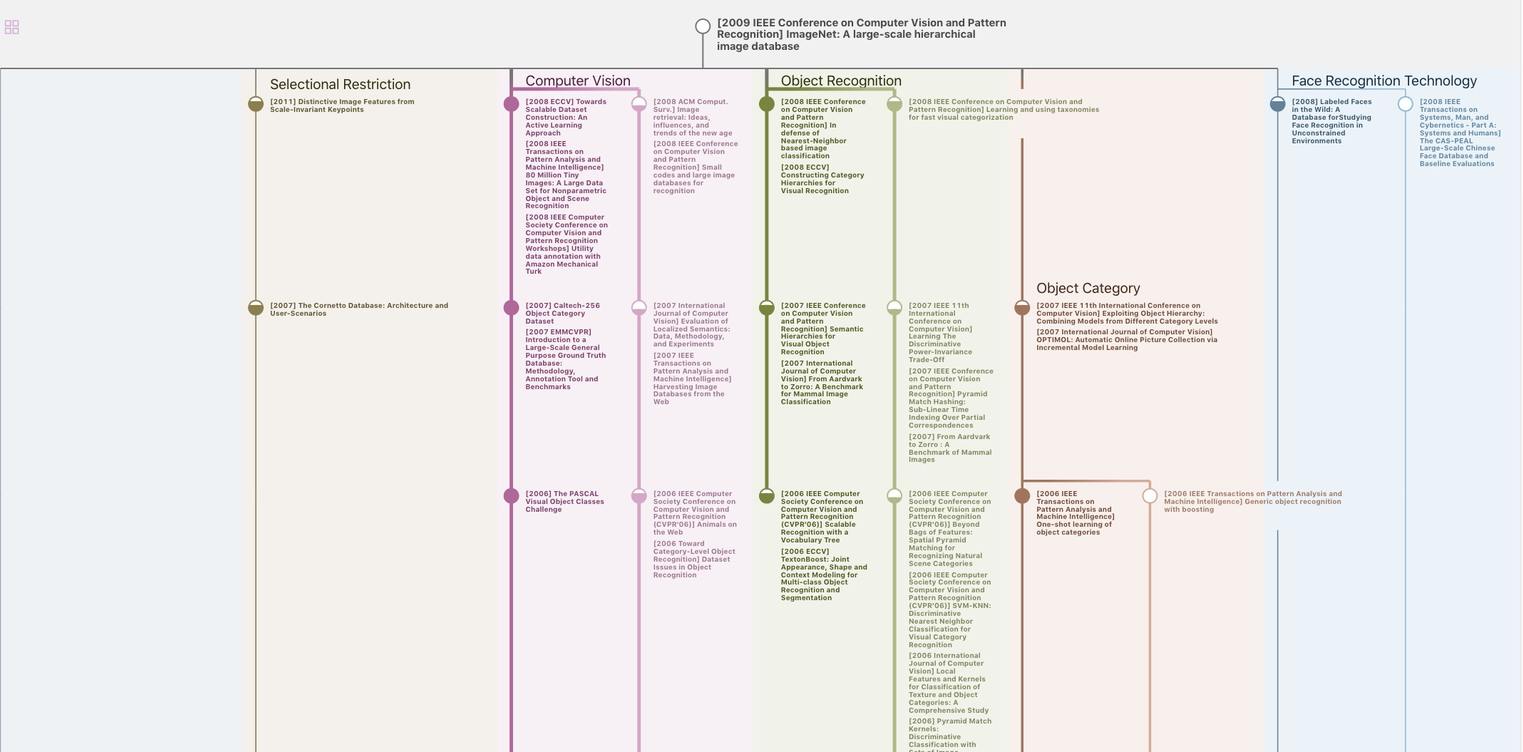
生成溯源树,研究论文发展脉络
Chat Paper
正在生成论文摘要