Preemptive Identification of End-of-Life for Stationary Battery Systems
2021 IEEE 2nd International Conference on Smart Technologies for Power, Energy and Control (STPEC)(2021)
摘要
Stationary Battery Systems (SBS) are becoming a critical component in power distribution networks across the world. Penetration of intermittent renewable energy sources is a huge influence on the requirement of SBS. SBSs are also used in other applications such as peak load management, load-shifting, voltage regulation, and power quality improvement. With the increase in penetration of SBS, the requirement for predicting the End-of-Life (EOL) of these batteries is also increasing drastically. Machine-Learning (ML) based models are widely used for such predictions in various applications. With minimal resource requirements and the capability to leverage smart meter/sensor data, ML approaches provide flexibility in predicting EOL. In this paper, the study on the preemptive identification of EOL for lithium-ion batteries utilizing historical data is presented. Various ML methods are used to predict the EOL of the batteries; with a goal to predict the EOL at least 30 cycles in advance. The prediction is aimed to enable timely maintenance and replacement of the batteries effectively. The performance of the EOL prediction with the different methods that can improve the accuracy is presented as an analysis. Correlation to the data from a practical Battery Management System is also presented to demonstrate the practical applicability.
更多查看译文
关键词
stationary battery system,Machine Learning,end-of-life,lithium ion batteries,charge/discharge capacity
AI 理解论文
溯源树
样例
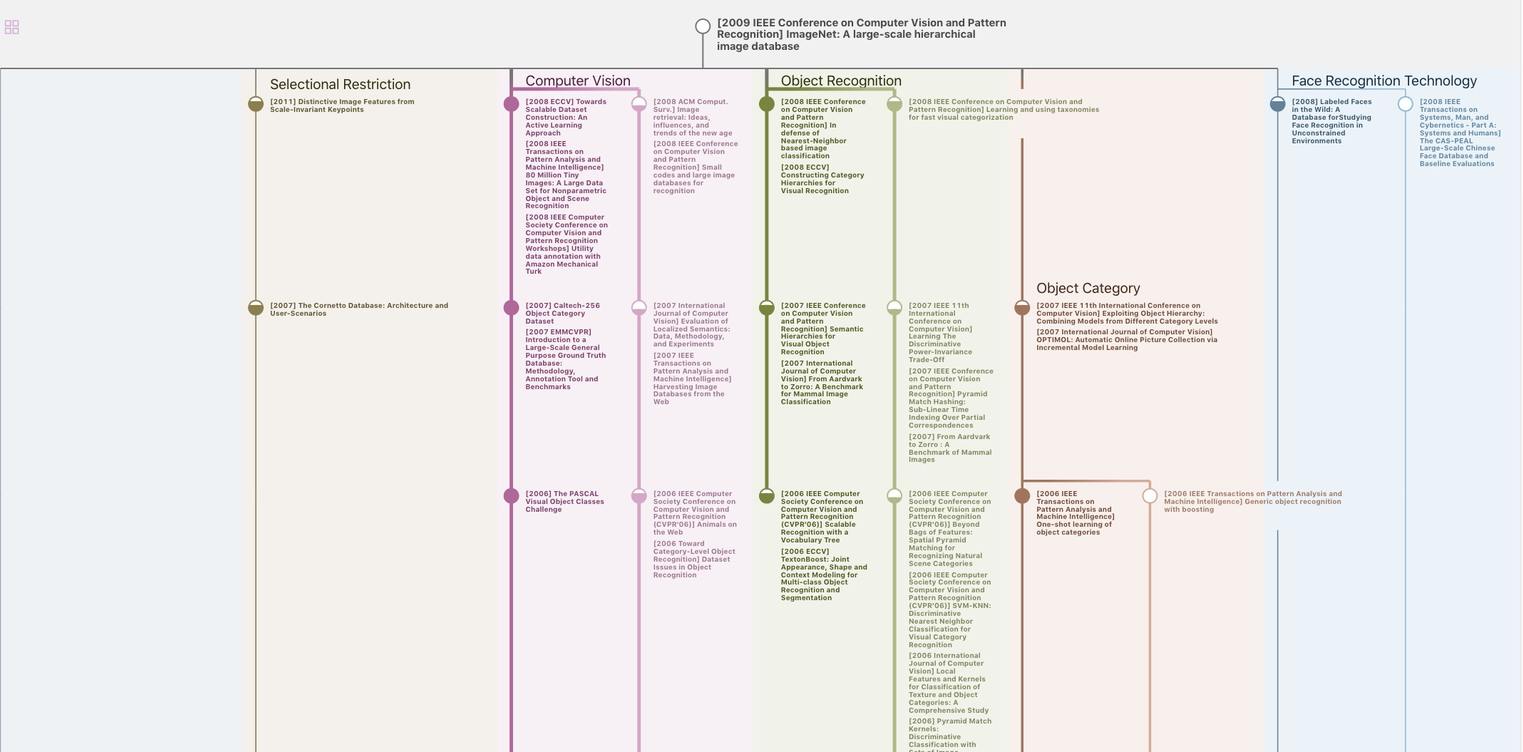
生成溯源树,研究论文发展脉络
Chat Paper
正在生成论文摘要