An Efficient and Robust Cloud-Based Deep Learning With Knowledge Distillation
IEEE Transactions on Cloud Computing(2023)
摘要
In recent years, deep neural networks have shown extraordinary power in various practical learning tasks, especially in object detection, classification, natural language processing. However, deploying such large models on resource-constrained devices or embedded systems is challenging due to their high computational cost. Efforts such as model partition, pruning, or quantization have been used at the expense of accuracy loss. Knowledge distillation is a technique that transfers model knowledge from a well-trained model (teacher) to a smaller and shallow model (student). Instead of using a learning model on the cloud, we can deploy distilled models on various edge devices, significantly reducing the computational cost, memory usage and prolonging the battery lifetime. In this work, we propose a novel neuron manifold distillation (NMD) method, where the student models imitate the teacher's output distribution and learn the feature geometry of the teacher model. In addition, to further improve the cloud-based learning system reliability, we propose a confident prediction mechanism to calibrate the model predictions. We conduct experiments with different distillation configurations over multiple datasets. Our proposed method demonstrates a consistent improvement in accuracy-speed trade-offs for the distilled model.
更多查看译文
关键词
Deep learning,transfer learning,knowledge distillation
AI 理解论文
溯源树
样例
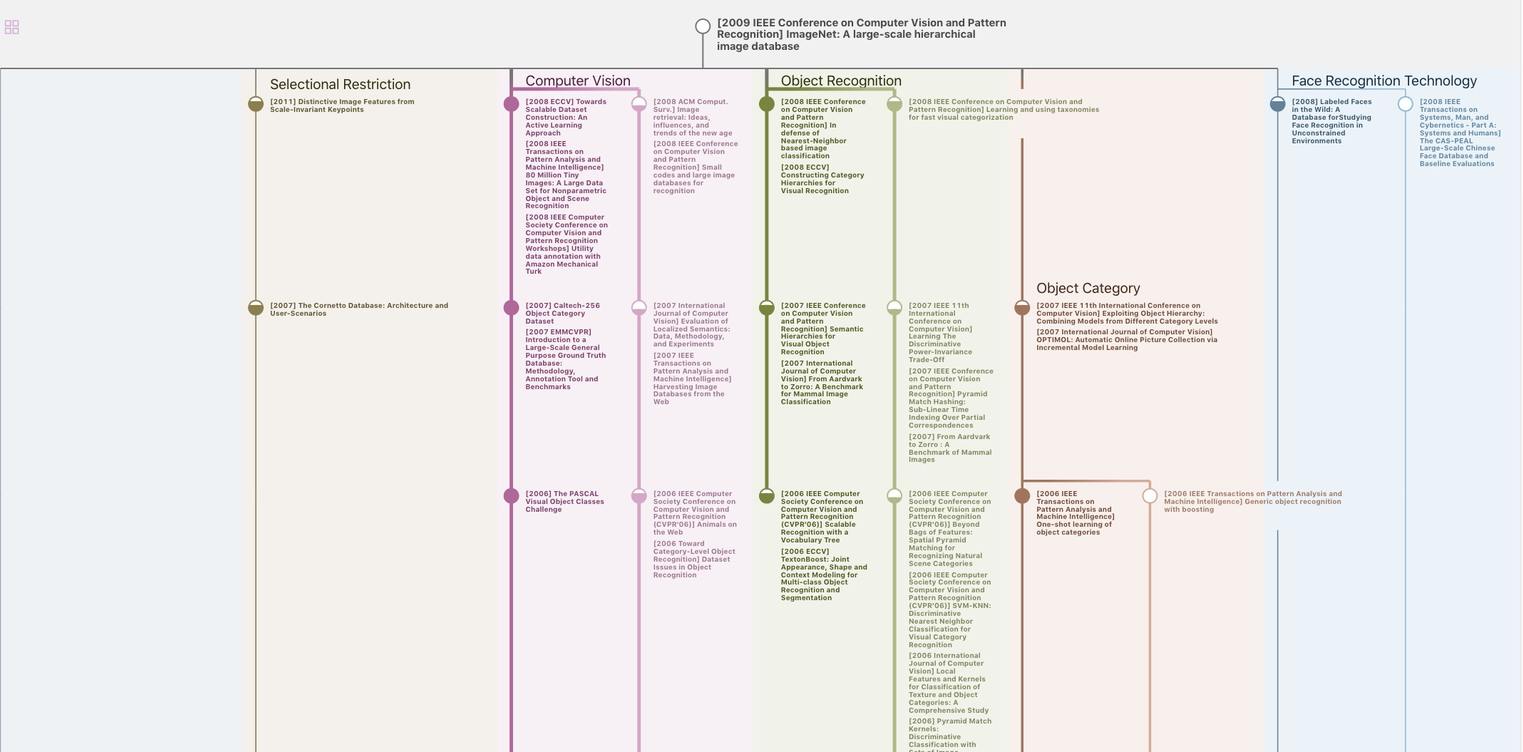
生成溯源树,研究论文发展脉络
Chat Paper
正在生成论文摘要