Optimization of time-multiplexed computer-generated holograms with surrogate gradients
Emerging Digital Micromirror Device Based Systems and Applications XIV(2022)
摘要
Gradient descent is an efficient algorithm to optimize differentiable functions with continuous variables, yet it is not suitable for computer generated holography (CGH) with binary light modulators. To address this, we replaced binary pixel values with continuous variables that are binarized with a thresholding operation, and we introduced gradients of the sigmoid function as surrogate gradients to ensure the differentiability of the binarization step. We implemented this method both to directly optimize binary holograms, and to train deep learning-based CGH models. Simulations and experimental results show that our method achieves greater speed, and higher accuracy and contrast than existing algorithms.
更多查看译文
AI 理解论文
溯源树
样例
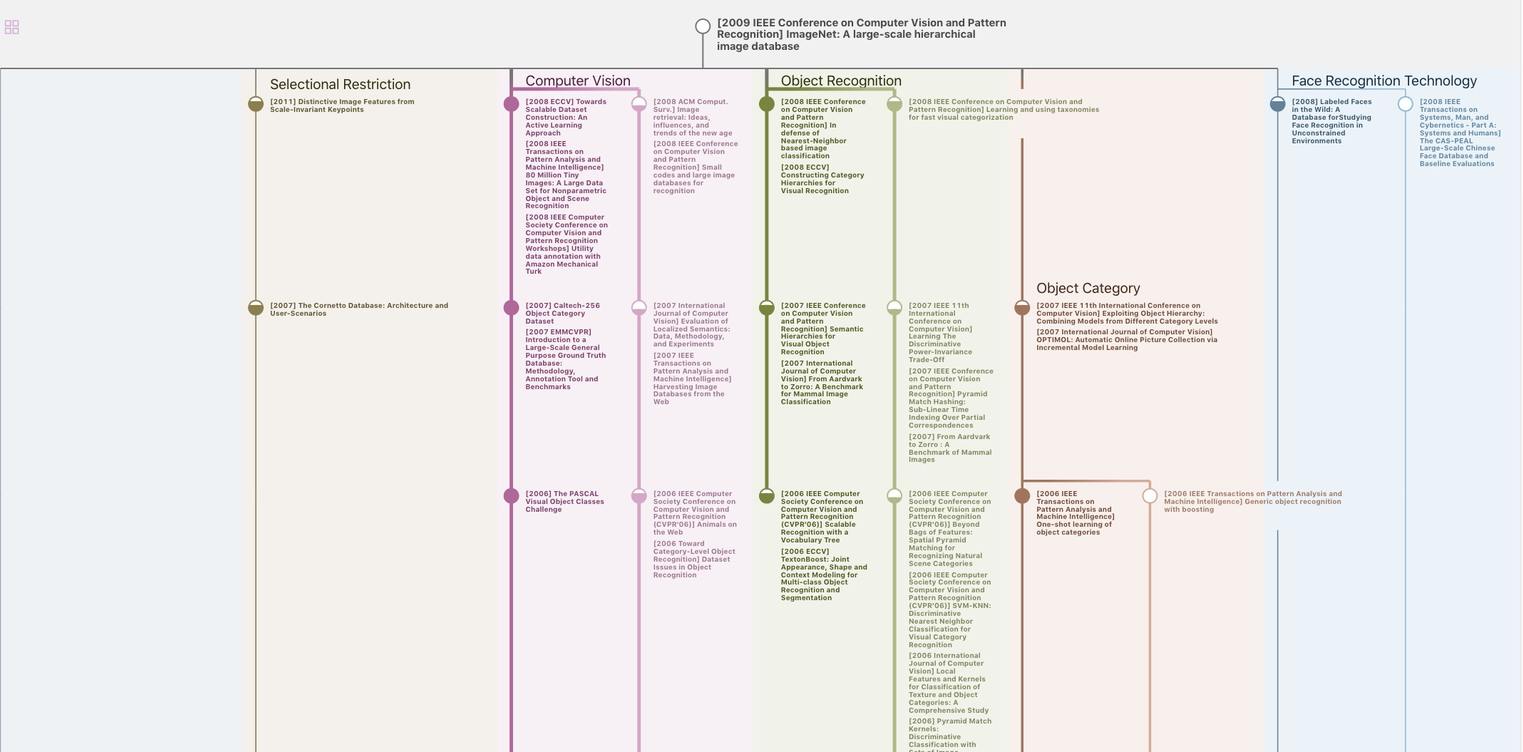
生成溯源树,研究论文发展脉络
Chat Paper
正在生成论文摘要