Correspondence Attention Transformer: A Context-Sensitive Network for Two-View Correspondence Learning
IEEE TRANSACTIONS ON MULTIMEDIA(2023)
摘要
Seeking reliable correspondences then recovering camera poses from a set of putative correspondences extracted from two images of the same scene is a fundamental problem in computer vision. Recent advances have demonstrated that this problem can be effectively solved by using a deep architecture based on the multi-layer perceptron, where the context normalization is designed to make the network permutation-equivariant and embed global information in the sparse point data. However, the context normalization simply normalizes the feature maps according to their distribution and treats each correspondence equally, leading to difficulties in adequately capturing scene geometry encoded by the inliers, especially in case of severe outliers. To address this issue, this paper designs a context-sensitive network based on the self-attention mechanism, termed as correspondence attention transformer (CAT), to enhance the consistent geometry information of inliers and simultaneously suppress outliers during embedding global information. In particular, we design an attentionstyle structure to aggregate features from all correspondences, i.e., a spatial attention namely CAT-S, which provides each correspondence with information exchange from others in the putative set. To capture the contextual information in a more comprehensive and robust way, we also introduce a multi-head mechanism in our structure to exploit the geometrical context from different aspects. Moreover, considering the high memory request in spatial attention, we propose a covariance normalized channel attention CAT-C in our framework, which can largely reduce the memory consumption and parameter scale, but it asks for eigenvalue decomposition in each attention block thus resulting in more runtime. Anyway, these two attention mechanisms can realize information exchange from the spatial or channel aspect, which both contribute to constructing the geometrical context between inliers and encourage the network to pay more attention to the feature subset about potential inliers. Extensive experiments have been conducted over both indoor and outdoor datasets on the tasks of camera pose estimation, outlier removal, and image registration, which demonstrate the superiority of our method that realizes a large performance improvement compared with the current state-of-the-art approaches.
更多查看译文
关键词
Task analysis,Feature extraction,Transformers,Memory management,Information exchange,Geometry,Cameras,Feature matching,deep learning,context-sensitive,self-attention
AI 理解论文
溯源树
样例
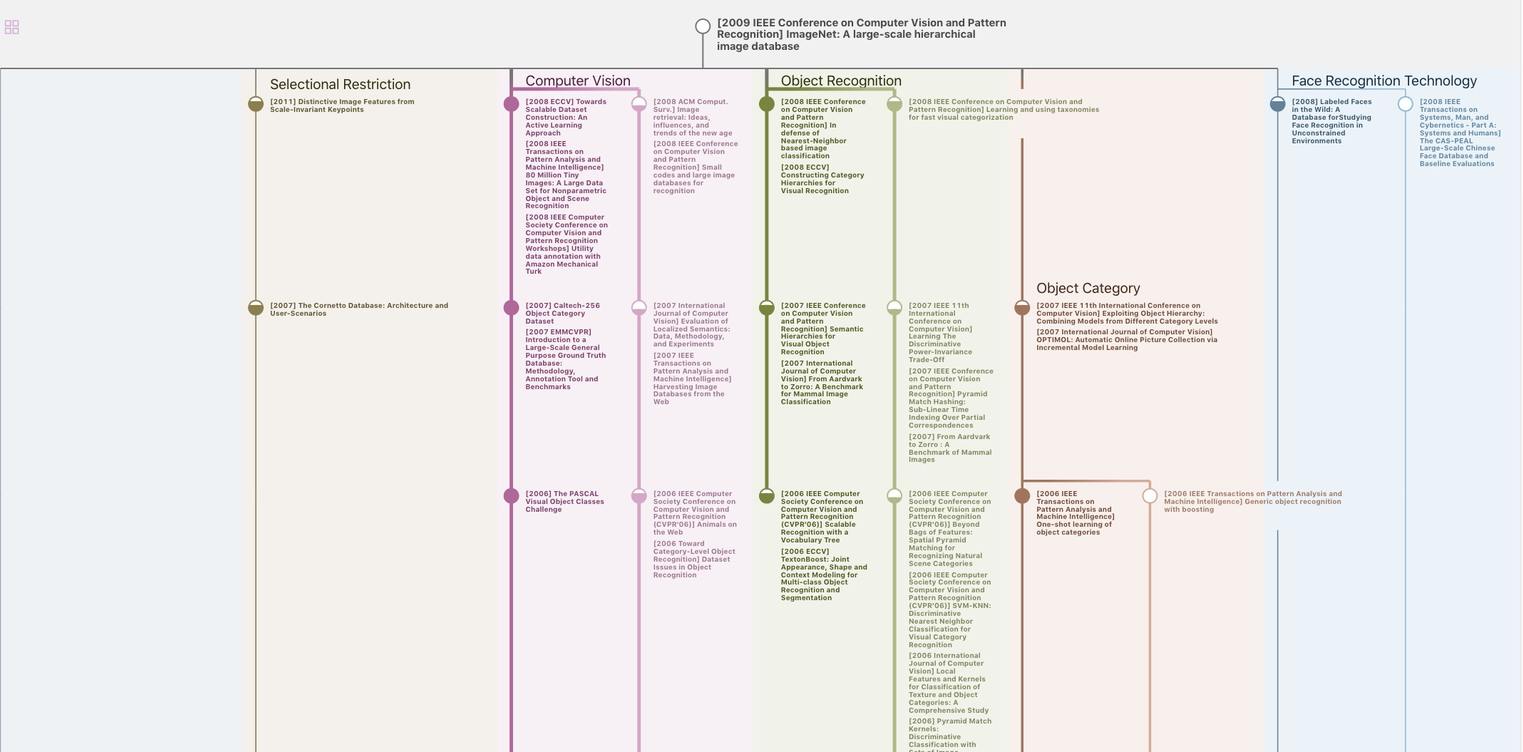
生成溯源树,研究论文发展脉络
Chat Paper
正在生成论文摘要