Improved Representation Learning For Acoustic Event Classification Using Tree-Structured Ontology
ICASSP 2022 - 2022 IEEE International Conference on Acoustics, Speech and Signal Processing (ICASSP)(2022)
摘要
Acoustic events have a hierarchical structure analogous to a tree (or a directed acyclic graph). In this work, we propose a structure-aware semi-supervised learning framework for acoustic event classification (AEC). Our hypothesis is that the audio label structure contains useful information that is not available in audios and plain tags. We show that by organizing audio representations with a human-curated tree ontology, we can improve the quality of the learned audio representations for downstream AEC tasks. We use consistency training to use large amounts of unlabeled data for structured representation manifold learning. Experimental results indicate that our framework learns high quality representations which enable us to achieve comparable performance in discriminative tasks as fully supervised baselines. Moreover, our framework can better handle audios with unseen tags by confidently assigning a super-category (internal node like "animal" in Fig. 1) tag to the audio.
更多查看译文
关键词
Acoustic event classification,Representation learning,Audio ontology,Decision tree
AI 理解论文
溯源树
样例
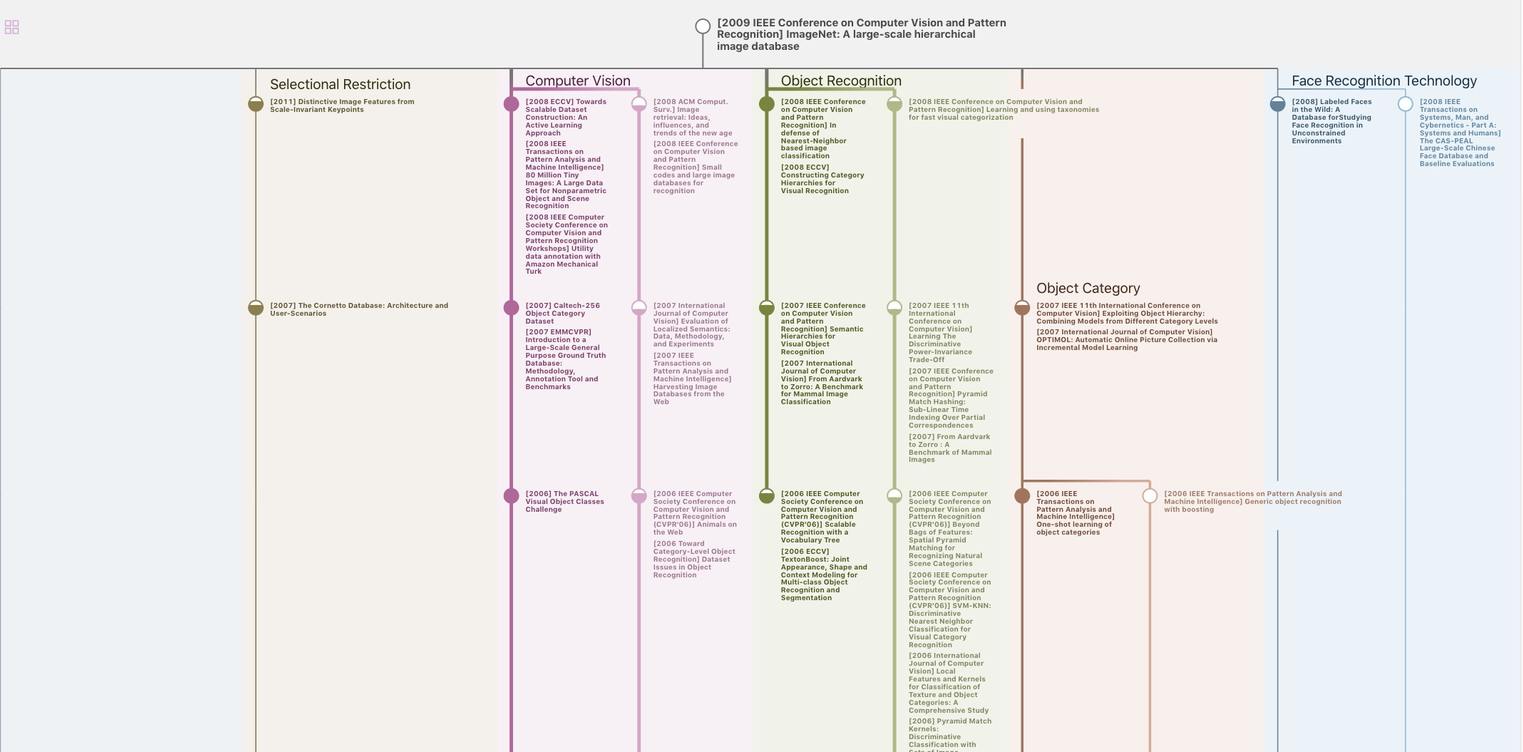
生成溯源树,研究论文发展脉络
Chat Paper
正在生成论文摘要