Information Criteria for Outlier Detection Avoiding Arbitrary Significance Levels
ECONOMETRICS AND STATISTICS(2024)
摘要
Information criteria for model choice are extended to the detection of outliers in regression models. For deletion of observations (hard trimming) the family of models is generated by monitoring properties of the fitted models as the trimming level is varied. For soft trim-ming (downweighting of observations), some properties are monitored as the efficiency or breakdown point of the robust regression is varied. Least Trimmed Squares and the For-ward Search are used to monitor hard trimming, with MM-and S-estimation the methods for soft trimming. Bayesian Information Criteria (BIC) for both scenarios are developed and results about their asymptotic properties provided. In agreement with the theory, simula-tions and data analyses show good performance for the hard trimming methods for outlier detection. Importantly, this is achieved very simply, without the need to specify either sig-nificance levels or decision rules for multiple outliers.Crown Copyright (c) 2022 Published by Elsevier B.V. on behalf of EcoSta Econometrics and Statistics. All rights reserved.
更多查看译文
关键词
automatic data analysis,Bayesian Information Criterion (BIC),Forward Search,Least Trimmed Squares,MM -estimation,S -estimation
AI 理解论文
溯源树
样例
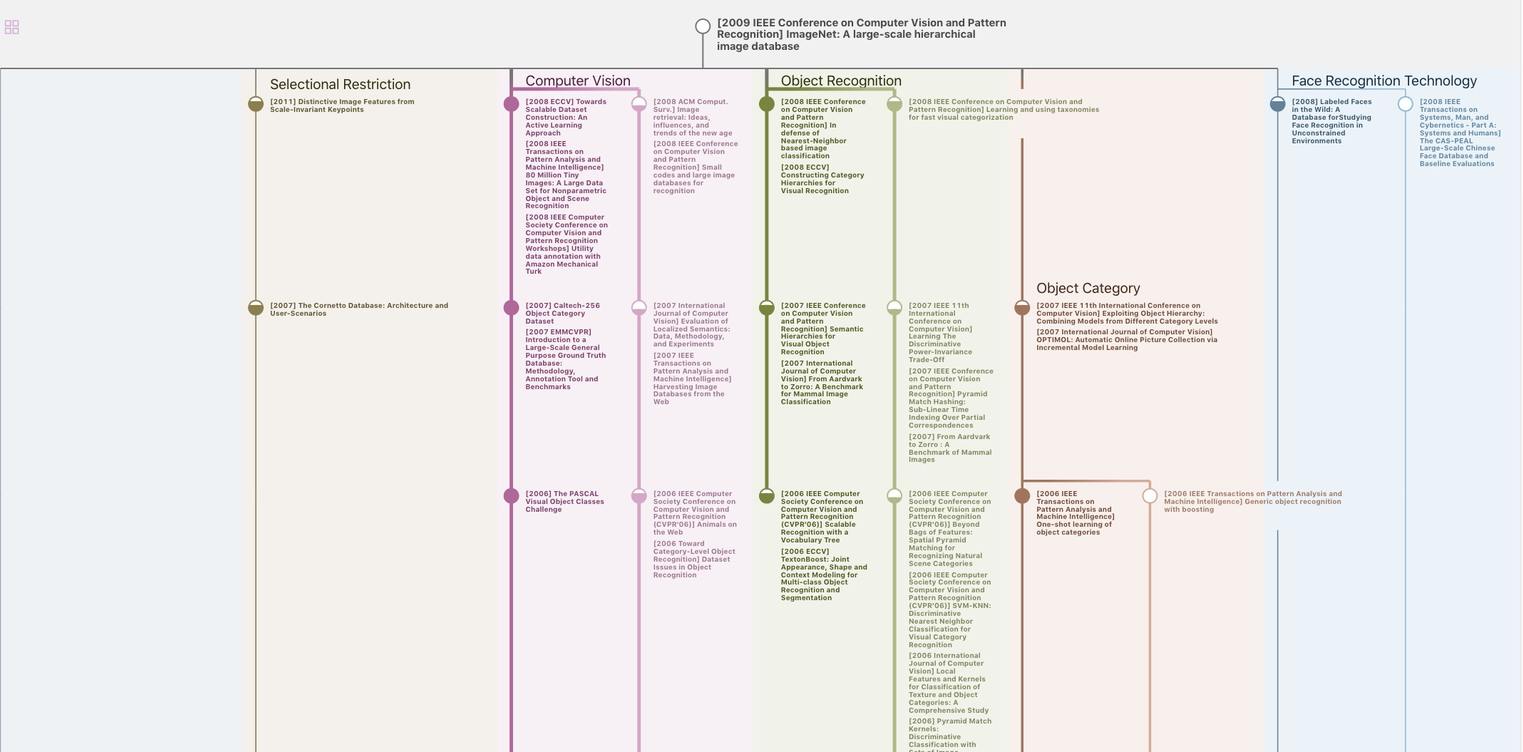
生成溯源树,研究论文发展脉络
Chat Paper
正在生成论文摘要