Multivariate prediction of retail sales by multi-task time series learning
International Conference on Computer Graphics, Artificial Intelligence, and Data Processing (ICCAID 2021)(2022)
摘要
Predicting retail sales is a hot research topic that can help firms achieve on-demand procurement. That can reduce the extra costs caused by an inventory shortage or surpluses. Traditional methods usually regard the sales of a certain product as the independent time series and then solve the task by a curve fitting model. However, the sales volume is not a single value, but the one aggregated from many types of products. There are correlations between different types of product sales. The increase in one type of product sales may lead to changes in sales in another type. Without capturing the correlation between different product sales, it is difficult to obtain satisfactory performance. To solve this problem, we propose a new multi-task method based on multivariate time series learning. Considering there are multiple product types and each type has different trend characteristics, we first cluster the time series of all product types and then model each as a multivariate prediction task. We then design a new dilated convolution network to fusion the features of related products and the trend characteristic of each task. Moreover, we develop a trend structural entropy network to grasp the fluctuation features of the task. A new self-enhancement mechanism is proposed to finely capture the correlations among tasks. Through multi-task learning, the model can effectively improve prediction accuracy by using the complementary information of closely related time series. Experimental results on two real datasets show the effectiveness of our approach.
更多查看译文
AI 理解论文
溯源树
样例
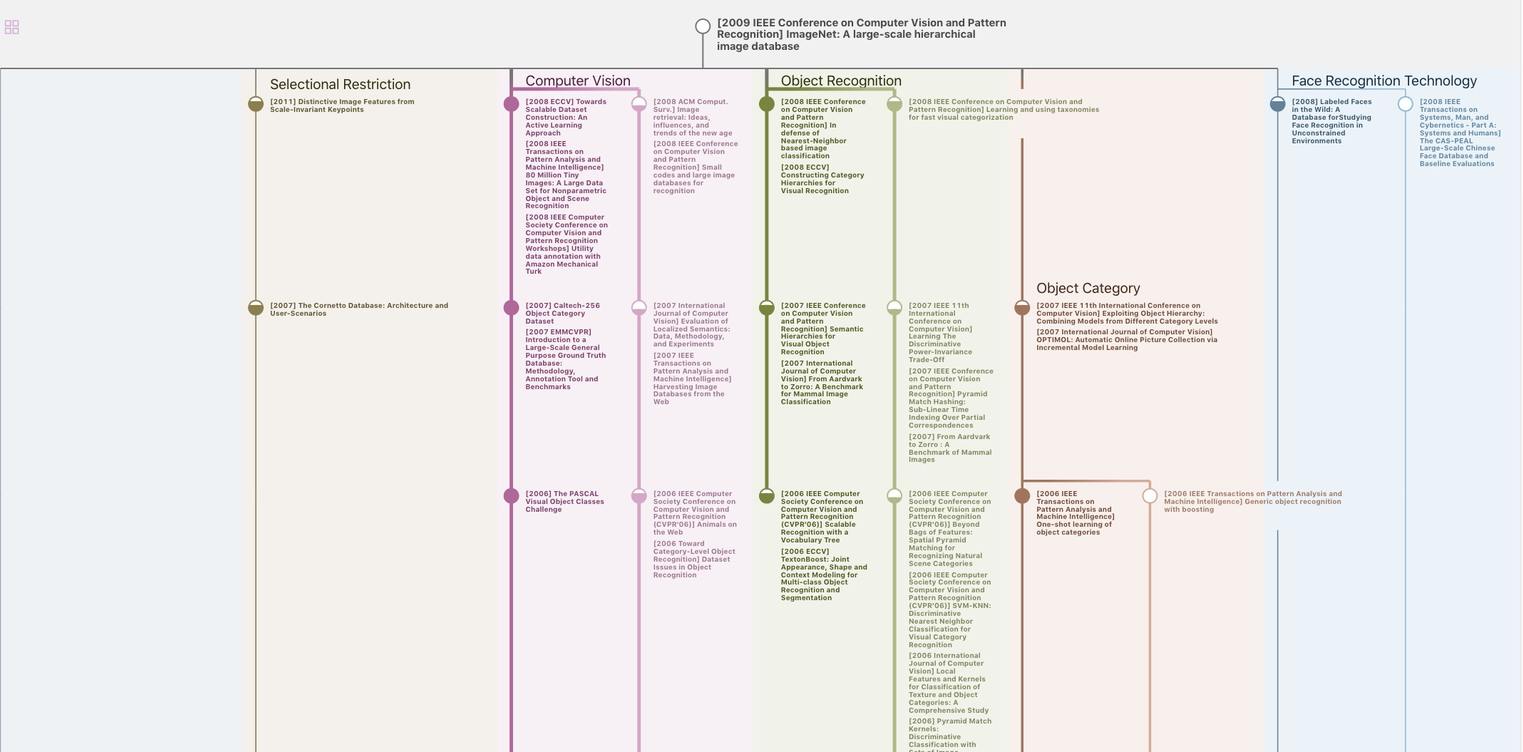
生成溯源树,研究论文发展脉络
Chat Paper
正在生成论文摘要