Provably Safe Reinforcement Learning: A Theoretical and Experimental Comparison
arxiv(2022)
摘要
Ensuring safety of reinforcement learning (RL) algorithms is crucial for many real-world tasks. However, vanilla RL does not guarantee safety for an agent. In recent years, several methods have been proposed to provide safety guarantees for RL. To the best of our knowledge, there is no comprehensive comparison of these provably safe RL methods. We therefore introduce a categorization for existing provably safe RL methods, and present the theoretical foundations for both continuous and discrete action spaces. Additionally, we evaluate provably safe RL on an inverted pendulum. In the experiments, it is shown that indeed only provably safe RL methods guarantee safety.
更多查看译文
关键词
safe,learning
AI 理解论文
溯源树
样例
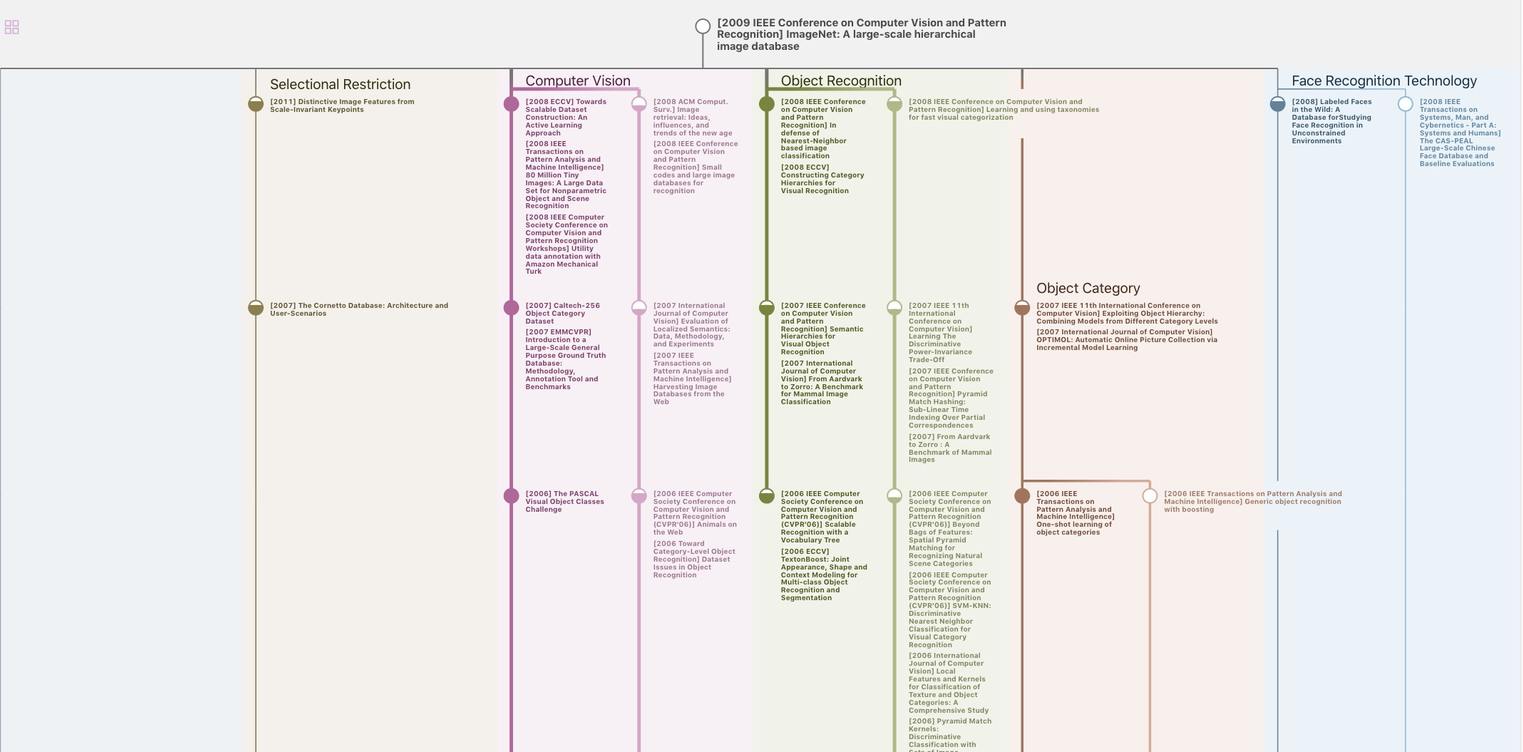
生成溯源树,研究论文发展脉络
Chat Paper
正在生成论文摘要