Knowledge Graph Question Answering Datasets and Their Generalizability: Are They Enough for Future Research?
SIGIR '22: Proceedings of the 45th International ACM SIGIR Conference on Research and Development in Information Retrieval(2022)
摘要
Existing approaches on Question Answering over Knowledge Graphs (KGQA) have weak generalizability. That is often due to the standard i.i.d. assumption on the underlying dataset. Recently, three levels of generalization for KGQA were defined, namely i.i.d., compositional, zero-shot. We analyze 25 well-known KGQA datasets for 5 different Knowledge Graphs (KGs). We show that according to this definition many existing and online available KGQA datasets are either not suited to train a generalizable KGQA system or that the datasets are based on discontinued and out-dated KGs. Generating new datasets is a costly process and, thus, is not an alternative to smaller research groups and companies. In this work, we propose a mitigation method for re-splitting available KGQA datasets to enable their applicability to evaluate generalization, without any cost and manual effort. We test our hypothesis on three KGQA datasets, i.e., LC-QuAD, LC-QuAD 2.0 and QALD-9). Experiments on re-splitted KGQA datasets demonstrate its effectiveness towards generalizability. The code and a unified way to access 18 available datasets is online at https://github.com/semantic-systems/KGQA-datasets as well as https://github.com/semantic-systems/KGQA-datasets-generalization.
更多查看译文
关键词
KGQA, Question Answering, Generalization, Generalizability, Benchmark, Evaluation
AI 理解论文
溯源树
样例
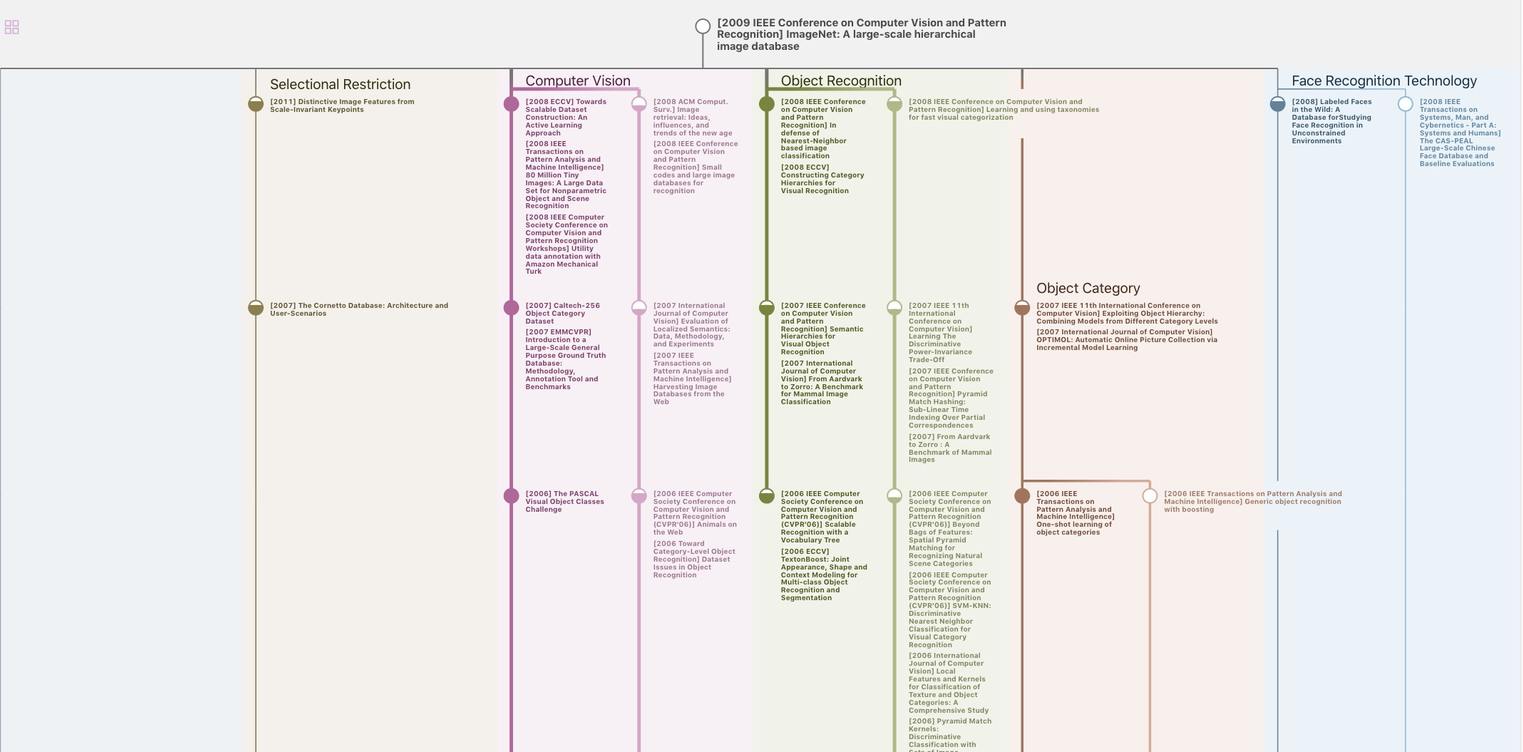
生成溯源树,研究论文发展脉络
Chat Paper
正在生成论文摘要