Data-Driven Estimation of Capacity Upper Bounds
IEEE Communications Letters(2022)
摘要
We consider the problem of estimating an upper bound on the capacity of a memoryless channel with unknown channel law and continuous output alphabet. A novel data-driven algorithm is proposed that exploits the dual representation of capacity where the maximization over the input distribution is replaced with a minimization over a reference distribution on the channel output. To efficiently compute the required divergence maximization between the conditional channel and the reference distribution, we use a modified mutual information neural estimator that takes the channel input as an additional parameter. We numerically evaluate our approach on different memoryless channels and show empirically that the estimated upper bounds closely converge either to the channel capacity or to best-known lower bounds.
更多查看译文
关键词
Autoencoders,channel capacity,divergence estimation,duality,dual capacity representation,mutual information neural estimation,neural networks,upper capacity bounds
AI 理解论文
溯源树
样例
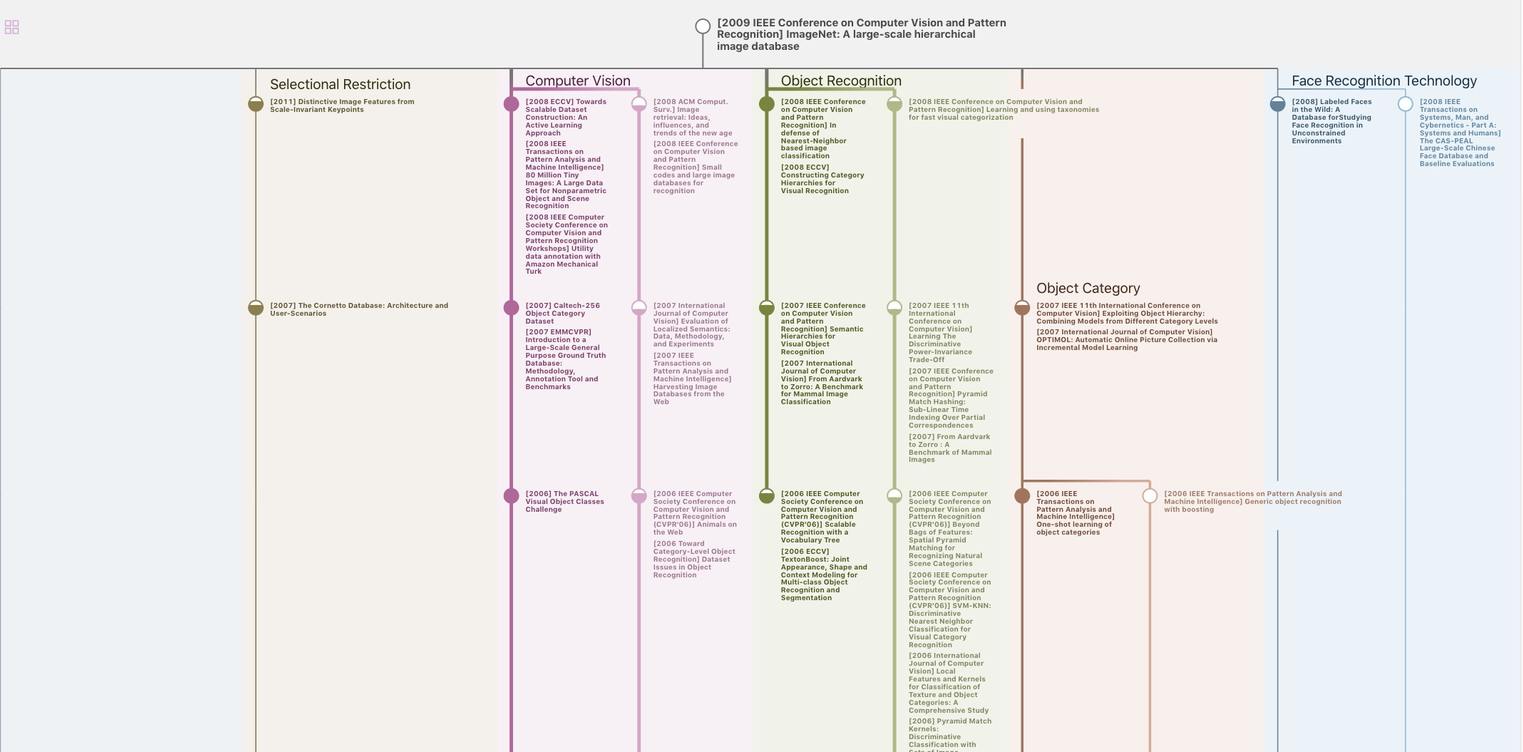
生成溯源树,研究论文发展脉络
Chat Paper
正在生成论文摘要