ripple 2 vec : Node Embedding with Ripple Distance of Structures
Data Science and Engineering(2022)
摘要
Graph is a generic model of various networks in real-world applications. And, graph embedding aims to represent nodes (edges or graphs) as low-dimensional vectors which can be fed into machine learning algorithms for downstream graph analysis tasks. However, existing random walk-based node embedding methods often map some nodes with (dis)similar local structures to (near) far vectors. To overcome this issue, this paper proposes to implement node embedding by constructing a context graph via a new defined ripple distance over ripple vectors, whose components are the hitting times of fully condensed neighborhoods and thus characterize their structures as pure quantities. The distance is able to capture the (dis)similarities of nodes’ local neighborhood structures and satisfies the triangular inequality. The neighbors of each node in the context graph are defined via the ripple distance, which makes the short random walks from a given node over the context graph only visit its similar nodes in the original graph. This property guarantees that the proposed method, named as 𝗋𝗂𝗉𝗉𝗅𝖾2𝗏𝖾𝖼 , is able to map (dis)similar nodes to (far) near vectors. Experimental results on real datasets, where labels are mainly related to nodes’ local structures, show that the results of 𝗋𝗂𝗉𝗉𝗅𝖾2𝗏𝖾𝖼 behave better than those of state-of-the-art methods, in node clustering and node classification, and are competitive to other methods in link prediction.
更多查看译文
关键词
Node embedding, Structural similarity, Ripple distance
AI 理解论文
溯源树
样例
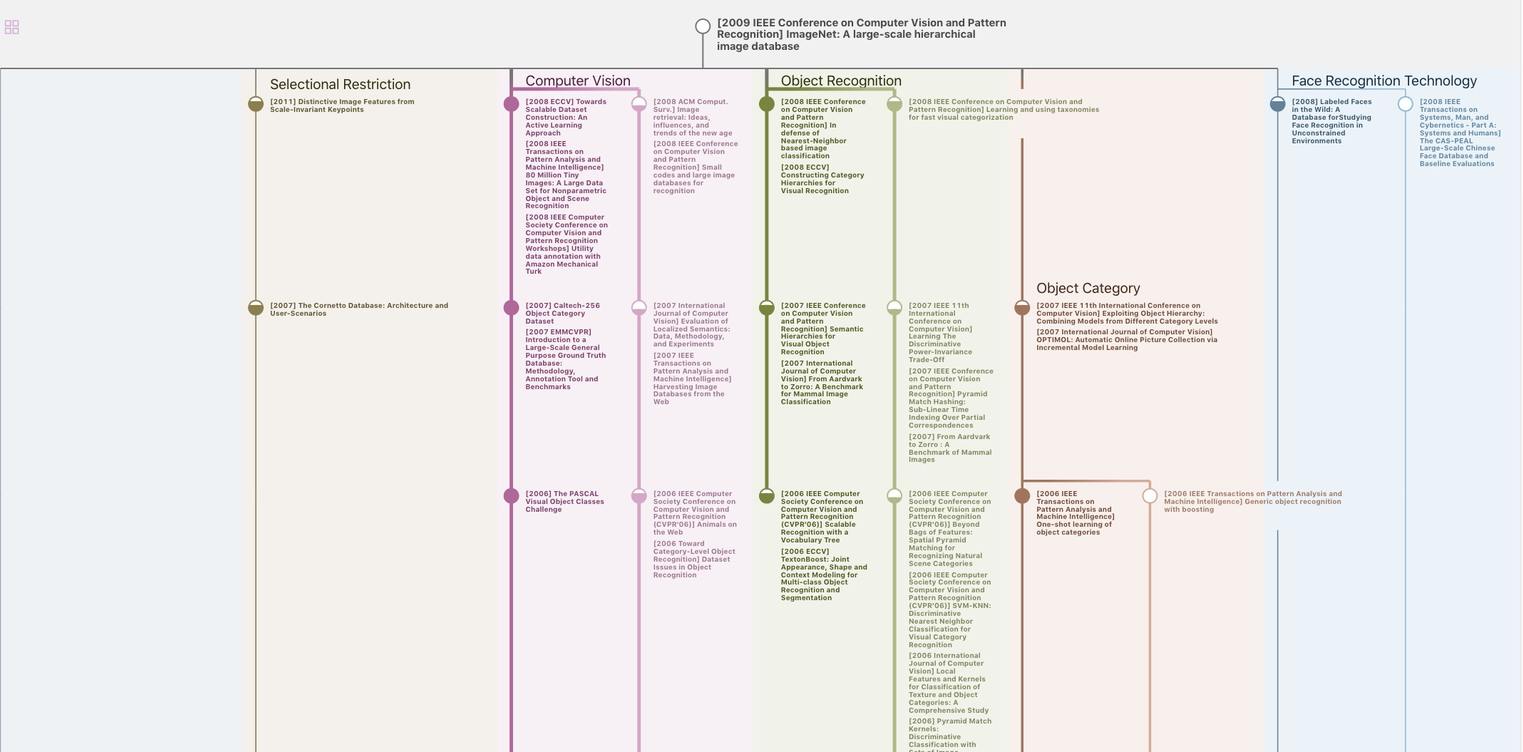
生成溯源树,研究论文发展脉络
Chat Paper
正在生成论文摘要