Understanding frailty: probabilistic causality between components and their relationship with death through a Bayesian network and evidence propagation
medRxiv(2022)
摘要
Identifying relationships between components of an index helps to have a better understanding of the condition they define. The Frailty Index (FI) measures the global health of individuals and can be used to predict outcomes as mortality. Previously, we modelled the relationship between the FI components (deficits) and death through an undirected graphical model, analyzing their relevance from a social network analysis framework. Here, we model the FI components and death through an averaged Bayesian network obtained through a structural learning process and resampling, to understand how the FI components and death are causally related. We identified that the components are not similarly related between them and that deficits are related according to its type. Two deficits were the most relevant in terms of their connections and other two were directly associated with death. We also obtained the strength of the relationships to identify the most plausible, identifying clusters of deficits heavily related. Finally, we propagated evidence (assigned values to all deficits) and studied how FI components predict mortality, obtaining a correct assignation of almost 74%, whereas a sensitivity of 56%. As a classifier of death, the more number of deficits included for the evidence, the best performance; but the FI seems not to be very good to correctly classify death people.
更多查看译文
关键词
Bayesian networks, evidence propagation, classification, aging, frailty index, mortality
AI 理解论文
溯源树
样例
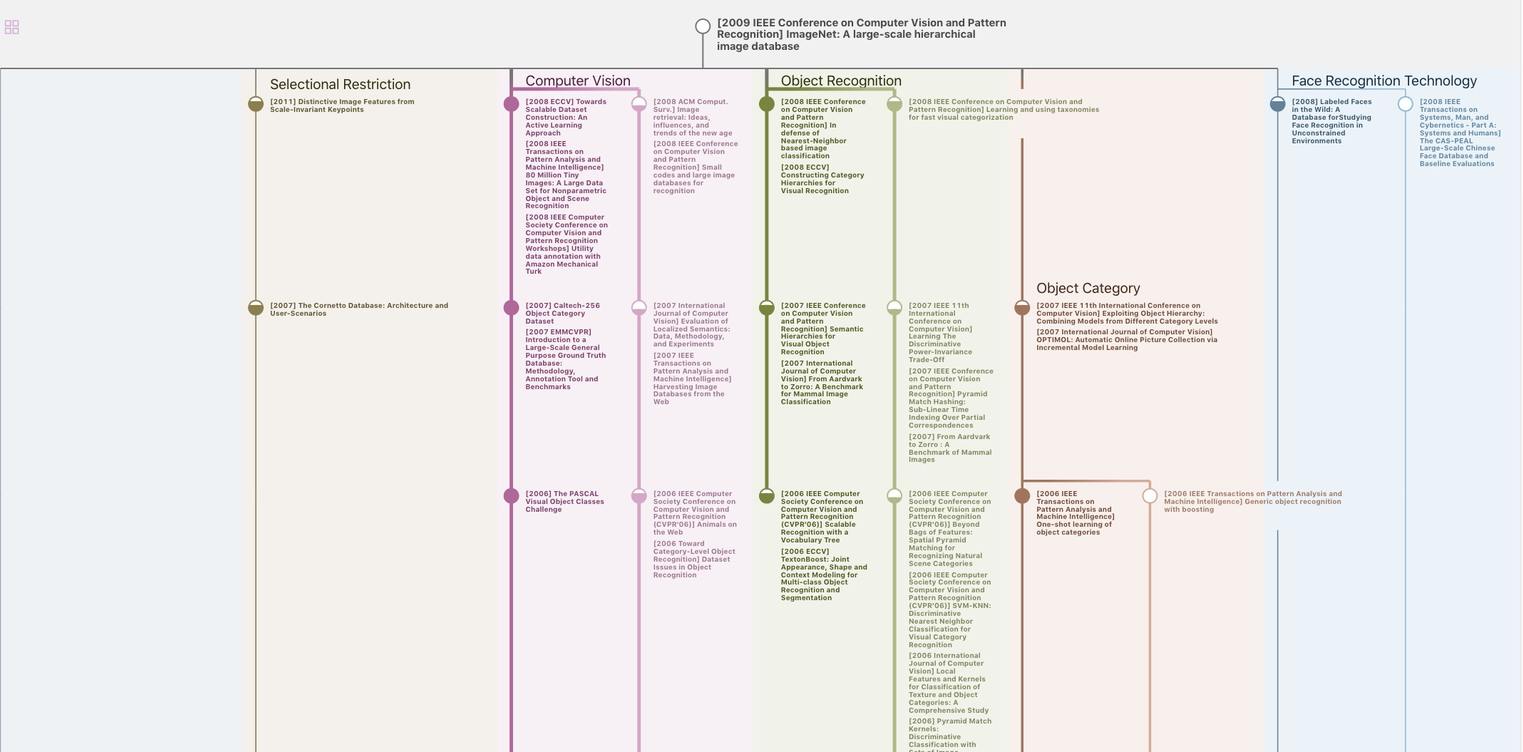
生成溯源树,研究论文发展脉络
Chat Paper
正在生成论文摘要