Multi-Modal Brain and Ventricle Segmentation Using Weakly Supervised Transfer Learning
semanticscholar(2021)
摘要
Purpose : To quantify performance of brain and ventricle segmentation using weakly supervised Transfer Learning (TL) and cross-modality CT-to-MR deep learning models trained with coarse-grained versus fine-grained data to en able accurate segmentation using small datasets. Methods : An IRB approved, retrospective study using MR and CT images was performed. Three datasets consist ing of roughly 2500 total images with coarse or fine annota tions (labels) were used for training, validation and testing of Convolution Neural Networks (CNNs) models. The best CNN architecture was used to investigate TL performance on segmentation, influence of training set size for TL accu racy and effectiveness of cross-modality TL. Dice Score (DS) and Percent Volume Difference (PVD) were used to quantify segmentation accuracy. Two-sided Wilcoxon signed rank with p<0.05 indicated statistical significance. Results : Deeper, wider models outperformed other ar chitectures for segmentation tasks. Ventricle segmentation models trained with fine-grained data improved DS from 0.75 to 0.82 and PVD from 21.5% to 8.02% over coarse-grained models, both statistically significant. DS and PVD improved when using TL over noTL (0.86 vs. 0.83, p<0.01 and 6.87% vs. 8.02%, p=0.80, respectively). Both MR-to-MR and cross-modality MR-to-CT TL models trained with as few as 20 images showed similar results to models trained with 100 images and vastly outperformed small training size de novo models. Additionally, the cross-modality TL showed statistically significant improved results over noTL models and slightly lower DS and PVD than within-modality models. Conclusion : Brain and ventricle segmentation using deep and wide CNN networks outperformed shallower CNN models. Within-modality and cross-modality TL models achieved similar or superior performance compared to noTL models and TL models showed these results when trained with as little at 20% of the data of noTL models.
更多查看译文
AI 理解论文
溯源树
样例
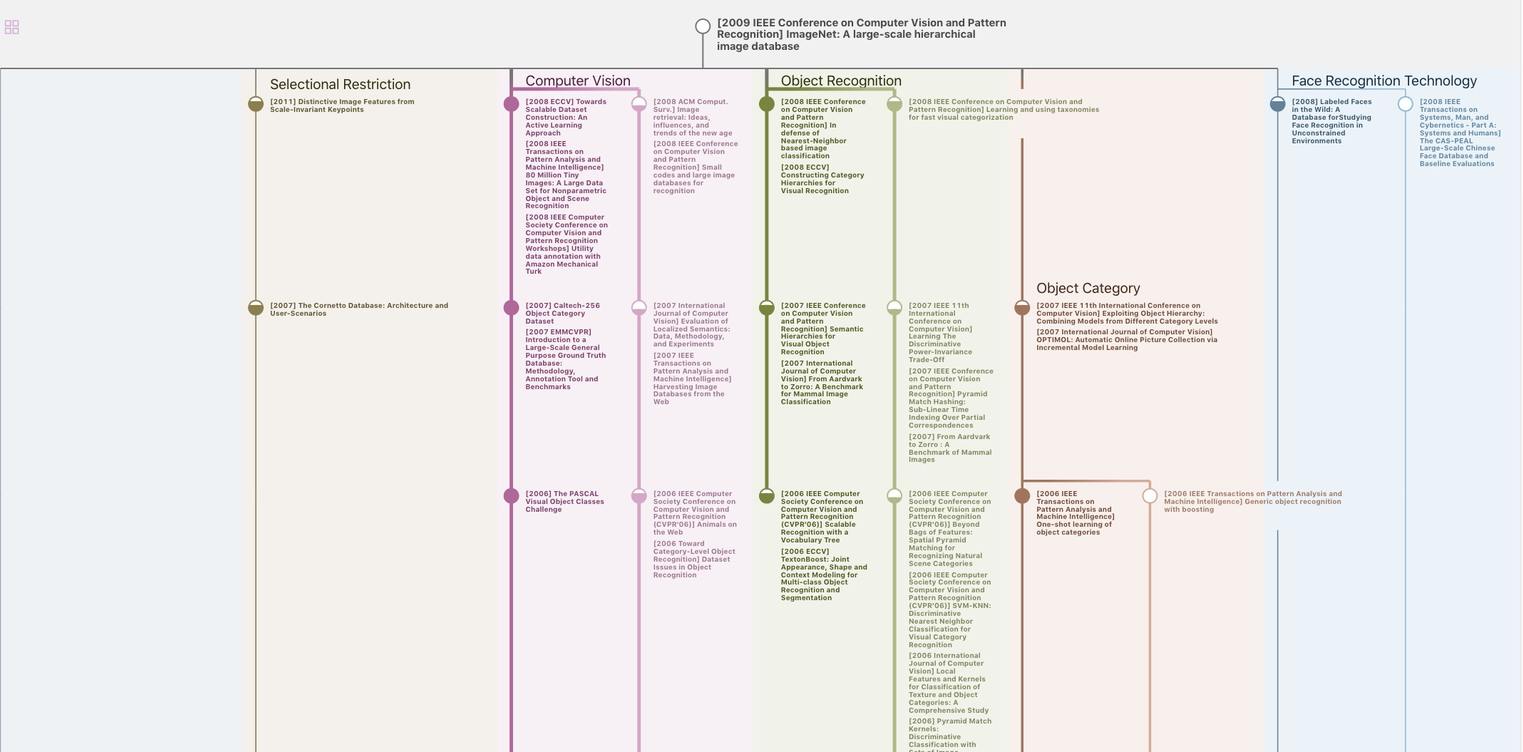
生成溯源树,研究论文发展脉络
Chat Paper
正在生成论文摘要