Medium Transmission Map Matters for Learning to Restore Real-World Underwater Images.
CoRR(2022)
摘要
Underwater visual perception is essentially important for underwater exploration, archeology, ecosystem and so on. The low illumination, light reflections, scattering, absorption and suspended particles inevitably lead to the critically degraded underwater image quality, which causes great challenges on recognizing the objects from the underwater images. The existing underwater enhancement methods that aim to promote the underwater visibility, heavily suffer from the poor image restoration performance and generalization ability. To reduce the difficulty of underwater image enhancement, we introduce the media transmission map as guidance to assist in image enhancement. We formulate the interaction between the underwater visual images and the transmission map to obtain better enhancement results. Even with simple and lightweight network configuration, the proposed method can achieve ad-vanced results of 22.6 dB on the challenging Test-R90 with an impressive 30 times faster than the existing models. Com-prehensive experimental results have demonstrated the superiority and potential on underwater perception. Paper’s code is offered on: https://github.com/GroupG-yk/MTUR-Net.
更多查看译文
AI 理解论文
溯源树
样例
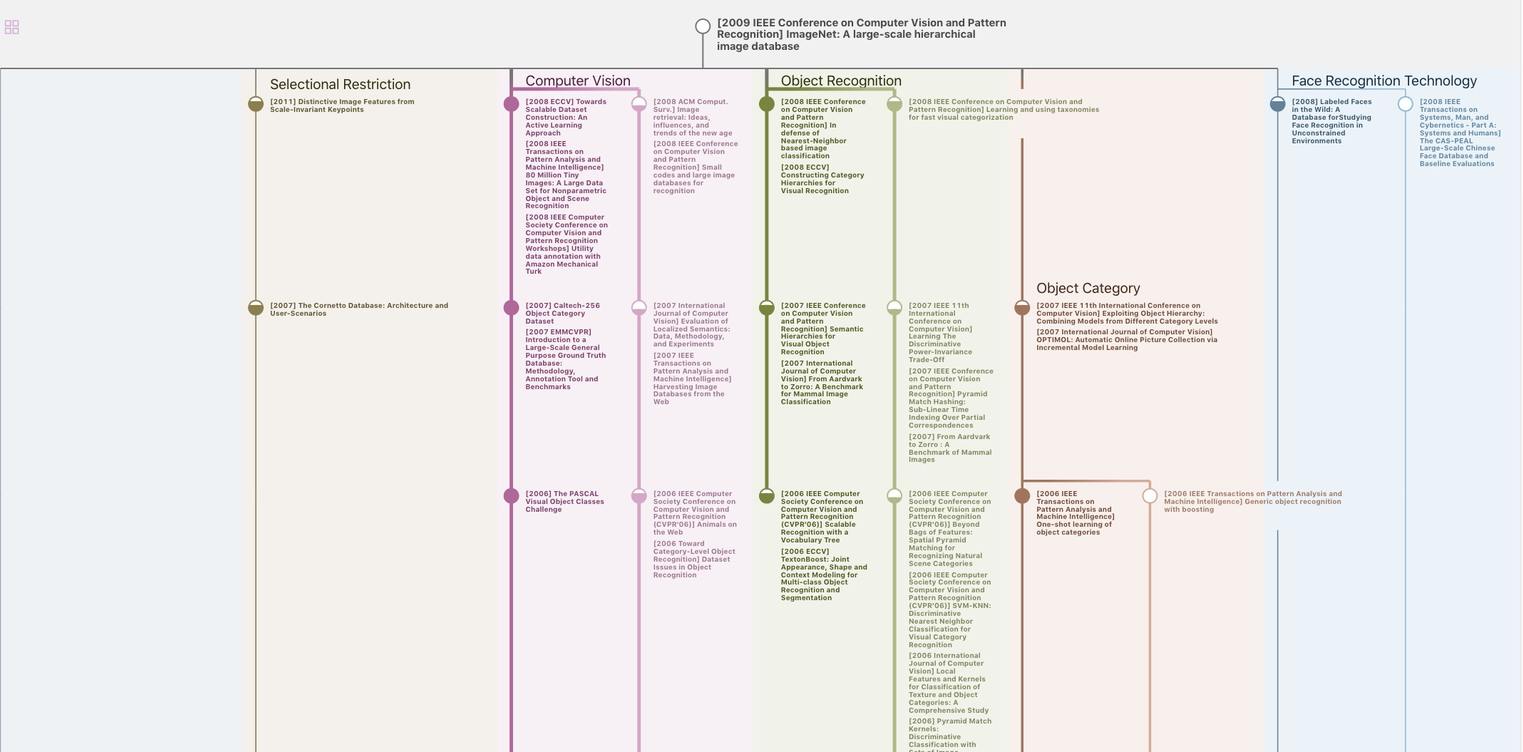
生成溯源树,研究论文发展脉络
Chat Paper
正在生成论文摘要