Fast Finite Width Neural Tangent Kernel
International Conference on Machine Learning(2022)
摘要
bians, Θθ(x1, x2) = [ ∂f(θ, x1) / ∂θ ] [ ∂f(θ, x2) / ∂θ ]T , has emerged as a central object of study in deep learning. However, it is notoriously expensive to compute, severely limiting its practical utility. We perform the first in-depth analysis of the compute and memory requirements for NTK computation in finite NNs. Leveraging their structure, we propose two novel algorithms that change the exponent of the compute and memory requirements of the finite width NTK, dramatically improving efficiency in a wide range of NN architectures on all hardware platforms. We open-source [github.com/iclr2022anon/fast finite width ntk] our two algorithms as general-purpose JAX function transformations that apply to any differentiable computation and introduce no hyperparameters.
更多查看译文
AI 理解论文
溯源树
样例
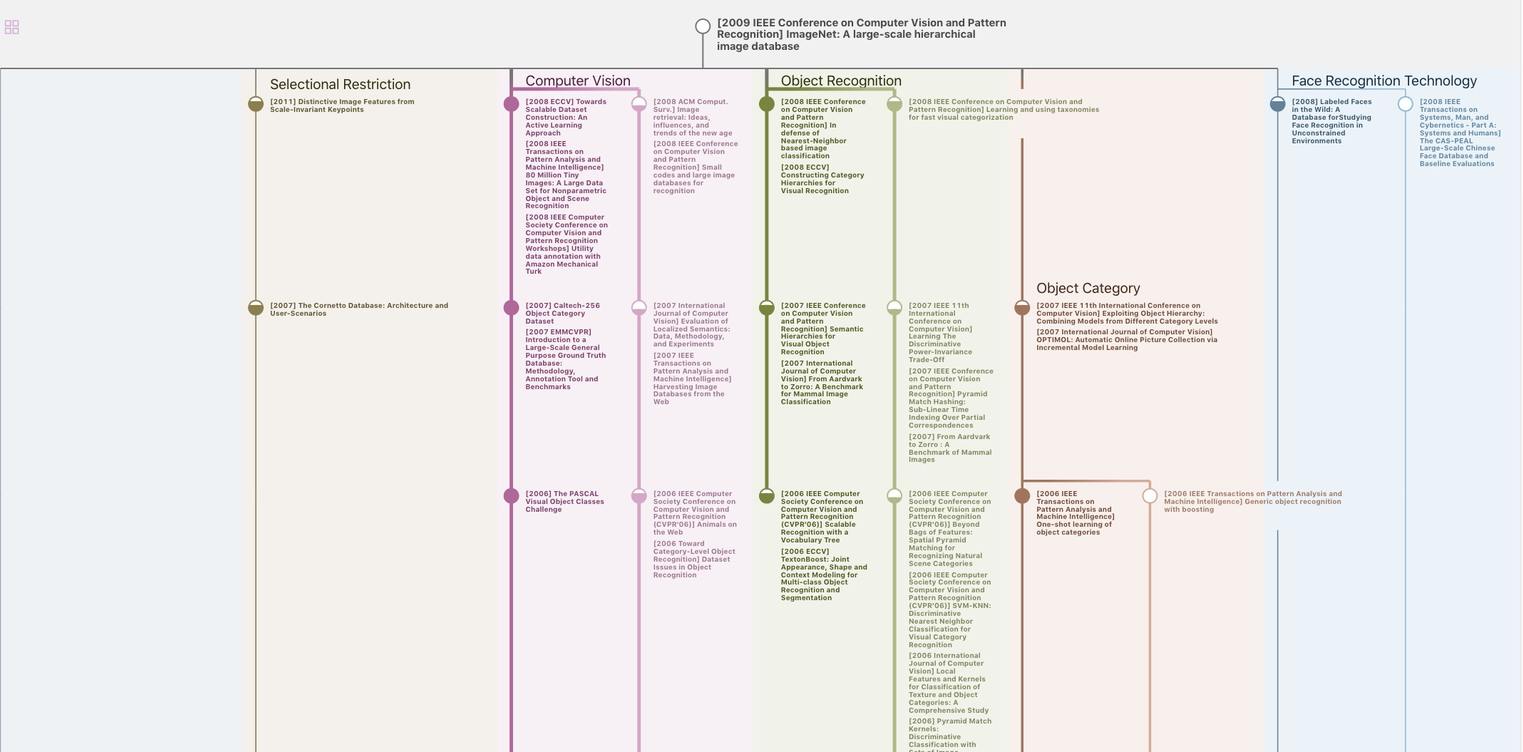
生成溯源树,研究论文发展脉络
Chat Paper
正在生成论文摘要