Power output optimization of electric vehicles smart charging hubs using deep reinforcement learning
Expert Systems with Applications(2022)
摘要
Since most branches of the distribution grid may already be close to their maximum capacity, smart management when charging electric vehicles (EVs) is becoming more and more crucial. In fact, office buildings might not be able to handle several transactions at the same time, especially considering the next generation of fast chargers which are very power expensive. Thus, an efficient charging policy needs to be found. This paper proposes the scheduling of real-time EVs charging through deep reinforcement learning (DRL) techniques. DRL has been chosen because it can adaptively learn from interacting with the surrounding environment. The focus of the optimization is to ensure the completion of the charging transactions in a timely manner, while shifting the load from the times of peak demand. The novelty of the proposed approach lies in its innovative framework: pools of electric vehicles with different characteristics are categorized using a clustering algorithm, a tree-based classifier has been developed to sort new instances of EVs, and a multilayer perceptron artificial deep neural network has been trained to predict the expected duration of each charging session. These features are used as inputs to the DRL agent, and are mapped into actions that adjust the maximum power associated to each charging station. The model has been compared to a traditional charging algorithm and increasingly challenging scenarios have been considered. Results have shown that the developed algorithm fails less than the baseline, with a reduction of the load due to EVs charging of 80% during peak times.
更多查看译文
关键词
Reinforcement learning,Electric vehicles,Real-time charging scheduling,Neural network,Clustering algorithm
AI 理解论文
溯源树
样例
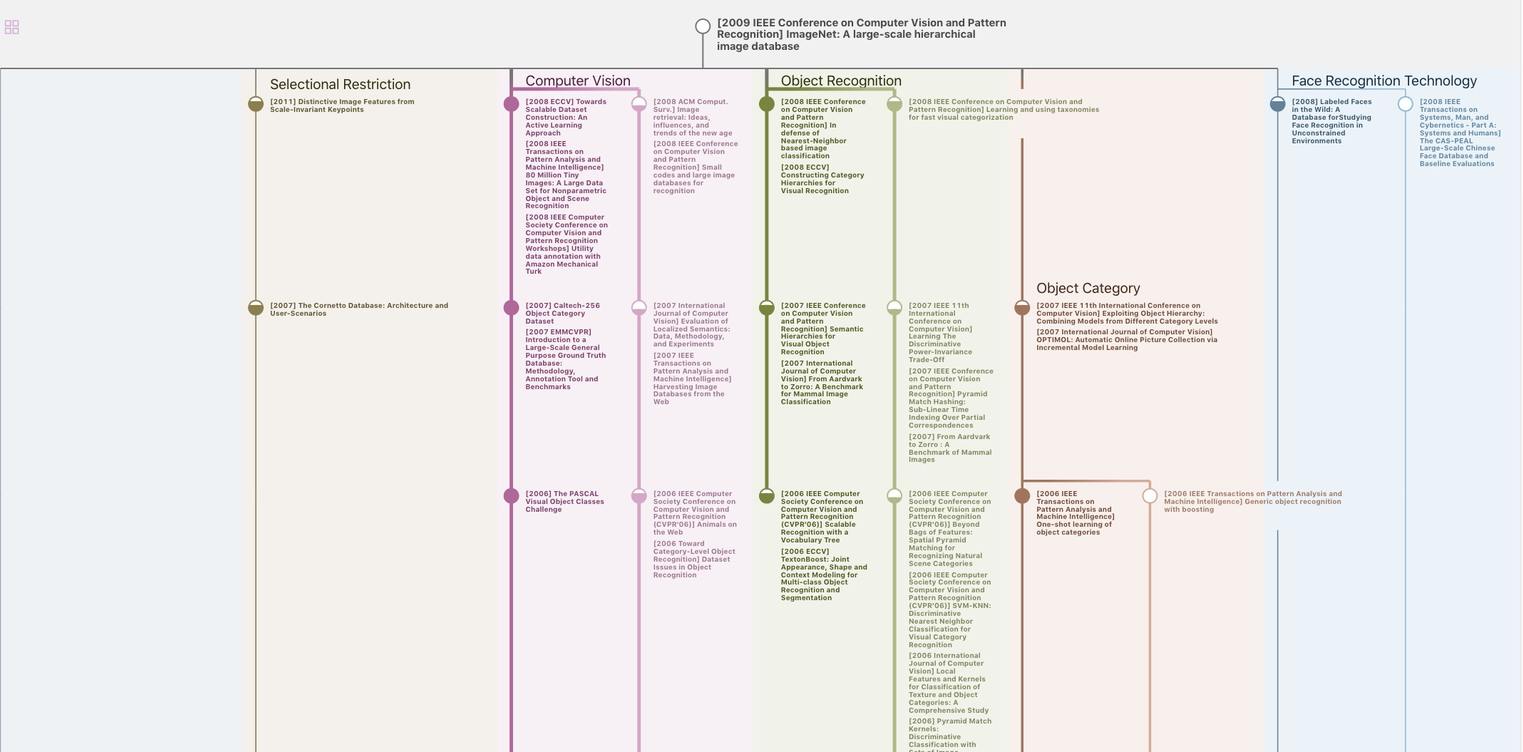
生成溯源树,研究论文发展脉络
Chat Paper
正在生成论文摘要