Dual Distance Optimized Deep Quantization With Semantics-Preserving
IEEE SIGNAL PROCESSING LETTERS(2022)
摘要
Recently, quantization has been an effective technique for large-scale image retrieval, which can encode feature vectors into compact codes. However, it is still a great challenge to improve the discriminative capability of codewords while minimizing the quantization error. This letter proposes Dual Distance Optimized Deep Quantization (D(2)ODQ) to deal with this issue, by minimizing the Euclidean distance between samples and codewords, and maximizing the minimum cosine distance between codewords. To generate the evenly distributed codebook, we find the general solution for the upper bound of the minimum cosine distance between codewords. Moreover, scaler constrained semantics-preserving loss is considered to avoid trivial quantization boundary, and ensure that a codeword can only quantize the features of one category. In contrast to state-of-the-art methods, our method has a better performance on three benchmark datasets.
更多查看译文
关键词
Quantization (signal),Upper bound,Training,Semantics,Image retrieval,Euclidean distance,Binary codes,Deep learning,image retrieval,quantization
AI 理解论文
溯源树
样例
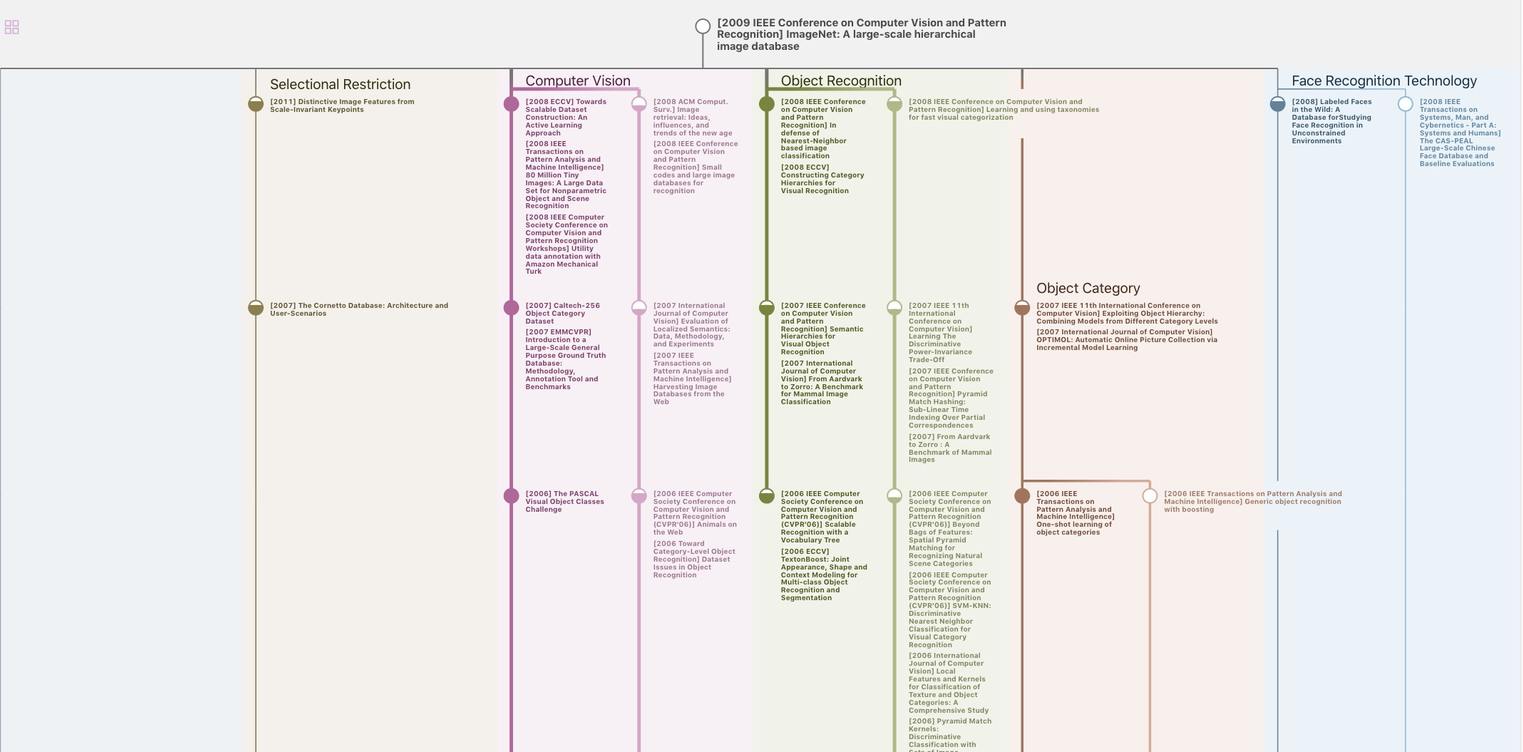
生成溯源树,研究论文发展脉络
Chat Paper
正在生成论文摘要