A Study of Explainable Community-Level Features
semanticscholar(2021)
摘要
Community finding algorithms are complex, stochastic algorithms used to find highly connected groups of individuals in a graph. As with “black-box” machine learning approaches, these algorithms provide little explanation or insight into their outputs. In this novel research paper, inspired by work in explainable artificial intelligence (XAI), we look to develop post-hoc explanations for community finding algorithms. Specifically, we aim to identify features that indicate whether a set of nodes comprises a community or not. We evaluate our model-agnostic methodology, which selects interpretable features from a longlist of candidates, in the context of three well-known community finding algorithms.
更多查看译文
AI 理解论文
溯源树
样例
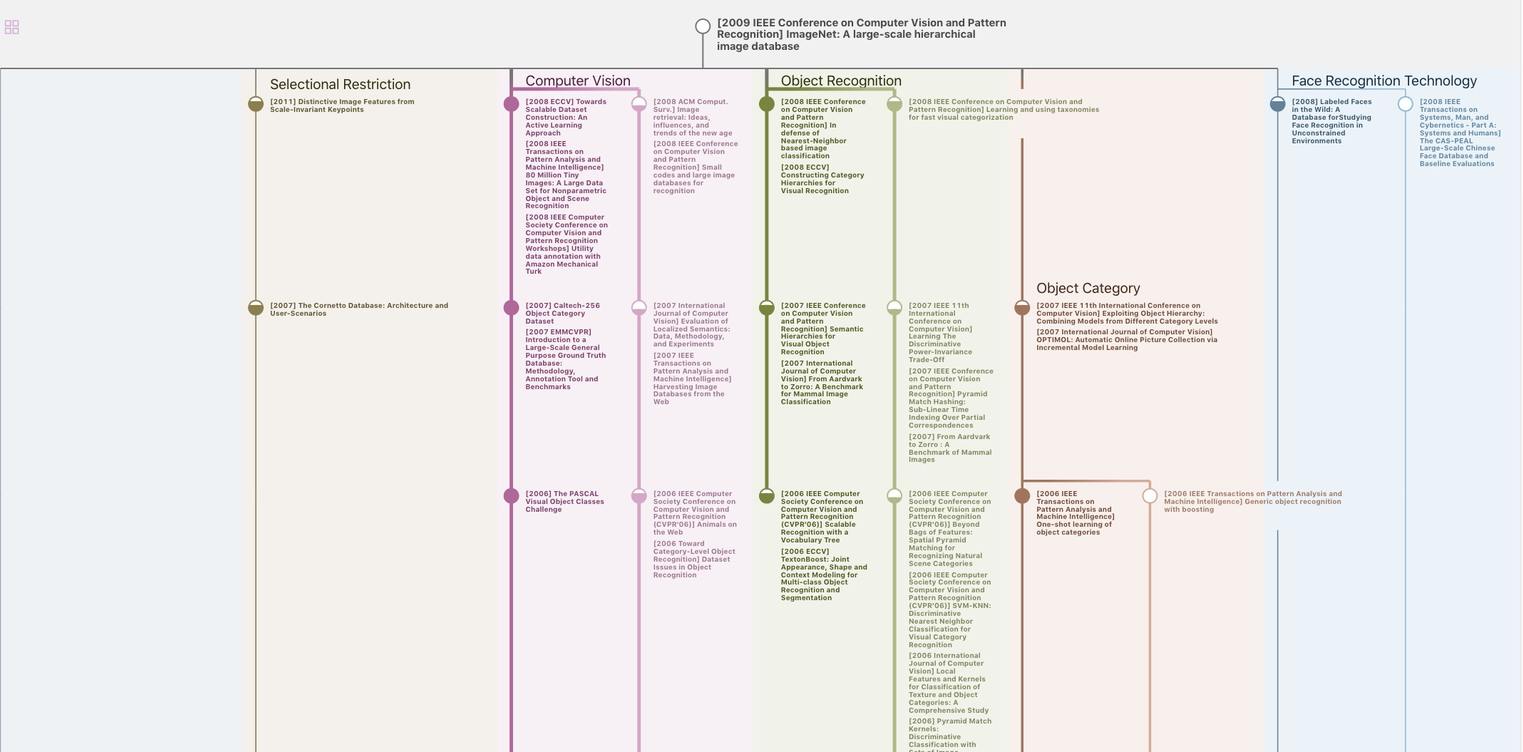
生成溯源树,研究论文发展脉络
Chat Paper
正在生成论文摘要