Extracting Creativity from Narratives using Distributional Semantic Modeling
semanticscholar(2021)
摘要
Narrative text permeates our lives from job applications to journalistic stories to works of fiction. Developing automated metrics that capture creativity in narrative text has potentially far reaching implications. Human ratings of creativity in narrative text are labor-intensive, subjective, and difficult to replicate. Across 27 different story prompts and over 3,500 short stories, we used distributional semantic modeling to automate the assessment of creativity in narrative texts. We tested a new metric to capture one key component of creativity in writing – a writer’s ability to connect divergent ideas. We termed this metric, word-to-word semantic diversity (w2w SemDiv). We compared six models of w2w SemDiv that varied in their computational architecture. The best performing model employed Bidirectional Encoder Representations Transformer (BERT), which generates context-dependent numerical representations of words (i.e., embeddings). The BERT w2w SemDiv scores demonstrated impressive predictive power, explaining up to 72% of the variance in human creativity ratings, even exceeding human inter-rater reliability for some tasks. In addition, w2w SemDiv scores generalized across Ethnicity and English language proficiency, including individuals identifying as Hispanic and L2 English speakers. We provide a tutorial with R code (osf.io/ath2s) on how to compute w2w SemDiv. This code is incorporated into an online web app (semdis.wlu.psu.edu) where researchers and educators can upload a data file with stories and freely retrieve w2w SemDiv scores.
更多查看译文
AI 理解论文
溯源树
样例
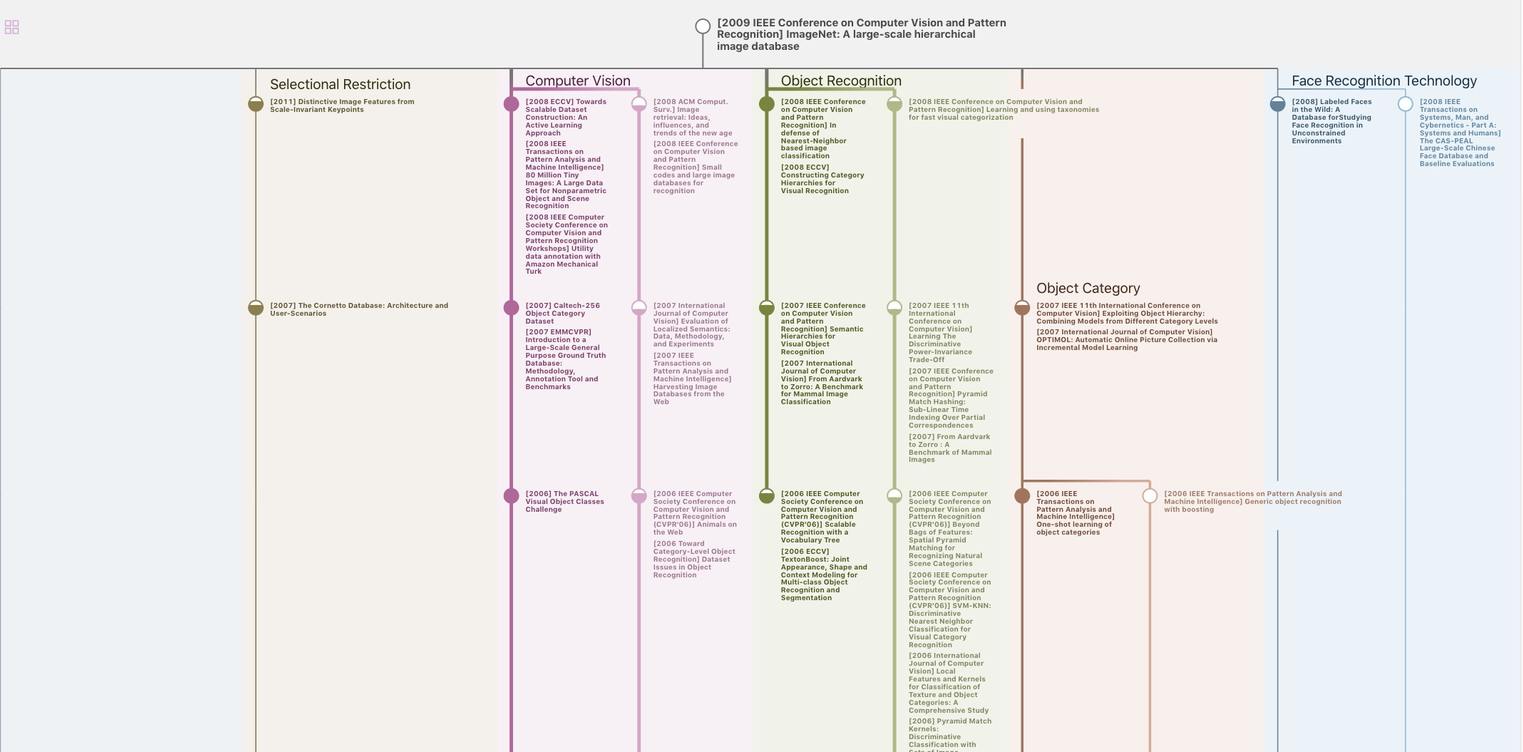
生成溯源树,研究论文发展脉络
Chat Paper
正在生成论文摘要