An Entropy-Weighted Network for Polar Sea Ice Open Lead Detection From Sentinel-1 SAR Images
IEEE TRANSACTIONS ON GEOSCIENCE AND REMOTE SENSING(2022)
摘要
Sea ice leads in the Arctic Ocean and Antarctic Ocean are of great significance to polar ecology, climate change, and ship navigation. While rule-based remote-sensing classification methods, such as the threshold method, have been widely used for surveying and mapping polar sea ice leads, they have difficulty in overcoming the problems of noise and poor generalization. In this article, we presented an automated, deep learning sea ice open lead detection network in low wind speed conditions, entropy-weighted network (EW-Net). In EW-Net, a dense block was introduced into a U-Net baseline network to strengthen feature propagation, and an entropy sampling structure was designed to improve the pertinence of the network to leads and alleviate the influence of noise. Furthermore, an entropy-weighted feature fusion block was designed to better restore features. EW-Net was trained on the Sentinel-1 synthetic aperture radar (SAR) dataset in order to obtain lead maps of different polar regions from Sentinel-1 images. The results showed that the proposed EW-Net model was more effective and more generalizable for polar sea ice lead detection than the threshold method and the deep learning methods U-Net and DeepLab V3 Plus, with an overall accuracy (OA) exceeding 0.97. In addition, EW-Net showed advantages in terms of outstanding generalization from cross-sensor image monitoring of leads. The method proposed in this article could be beneficial for providing accurate maps of polar sea ice leads in open water and contribute to further research on polar science.
更多查看译文
关键词
Sea ice,Radar polarimetry,Lead,Synthetic aperture radar,Entropy,Remote sensing,Deep learning,Antarctic Ocean,Arctic Ocean,deep learning,entropy,sea ice lead,synthetic aperture radar (SAR)
AI 理解论文
溯源树
样例
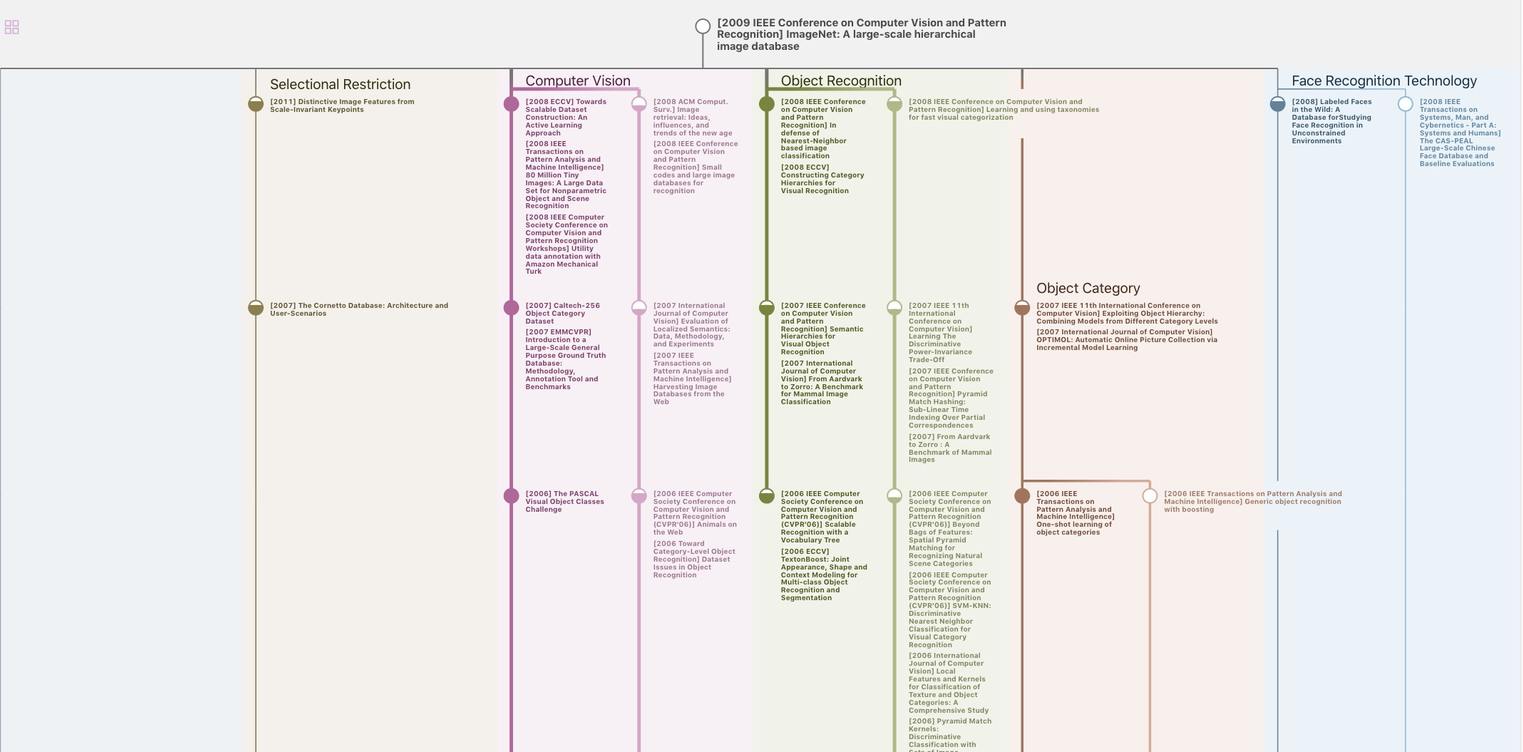
生成溯源树,研究论文发展脉络
Chat Paper
正在生成论文摘要