A Multi-strategy-mode-waterlogging-prediction Framework for Urban Flood Depth
semanticscholar(2022)
摘要
Abstract. Flood is one of the most disruptive natural hazards, leading to massive loss of lives and considerable damage to properties. Coastal cities in Asia face floods almost every year due to monsoons influences. Early notification of flood incidents benefits the authorities and public to devise both short and long terms preventive measures, prepare evacuation and rescue missions, and relieve the flood victims. Based on time series prediction and machine learning regression algorithm, an innovative multi-strategy-mode-waterlogging-prediction framework for predicting waterlogging depth is proposed in this paper. The framework combines the historical rainfall and waterlogging depth to predict the near future waterlogging in time under future weather conditions. An expanding rainfall model was proposed to consider the positive correlation of future rainfall on the waterlogging. By selecting a suitable prediction strategy, adjusting the optimal model parameters, and then comparing the different algorithms, the optimal configuration of prediction is selected. In the actual value testing, the selected model has high computational efficiency, and the accuracy of predicting the waterlogging depth after 30 minutes can reach 86.1 %, which is superior to many data-driven prediction models for waterlogging depth. The framework is helpful to timely predict the depth of target point with a high level of accuracy. It’s of great significance to timely release early warning information to avoid casualties and property losses.
更多查看译文
AI 理解论文
溯源树
样例
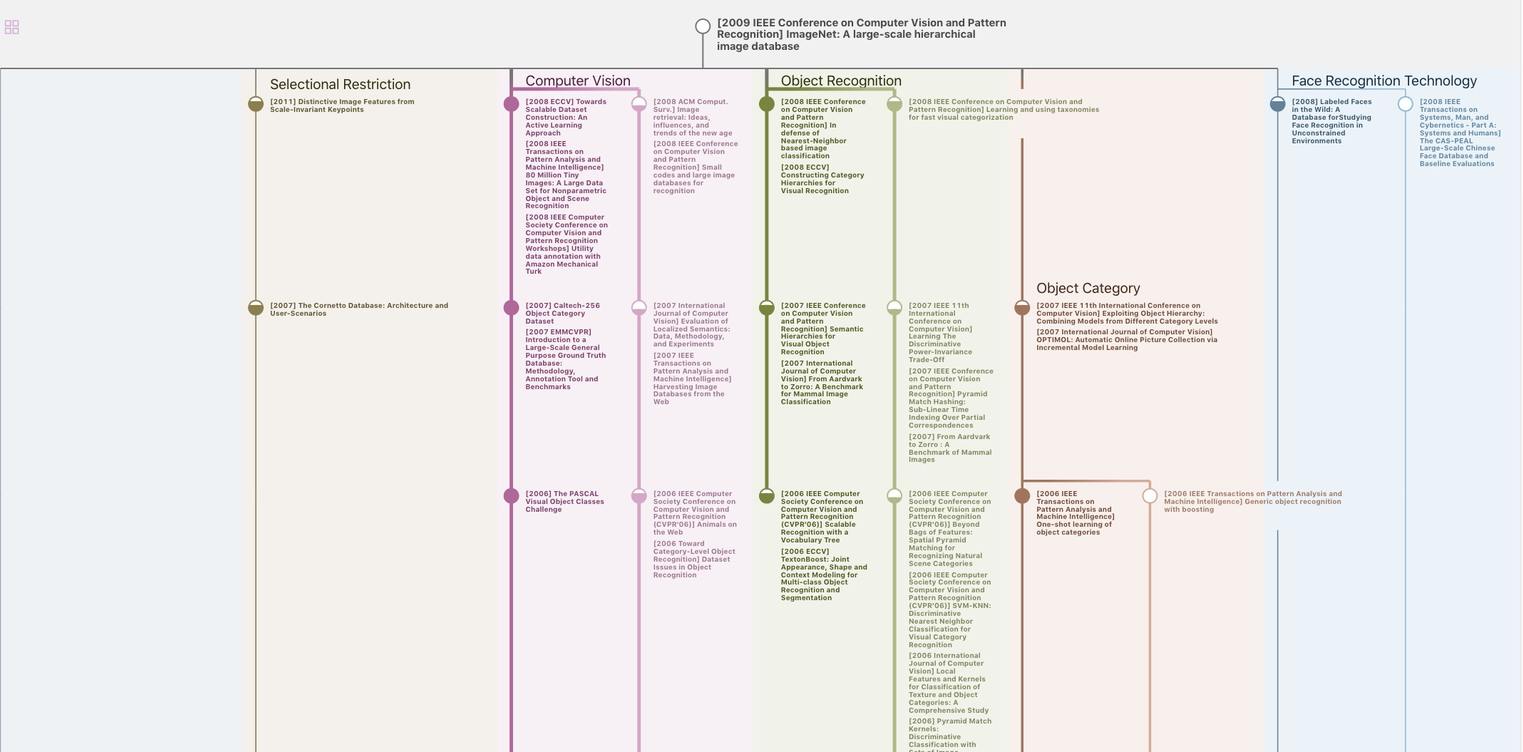
生成溯源树,研究论文发展脉络
Chat Paper
正在生成论文摘要