Learning Competitive Equilibria in Exchange Economies with Bandit Feedback
International Conference on Artificial Intelligence and Statistics (AISTATS)(2022)
摘要
The sharing of scarce resources among multiple rational agents is one of the classical problems in economics. In exchange economies, which are used to model such situations, agents begin with an initial endowment of resources and exchange them in a way that is mutually beneficial until they reach a competitive equilibrium (CE). The allocations at a CE are Pareto efficient and fair. Consequently, they are used widely in designing mechanisms for fair division. However, computing CEs requires the knowledge of agent preferences which are unknown in several applications of interest. In this work, we explore a new online learning mechanism, which, on each round, allocates resources to the agents and collects stochastic feedback on their experience in using that allocation. Its goal is to learn the agent utilities via this feedback and imitate the allocations at a CE in the long run. We quantify CE behavior via two losses and propose a randomized algorithm which achieves sublinear loss under a parametric class of utilities. Empirically, we demonstrate the effectiveness of this mechanism through numerical simulations.
更多查看译文
关键词
competitive equilibria,bandit
AI 理解论文
溯源树
样例
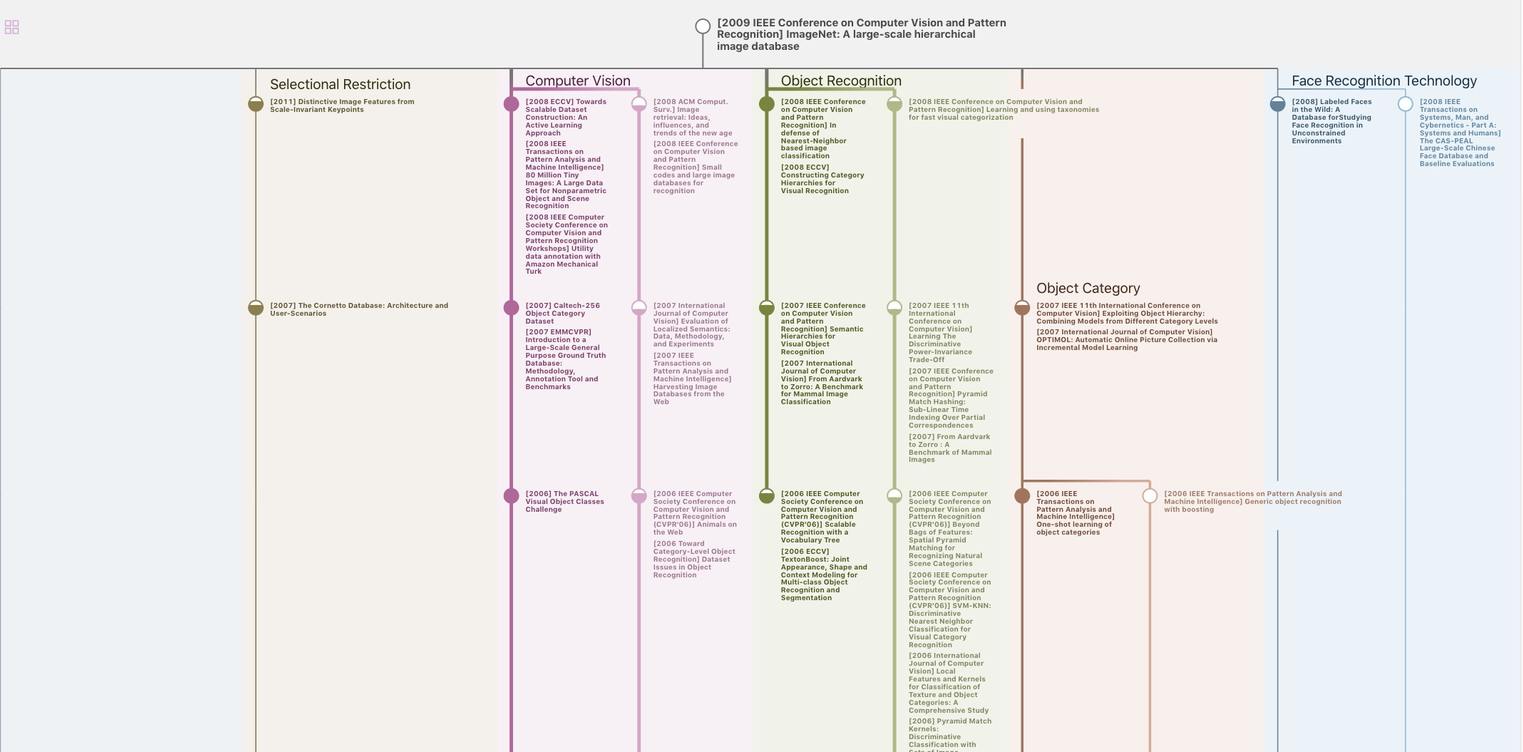
生成溯源树,研究论文发展脉络
Chat Paper
正在生成论文摘要