Federated Machine Learning For Augmenting The Safekeeping of Critical Energy Infrastructures
Research Square (Research Square)(2021)
摘要
In the recent years, there have been an increase in attacks targeting Supervisory Control and Data Acquisition (SCADA) infrastructures as there are many sensitive data released from peripheral devices. Wind-turbine systems are considered to be the most complex Cyber-Physical infrastructures.A privacy preserving Federated Machine Learning solution is proposed in order detect any possible anomalies in such infrastructures. Instead of centralizing the wind-turbine data into a common server, Federated Machine Learning allows the data to remain on-premise in the infrastructure. This enables the responsible authorities to consider the advantages of Machine Learning, and simultaneously protect their privacy. Different federated machine learning models namely Support Vector Machine (SVM), K-Nearest Neighbor (KNN), Radial Basis Function (RBF), Multi-Layer Perceptron (MLP) and Random Forest (RF) are deployed to analyze the anomalies in the wind turbines. It is inferred from the experimental results that Random Forest is superior in identifying the anomalies with regard to the performance metrics such asMean Absolute Error (MAE), Root Mean Square Error (RMSE) and Mean Square Error (MSE) are minimal for Random Forest in comparison to other models and Coefficient of Determination (R 2 ) is higher which is expected for a model that accurately predicts the anomalies. In addition, the time taken by the regression is 42.95 seconds which is minimal in comparison to other classifiers. Thus, a federated Random Forest Learner accurately analyzes the anomalies in critical wind turbine infrastructures.
更多查看译文
关键词
machine learning,energy
AI 理解论文
溯源树
样例
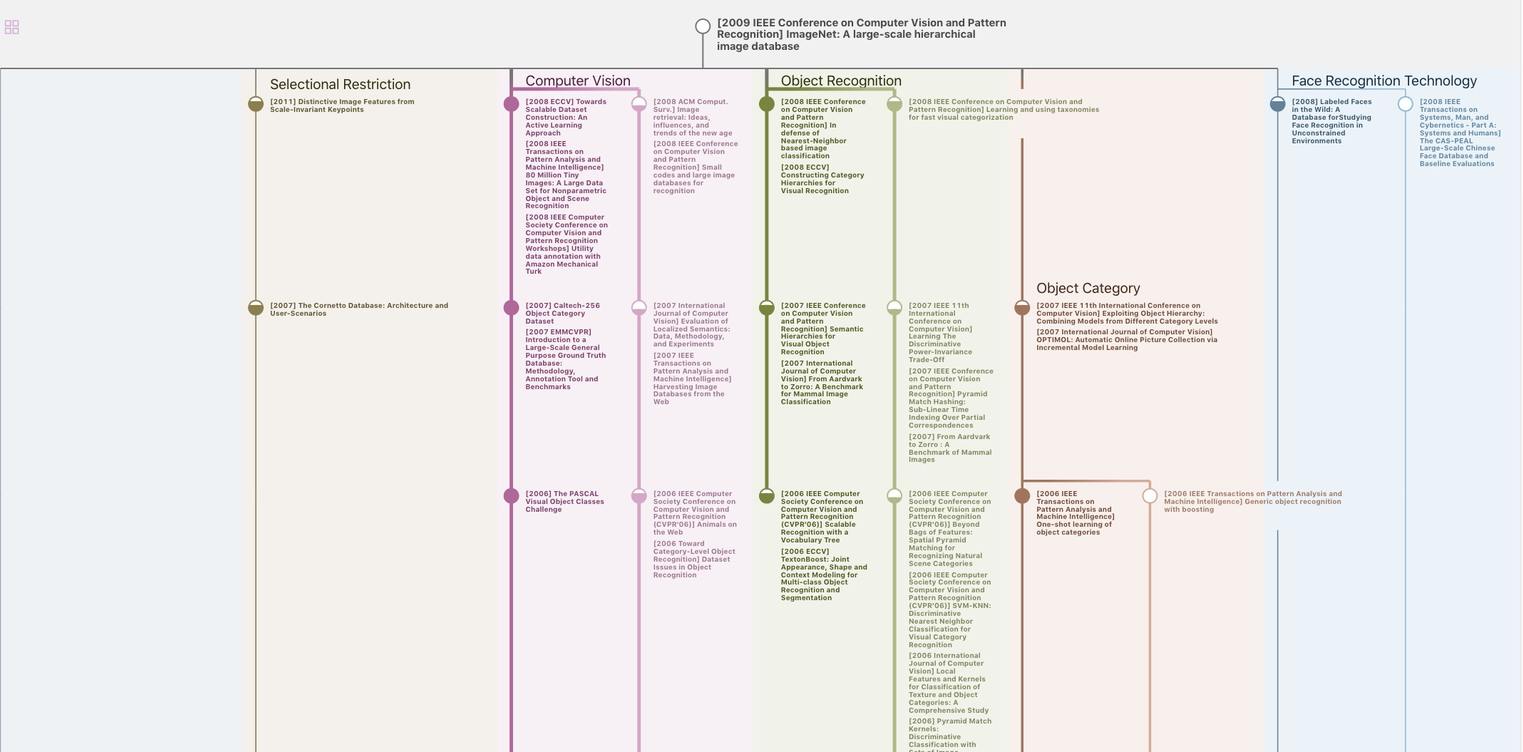
生成溯源树,研究论文发展脉络
Chat Paper
正在生成论文摘要