Deep learning feature extraction for image-based beef carcass yield estimation
Biosystems Engineering(2022)
摘要
Recently, there has been increased adoption of automation technologies in production facilities that help to curb mistakes, increase production speed and consistency, and reduce costs. Industrial automation owes its success to the advent of capable computers, smart algorithms, and data availability. In the modern-day slaughterhouse, automation technologies have been employed for operations such as cutting, deboning, grading. As one of the vital operations in the slaughterhouse, carcass grading is usually completed manually by grading staff, which is a bottleneck for production speed and consistency. However, due to the complexity of the problem, most of the technologies available for carcass grading suffer from low performance. This study aims to develop an image analysis system that uses deep-learning tools for the prediction of key beef yield parameters.The image data collected from the carcass samples were used to develop deep-learning models that extract key features, which were then used to model and predict 23 beef carcass yield parameters using multiple linear regression. The models developed achieved good prediction performance for yield parameters such as lean meat percentage (with R-2 = 0.90, RMSE = 1.98%) and other yield parameters using a few selected features. The results from this study can be used as a foundation for developing an online beef carcass grading system.(C) 2022 IAgrE. Published by Elsevier Ltd. All rights reserved.
更多查看译文
关键词
Key points,Convolutional neural networks,Yield prediction,Primal cuts,Lean meat percentage,Feature selection
AI 理解论文
溯源树
样例
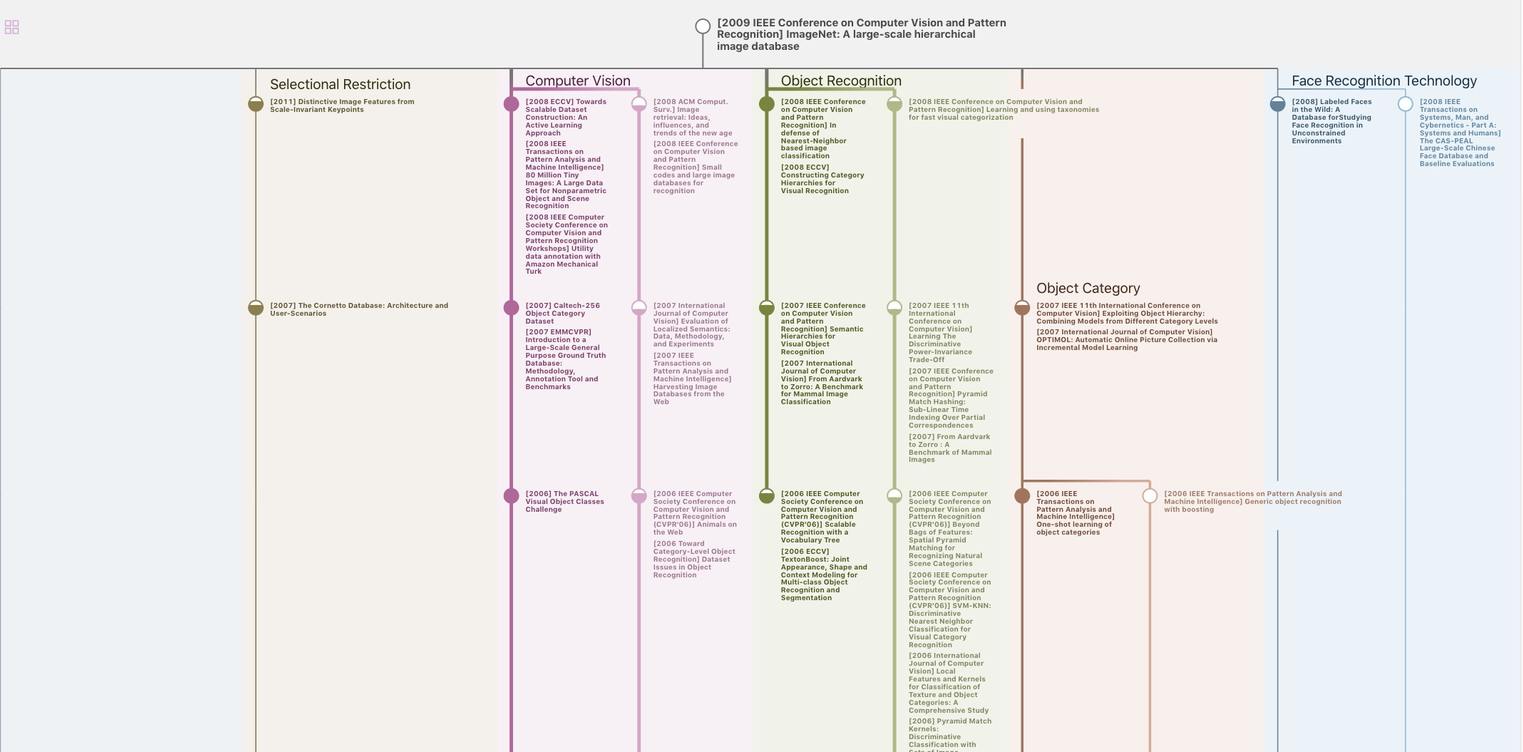
生成溯源树,研究论文发展脉络
Chat Paper
正在生成论文摘要