A sparse logistic mixture model for disease subtyping with clinical and genetic data
semanticscholar(2022)
摘要
Motivation: Identifying new genetic associations in non-Mendelian complex diseases is an increasingly difficult challenge. Yet, these diseases seem to have a significant part of heritability to explain. This missing heritability could be explained by the existence of subtypes involving different genetic factors. Taking genetic information into account in clinical trials can therefore be of interest to guide the process of subtyping a complex disease. Most methods dealing with multiple sources of information rely on data transformation, with two main tendencies regarding disease subtyping in that situation: i) the clustering of clinical data followed with posterior genetic analyzes and ii) the clustering of clinical and genetic variables. Both face limitations that we propose to leverage. Contribution: This work proposes an original method for disease subtyping from both longitudinal clinical variables and high-dimensionnal genetic markers via a sparse mixture of regressions model. The added value of our approach lies in its interpretability regarding two aspects. First, our model links both clinical and genetic data with regard to their respective initial nature (i. e. without transformation) and does not need post-processing to come back to the original information to interpret the subtypes. Also, it can adress large-scale problems thanks to a variable selection step to discard genetic variables that may not be relevant for subtyping. Results: The proposed method is validated on simulations. A dataset from a cohort of Parkinson’s disease patients was also analyzed. Several subtypes of the disease as well as genetic variants having potentially a role in this typology have been identified. Software availability: The R code for the proposed method, named DiSuGen, and a tutorial are made available at https://github.com/MCour/DiSuGen. Status: as of march 2021, this preprint has just been submitted to Pattern Recognition Letters.
更多查看译文
AI 理解论文
溯源树
样例
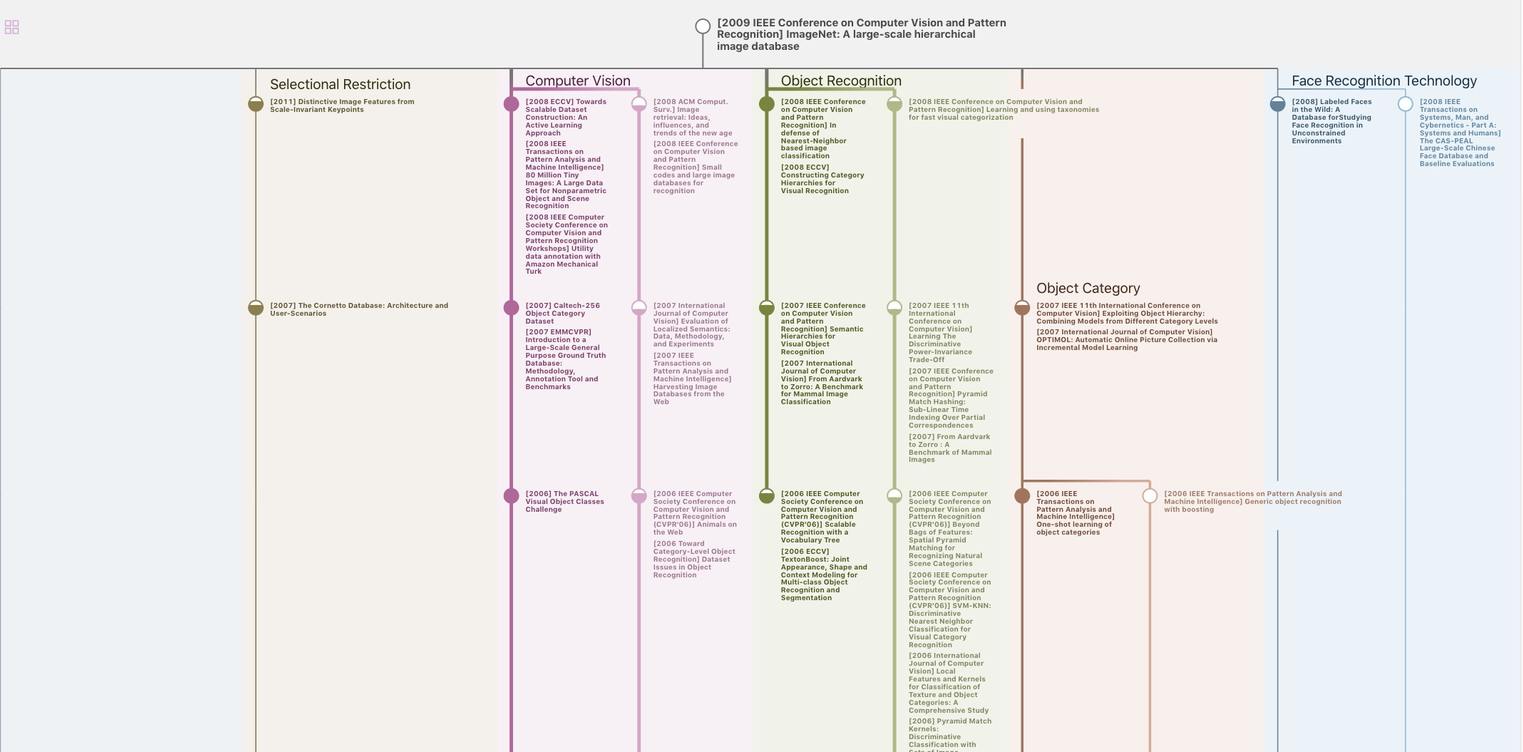
生成溯源树,研究论文发展脉络
Chat Paper
正在生成论文摘要