Spatial Transferability of Random Forest Models for Crop Type Classification Using Sentinel-1 and Sentinel-2
REMOTE SENSING(2022)
摘要
Large-scale crop type mapping often requires prediction beyond the environmental settings of the training sites. Shifts in crop phenology, field characteristics, or ecological site conditions in the previously unseen area, may reduce the classification performance of machine learning classifiers that often overfit to the training sites. This study aims to assess the spatial transferability of Random Forest models for crop type classification across Germany. The effects of different input datasets, i.e., only optical, only Synthetic Aperture Radar (SAR), and optical-SAR data combination, and the impact of spatial feature selection were systematically tested to identify the optimal approach that shows the highest accuracy in the transfer region. The spatial feature selection, a feature selection approach combined with spatial cross-validation, should remove features that carry site-specific information in the training data, which in turn can reduce the accuracy of the classification model in previously unseen areas. Seven study sites distributed over Germany were analyzed using reference data for the major 11 crops grown in the year 2018. Sentinel-1 and Sentinel-2 data from October 2017 to October 2018 were used as input. The accuracy estimation was performed using the spatially independent sample sets. The results of the optical-SAR combination outperformed those of single sensors in the training sites (maximum F1-score-0.85), and likewise in the areas not covered by training data (maximum F1-score-0.79). Random forest models based on only SAR features showed the lowest accuracy losses when transferred to unseen regions (average F1(loss)-0.04). In contrast to using the entire feature set, spatial feature selection substantially reduces the number of input features while preserving good predictive performance on unseen sites. Altogether, applying spatial feature selection to a combination of optical-SAR features or using SAR-only features is beneficial for large-scale crop type classification where training data is not evenly distributed over the complete study region.
更多查看译文
关键词
optical-SAR combination,crop type mapping,spatial cross-validation,spatial feature selection,group-wise forward feature selection
AI 理解论文
溯源树
样例
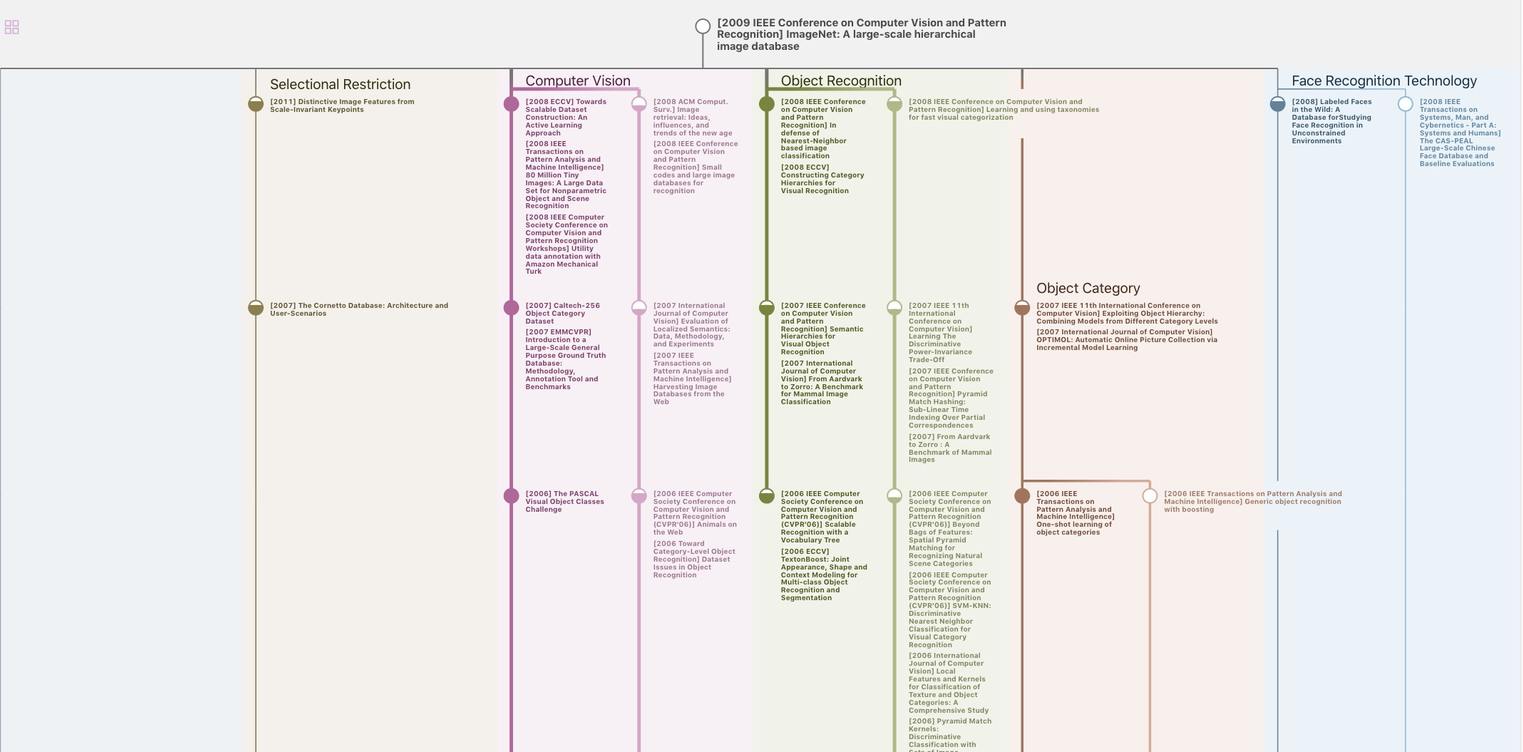
生成溯源树,研究论文发展脉络
Chat Paper
正在生成论文摘要