DLRP: Learning Deep Low-Rank Prior for Remotely Sensed Image Denoising
IEEE GEOSCIENCE AND REMOTE SENSING LETTERS(2022)
摘要
Remotely sensed images degraded by additive white Gaussian noise (AWGN) are not beneficial for the analysis of their contents. Such a phenomenon is usually modeled as an inverse problem which can be solved by model-based optimization methods or discriminative learning approaches. The former pursue their pleasing performance at the cost of a highly computational burden while the latter are impressive for their fast testing speed but are limited by their application range. To join their merits, this letter proposes a nonlocal self-similar (NSS) block-based deep image denoising scheme, namely deep low-rank prior (DLRP), which includes the following key points: First, the low-rank property of the neighboring NSS patches ordered lexicographically is utilized to model a global objective function (GOF). Second, with the aid of an alternative iteration strategy, the GOF can be easily decomposed into two independent subproblems. One is a quadratic optimization problem, and has a closed-form solution. While the other is a low-rank minimization denoising problem and is learned by deep convolutional neural network (DCNN). Then, the deep denoiser, acted as a modular part, is plugged into the model-based optimization method with adaptive noise level estimation to solve the inverse problem. In the experiments, we first discuss parameter setting and the convergence. Then, quantitative/qualitative comparisons of experimental results validate that the DLRP is a flexible and powerful denoising method to achieve competitive performance which even outperforms those produced by state-of-the-arts.
更多查看译文
关键词
Optimization,Noise reduction,Noise level,Image denoising,Convolution,AWGN,Training,Additive white Gaussian noise (AWGN),deep low-rank prior (DLRP),detail preservation,remotely sensed images (RSIs)
AI 理解论文
溯源树
样例
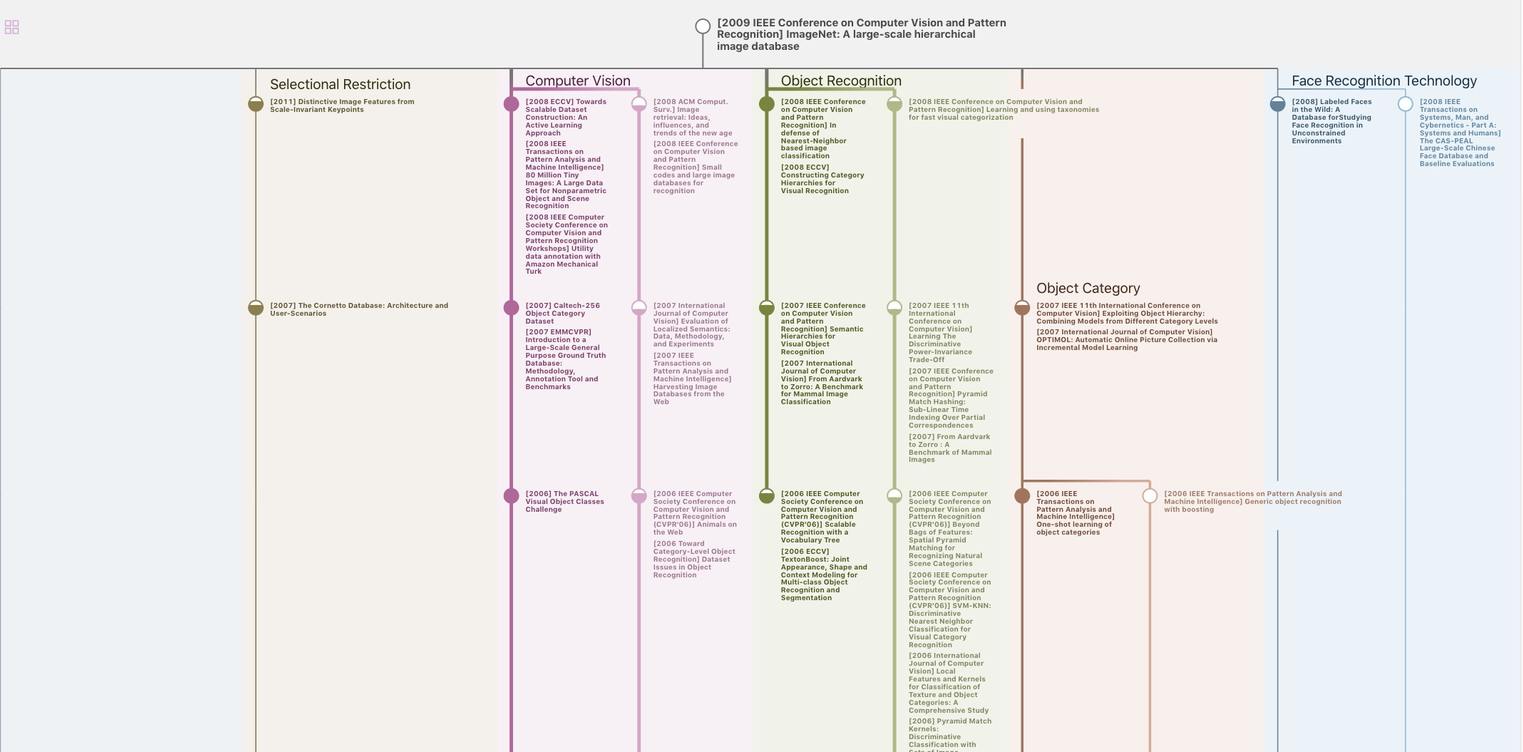
生成溯源树,研究论文发展脉络
Chat Paper
正在生成论文摘要