Scalable batch-correction approach for integrating large-scale single-cell transcriptomes
bioRxiv(2021)
摘要
Integration of the evolving large-scale single-cell transcriptomes requires scalable batch-correction approaches. Here we propose a simple batch-correction method that is scalable for integrating super large-scale single-cell transcriptomes from diverse sources. The core idea of the method is encoding batch information of each cell as a trainable parameter and added to its expression profile; subsequently, a contrastive learning approach is used to learn feature representation of the additive expression profile. We demonstrate the scalability of the proposed method by integrating 18 million cells obtained from the Human Cell Atlas. Our benchmark comparisons with current state-of-the-art single-cell integration methods demonstrated that our method could achieve comparable data alignment and cluster preservation. Our study would facilitate the integration of super large-scale single-cell transcriptomes. The source code is available at https://github.com/xilinshen/Fugue.
更多查看译文
关键词
single cell,data integration,scalability,deep learning
AI 理解论文
溯源树
样例
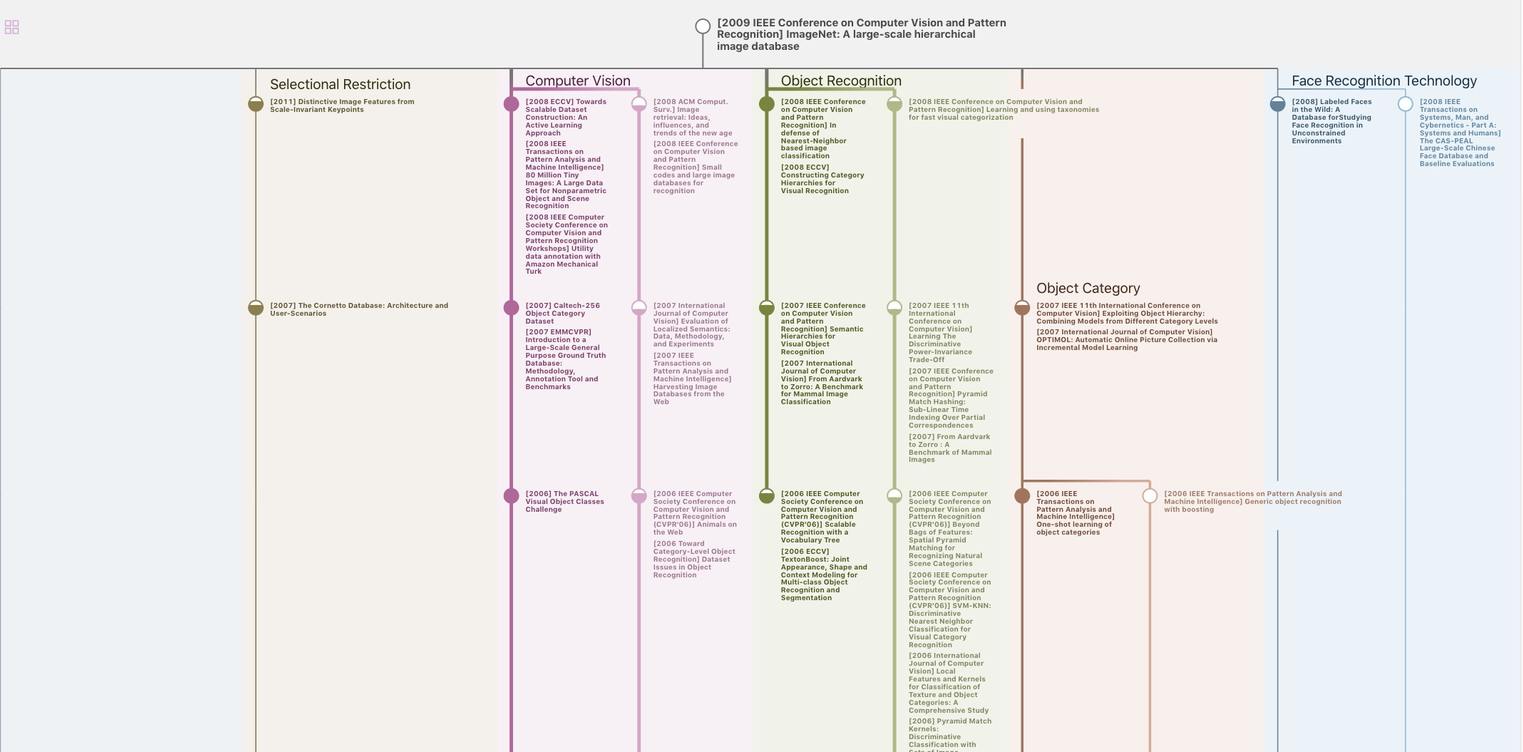
生成溯源树,研究论文发展脉络
Chat Paper
正在生成论文摘要