Deep learning method for fast prediction of film cooling performance
PHYSICS OF FLUIDS(2022)
摘要
This study examines the predictive capability of deep learning method for adiabatic film cooling effectiveness distribution with variable operating conditions and geometric layouts. A conditional generative adversarial network is trained to establish nonlinear mapping from input to output. We embed the boundary condition information directly into the input tensor, thereby imparting the capability to address variable operating conditions. The processed input tensors include different blowing ratios M, incoming turbulence intensity Tu, geometry profile controlled by the inclination angle phi, compound angle theta, number of hole rows n, pitch between hole rows P-x, and span-wise hole pitch P-y. The output data are adiabatic film cooling effectiveness fields generated by Reynolds-averaged Navier-Stokes simulations. The prediction results are in good agreement with the computational fluid dynamics results in terms of various statistical assessments. Furthermore, compared with conventional methods of solving Navier-Stokes equations, predictions based on deep learning result in better response times. Therefore, the method proposed in this study is of high significance in the early design of cooling structures for turbine blades.& nbsp;Published under an exclusive license by AIP Publishing.
更多查看译文
AI 理解论文
溯源树
样例
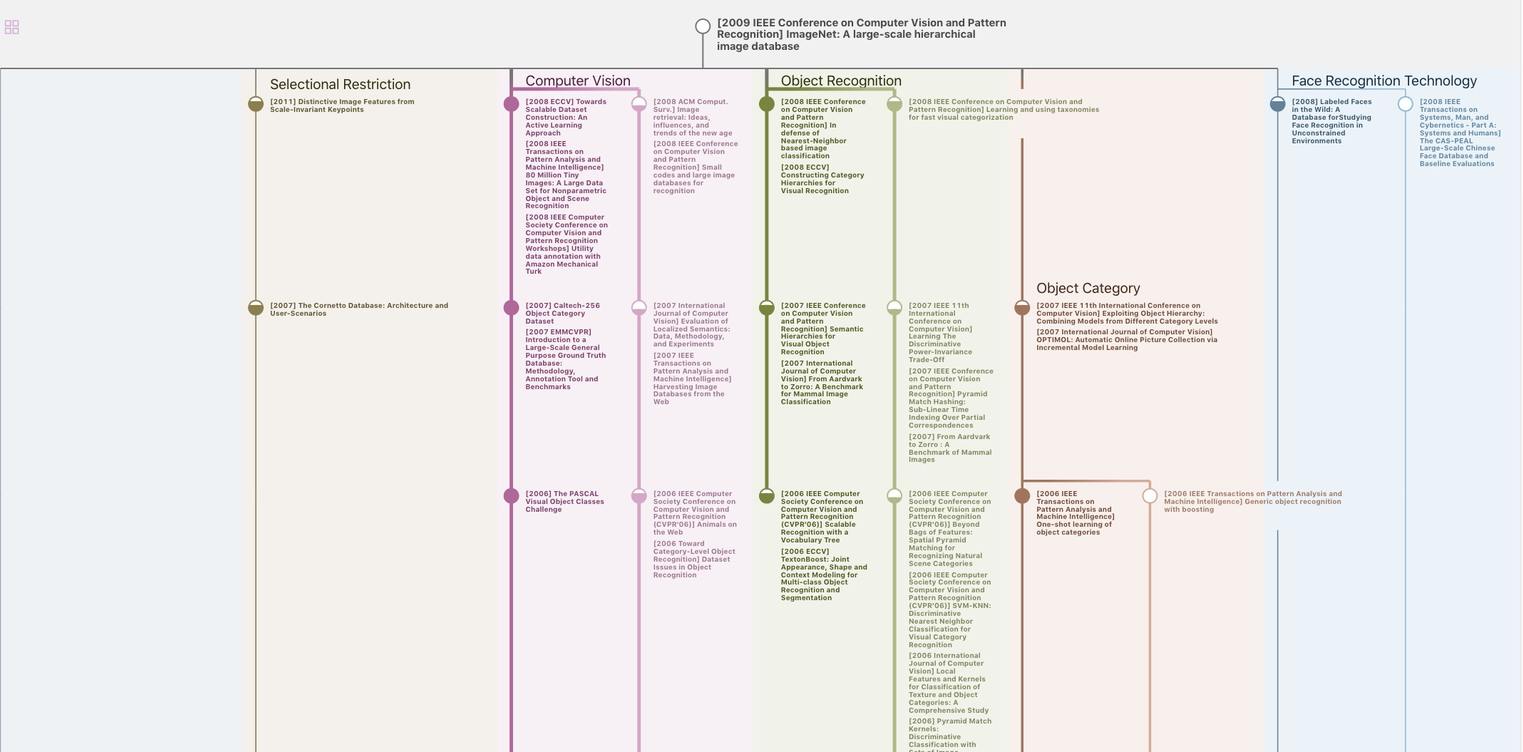
生成溯源树,研究论文发展脉络
Chat Paper
正在生成论文摘要