Difficulty-aware bi-network with spatial attention constrained graph for axillary lymph node segmentation
Science China Information Sciences(2022)
摘要
Axillary lymph node (ALN) segmentation in ultrasound images is important for the diagnosis and treatment of breast cancer. Recently, deep learning methods for automatic medical image segmentation have improved significantly. However, two problems arise. (1) A unified model is often employed to segment all images without considering the difficulty diversity. (2) The relationship between elements in the learned class probability map is disregarded. To address these two issues, we propose a novel difficulty-aware bi-network with a spatial attention constrained graph. First, a difficulty grading module (DGM) is developed to learn the difficulty grade of input images. Based on the difficulty grade of images, a novel bi-network architecture is proposed to segment the image adaptively using different branches. In complex branches, a novel spatial attention module (SAM) and graph-based energy with spatial attention constraint are proposed. The learned spatial attention map can provide additional discriminative information. Moreover, the graph-based segmentation framework can capture the relationship between pixels, further improving the segmentation performance for complex images. We conducted an experiment on our ultrasound database using 216 cases. The overall dice similarity coefficient, Jaccard coefficient, volumetric overlap error, and false positive rate are 83.41%, 74.4%, 12.02%, and 13.36% for ALN segmentation, respectively. The comparison results demonstrated that the proposed method outperforms other deep learning methods.
更多查看译文
关键词
ultrasound image,axillary lymph nodes segmentation,difficulty-aware segmentation,graph with spatial attention
AI 理解论文
溯源树
样例
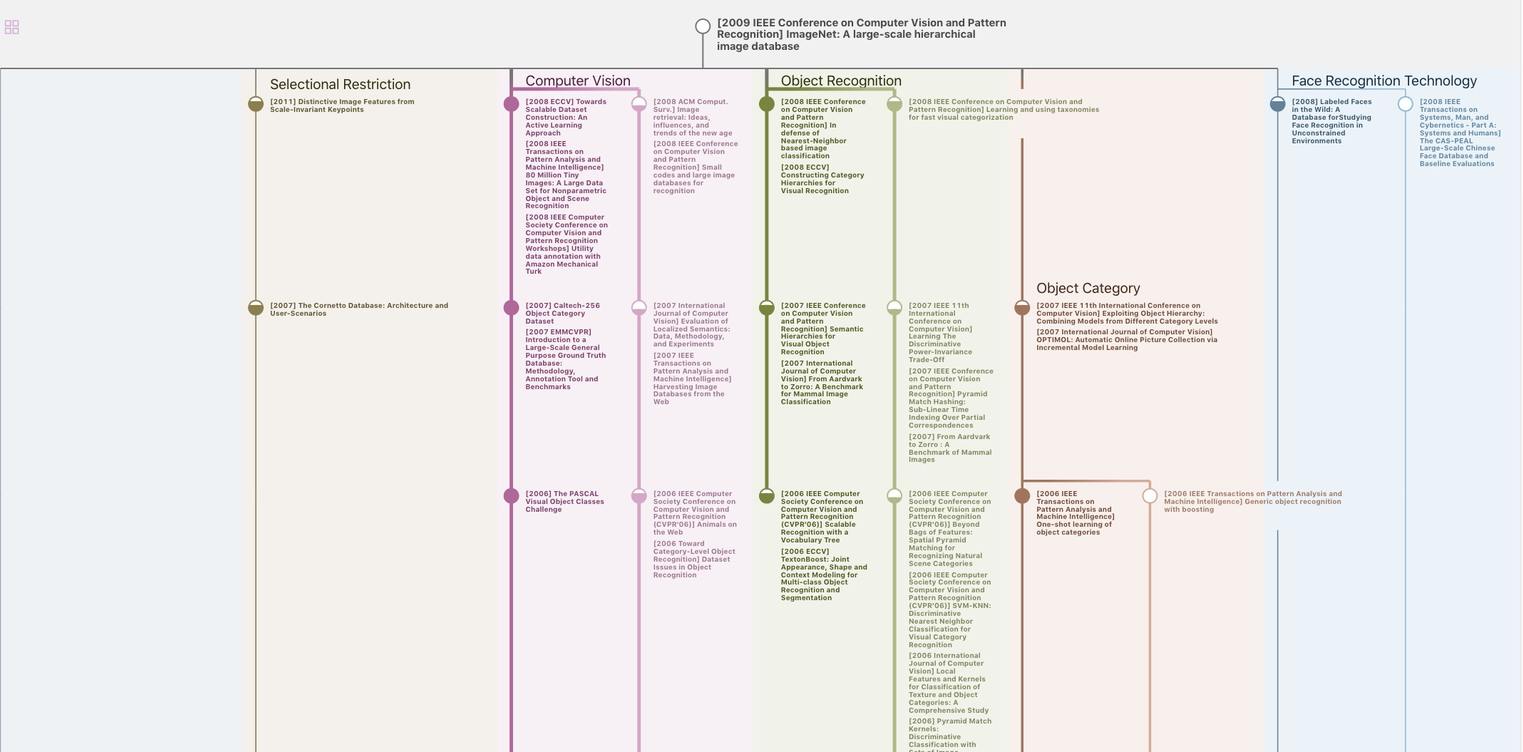
生成溯源树,研究论文发展脉络
Chat Paper
正在生成论文摘要