Deep Online Fused Video Stabilization-Supplementary Materials
semanticscholar(2021)
摘要
Network architecture. Table 1 shows the detailed network configuration of our model. Given an input video, we resize the input frames to extract forward and backward flows at the spatial resolution of 480 × 270. Our encoder consists of 5 strided convolutional layers that map the optical flow from 4×270×480 to 128×1×1. Each convolutional layer is followed by a ReLU activation function. The latent code is converted to 1× 1× 64 by an FC layer and then concatenated with the real and virtual pose histories. Therefore, our latent motion representation is a 188-channel feature vector, where 64 channels are from the encoded optical flow, 21×4 channels are from the real poses, and 10 × 4 channels are from the virtual poses. The hidden layers in the LSTM have 512 channels, and the last FC layer converts the output of LSTM to a 4D quaternion as the virtual pose of the current frame. We apply a soft-shrink activation function [6] in the last FC layer to smooth the prediction.
更多查看译文
AI 理解论文
溯源树
样例
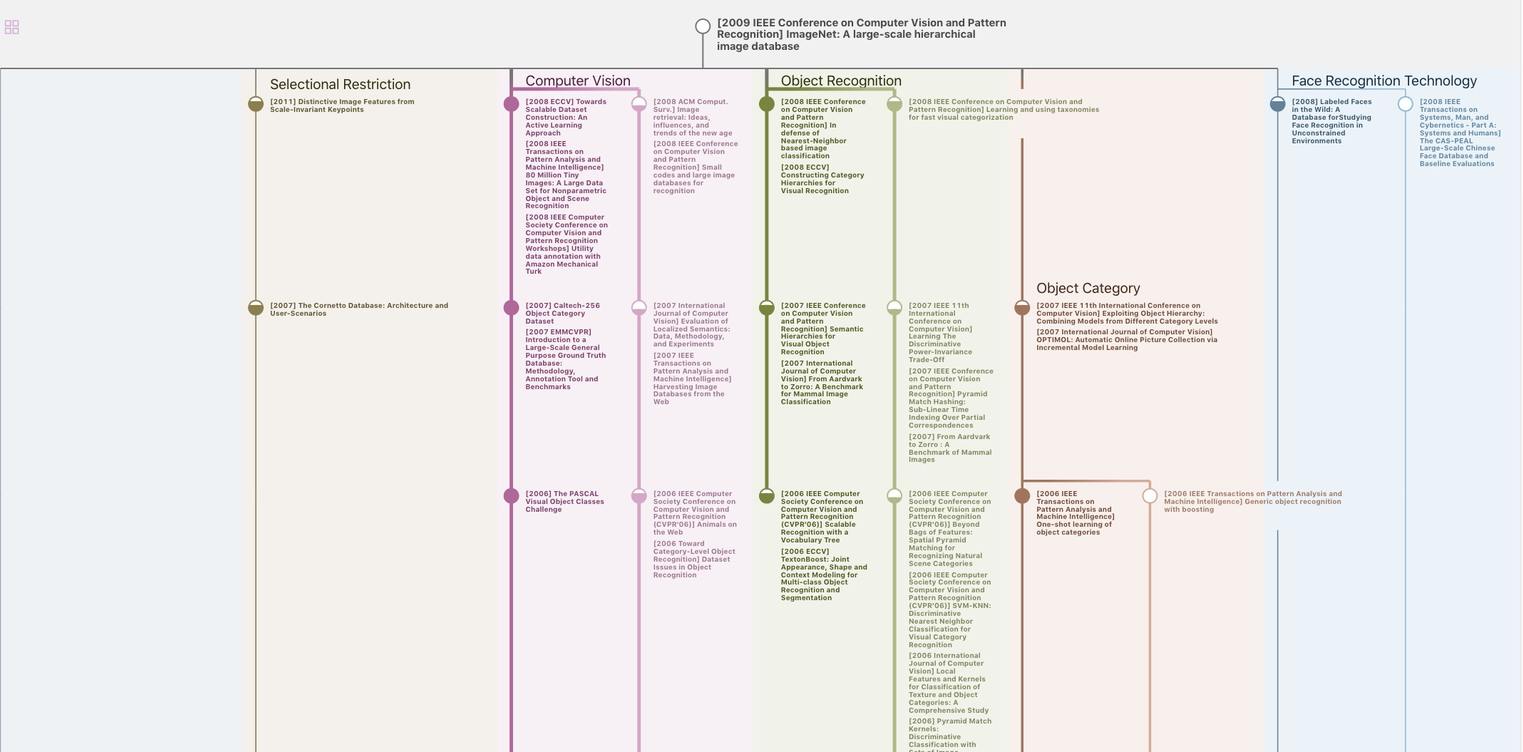
生成溯源树,研究论文发展脉络
Chat Paper
正在生成论文摘要