On the robustness of the minimim 2 interpolator
semanticscholar(2022)
摘要
We analyse the interpolator with minimal `2-norm β̂ in a general high dimensional linear regression framework where Y = Xβ∗ + ξ with X a random n × p matrix with independent N (0,Σ) rows. We prove that, with high probability, without assumption on the noise vector ξ ∈ R, the ellipsoid risk ‖β̂−β‖Σ = (β̂−β∗)T Σ(β̂−β∗) is bounded from above by (‖β‖2rcn(Σ)∨‖ξ‖)/n, where c is an absolute constant and, for any k > 1, rk(Σ) = ∑ i≥k λi(Σ) is the tail sum of the eigenvalues of Σ. These bounds show a transition in the rates. For high signal to noise ratios, the rates ‖β‖2rcn(Σ)/n broadly improve the existing ones. For low signal to noise ratio, we also provide lower bound holding with large probability. General lower bounds are proved under minor restrictions on the noise ξ (see Theorem 1). Under assumptions on the sprectrum of Σ, this lower bound is of order ‖ξ‖2/n, matching the upper bound. Consequently, in the large noise regime, we are able to precisely track the ellipsoid risk with large probability. These results give new insight when the interpolation can be harmless in high dimensions.
更多查看译文
AI 理解论文
溯源树
样例
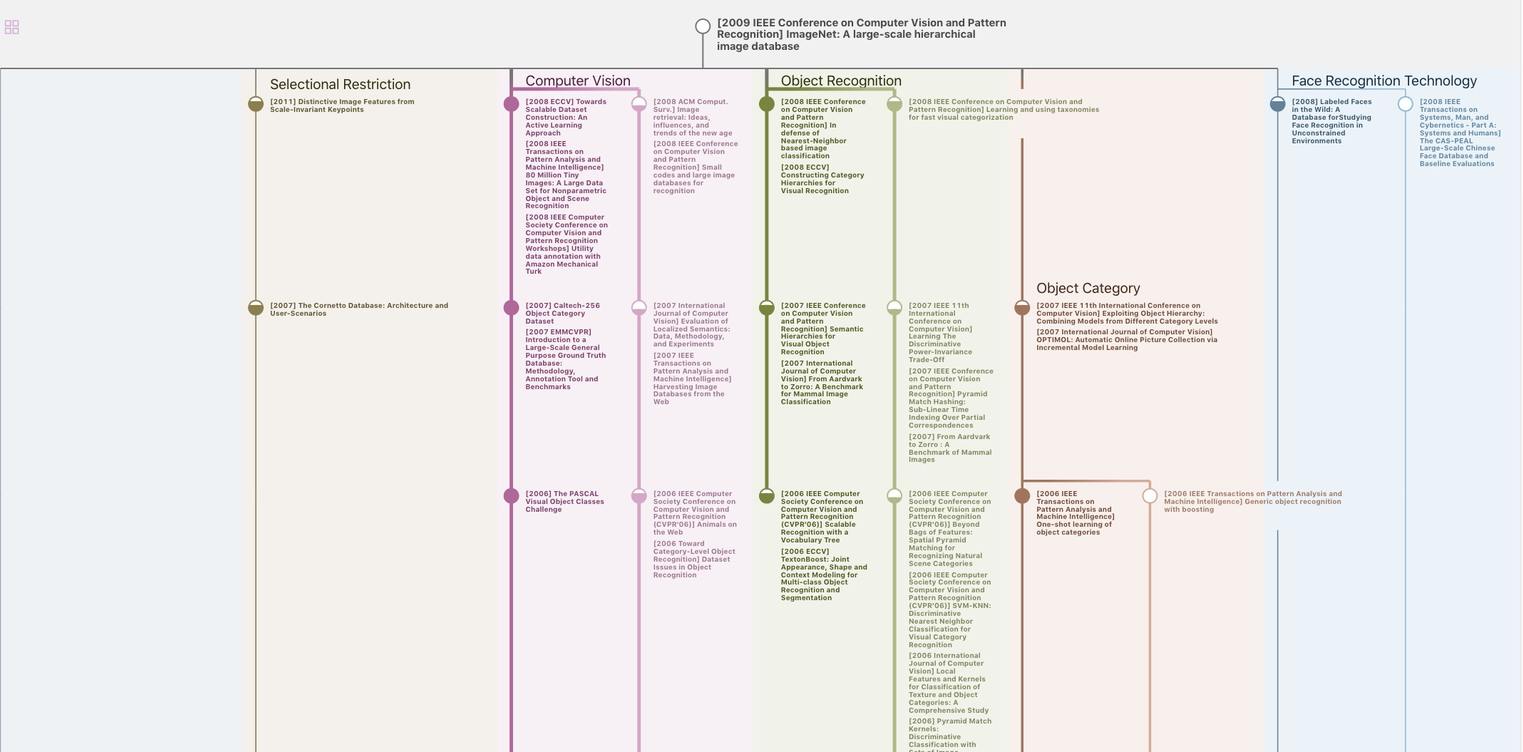
生成溯源树,研究论文发展脉络
Chat Paper
正在生成论文摘要