Lower Bounds for Differentially Private ERM: Unconstrained and Non-Euclidean
ICLR 2023(2021)
摘要
We consider the lower bounds of differentially private empirical risk minimization (DP-ERM) for convex functions in constrained/unconstrained cases with respect to the general ℓ p norm beyond the ℓ 2 norm considered by most of the previous works. We provide a simple black-box reduction approach which can generalize lower bounds in constrained case to unconstrained case. For ( ǫ, δ )-DP, we achieve Ω( √ d log(1 /δ ) ǫn ) lower bounds for both constrained and unconstrained cases and any ℓ p geometry where p ≥ 1 by introducing a novel biased mean property for fingerprinting codes, where n is the size of the data-set and d is the dimension.
更多查看译文
AI 理解论文
溯源树
样例
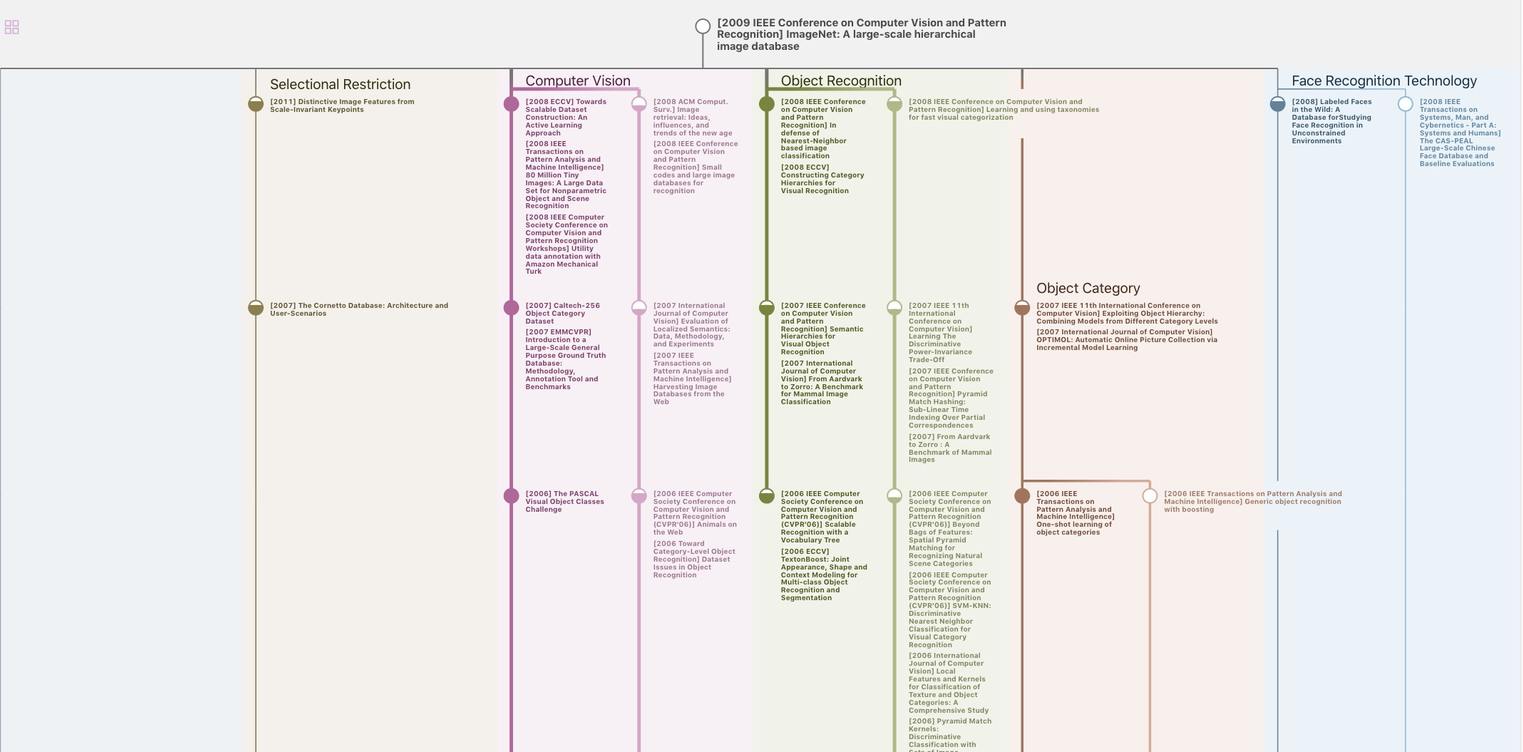
生成溯源树,研究论文发展脉络
Chat Paper
正在生成论文摘要