Fine-tuning Vision Transformers for the Prediction of State Variables in Ising Models
semanticscholar(2021)
摘要
Transformers are state-of-the-art deep learning models that are composed of stacked attention and point-wise, fully connected layers designed for handling sequential data. Transformers are not only ubiquitous throughout Natural Language Processing (NLP), but also have recently inspired a new wave of Computer Vision (CV) applications research. In this work, a Vision Transformer (ViT) is finetuned to predict the state variables of 2-dimensional Ising model simulations. Our experiments show that ViT outperforms state-of-the-art Convolutional Neural Networks (CNN) when using a small number of microstate images from the Ising model corresponding to various boundary conditions and temperatures. This work explores the possible of applications of ViT to other simulations and introduces interesting research directions on how attention maps can learn the underlying physics governing different phenomena.
更多查看译文
AI 理解论文
溯源树
样例
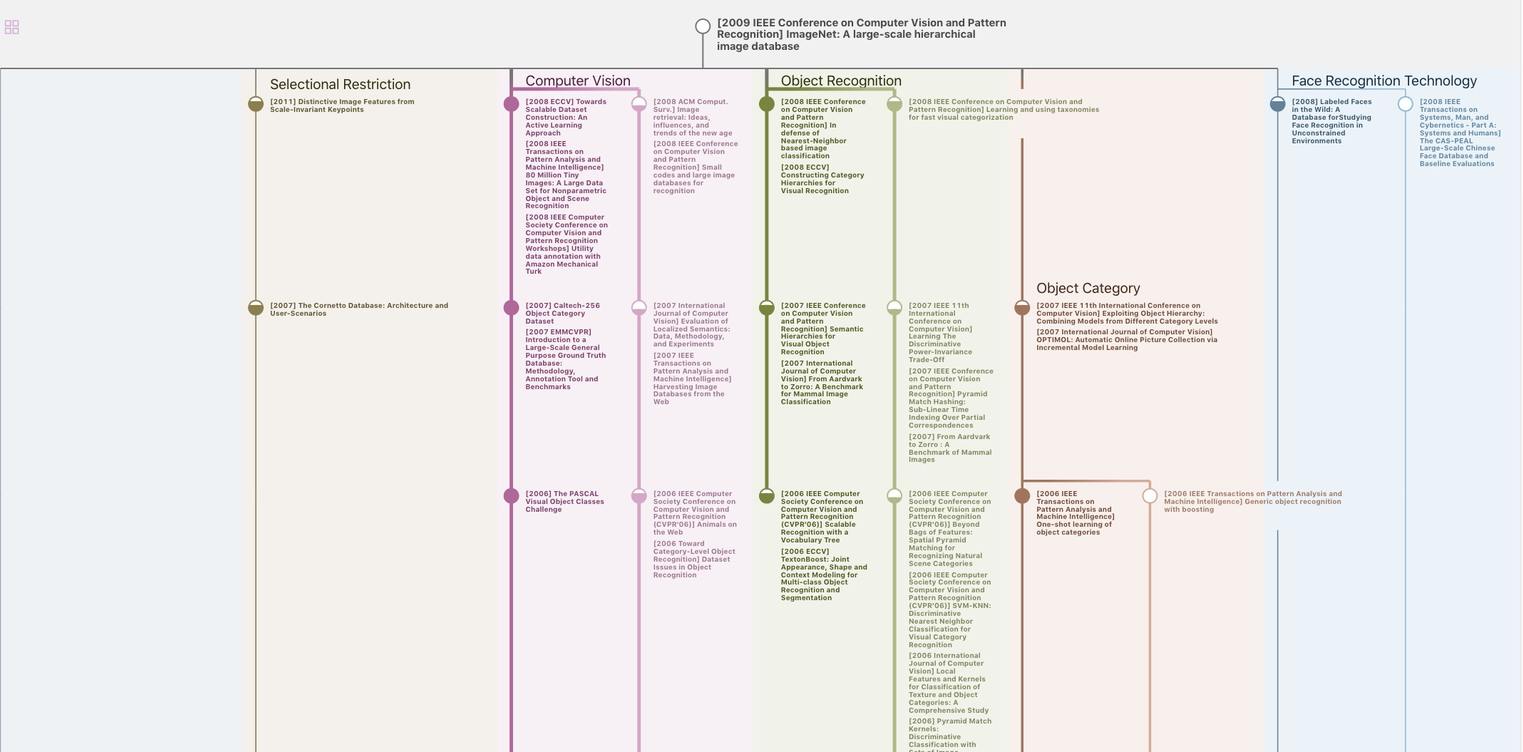
生成溯源树,研究论文发展脉络
Chat Paper
正在生成论文摘要