Short-term forecasting of Total Electron Content in South America
semanticscholar(2021)
摘要
The term space weather describes the variations in the environment between the Sun and Earth. This complex interaction is one of the principal sources of the ionosphere’s dynamics. The behavior of this part of the atmosphere represents one of the main concerns of modern society because it could threaten the proper working of modern technological infrastructure, e.g., communication networks, power grids, global navigation systems, oil pipelines. Therefore, monitoring and forecasting the Earth’s upper atmosphere behavior is a subject of increasing attention [1]. Several instruments provide information to describe the state and dynamics of the ionospheric plasma. These include ionosondes, incoherent radars, Faraday rotation, GNSS, space-borne sensors, among others. In this group, the GNSS technique is the only one that provides a 24x365 global coverage through the freely accessible global GNSS tracking infrastructure that contributes to the International GNSS Service (IGS). From these observations, it is possible to obtain the vertical total electron content (vTEC) and generate vTEC maps with a high time and space resolution. MAGGIA laboratory produces near-real-time regional vTEC maps [2] every 15 minutes incorporating data from approximately 80 GNSS satellites tracked by more than 200 ground stations. In this work, we present a vTEC forecast model for quiet and disturbed days for 2019. The input data are the regional vTEC maps produced by MAGGIA laboratory in a grid of 0.5o by 0.5o in longitude and latitude. The forecasting model consists of several steps. First, an interpolation algorithm is adapted to repair missing data. Second, an autoregressive nonlinear neural network (NAR-NN) is applied to compute vTEC multiple steps ahead in each grid point. The forecast was implemented with different horizons in advance using 1-hour sampling. Finally, interpolation techniques are studied to optimize the processing time without losing the resolution of MAGGIA maps.
更多查看译文
AI 理解论文
溯源树
样例
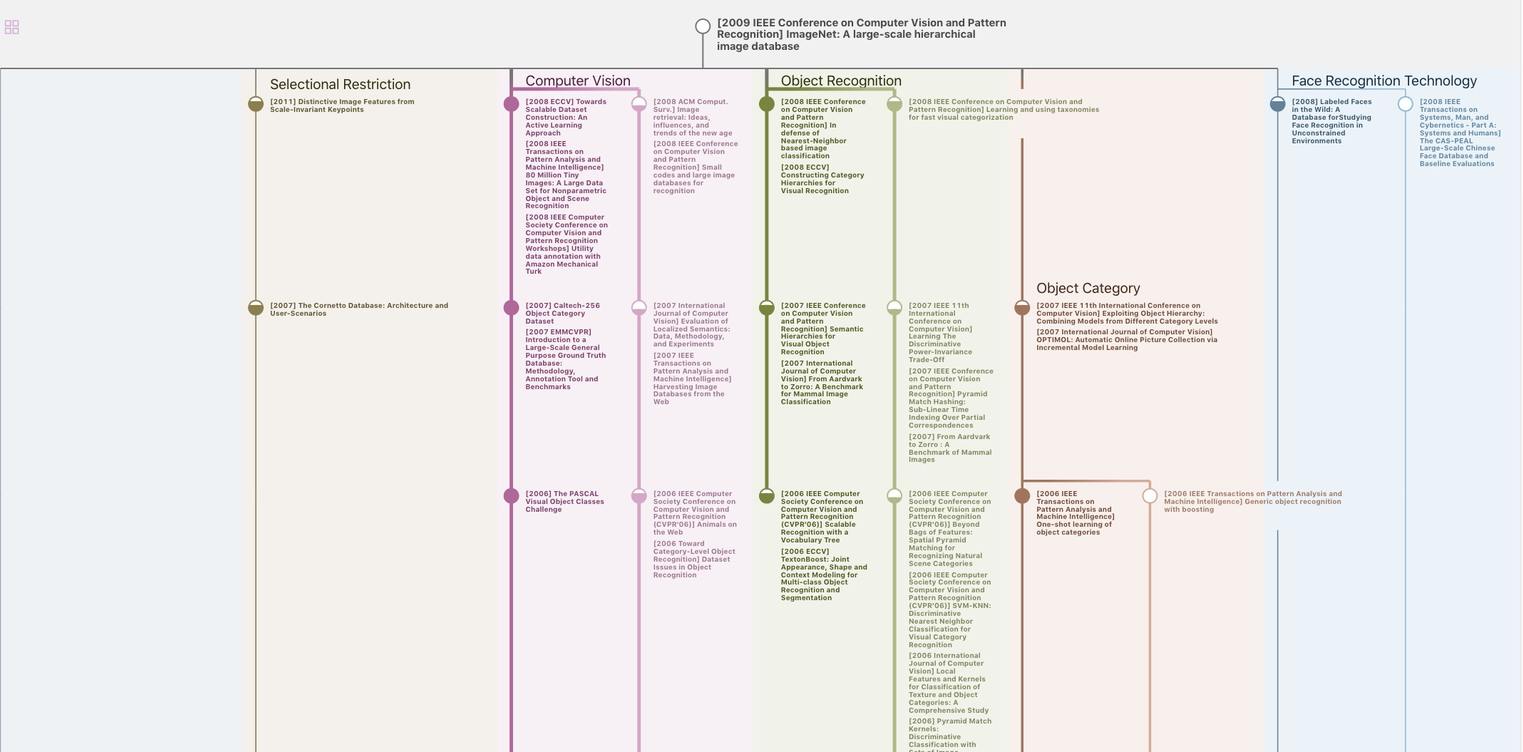
生成溯源树,研究论文发展脉络
Chat Paper
正在生成论文摘要